PRE2017 4 Groep7
0LAUK0 - Group 7
Group Members
- Bas Voermans | 0957153
- Julian Smits | 0995642
- Tijn Centen | 1006867
- Bart van Schooten | 0999971
- Jodi Grooteman | 1006743
- Emre Aydogan | 0902742
Planning
Problem Statement
A Personal assistant (PA) works closely with a person to provide administrative support, this support is usually delivered on a one-to-one basis. A PA helps a person to make the best use of their time because they limit the time spent on secretarial and administrative tasks. unfortunately having the luxury of a personal assistant is reserved for the rich and successful only, this is because of the one-to-one nature and the extensive knowledge usually required to perform PA tasks successfully. In this study the research will be focused on one aspect of a PA, which is to scan incoming messages and to only notify the person of noteworthy messages. The users in this “USE” study are defined as students in the netherlands, and because the main means of communication between students is Whatsapp Messenger. It is a good starting point to alleviate students of the current growing expected accessibility that is imposed onto them. Currently Whatsapp Messenger uses a notification system that lets you turn on and turn off notifications of a certain group or a certain person. However, in most cases this is far from ideal because if a group has a relatively low amount of relevant messages one would be inclined to switch off notifications from this group all together, but if a message sent in this group has direct relevance to the user this information would probably be missed. The goal of this study is to design some software agent that can distinguish which messages are relevant to a students academic exploits, and notifies the user accordingly. The student would effectively have a personal assistant whose role is to manage their whatsapp.
Users
Who are the users?
The users that this research is meant for the users that have to weed through countless notifications while deciding what is important to them and what is not. Hence users that deal with many of these notifications are the main goal. This research will focus mainly on the student user group, which makes it easier to define the needs and requirements of this group since this research is familiar with this group.
Requirements of the users
- The system should run on pre-owned devices
- The system should communicate with existing university infrastructure
- The system should manage the agenda of a user (e.g. notifications of upcoming deadlines, lectures and exams)
- The system should filter important information out of incoming messages
- The system should, when desired by the user, correspond on behalf of the student
- The system should tune its intrusiveness based on the users feedback
USE Aspects
This chapter takes a look at the potential impact of the product of the research. If the product fully works and solves the problem described in the problem description, it can have a great impact on the users of the product and the society as a whole. Beneath is described what impact the product can have on the users, society, possible relevant enterprises and the economy. Lastly it is described whether or not certain features are desirable.
Users
The users of the product will, as described above, primaliary be students, but it can also be extended to anybody with a smartphone who receives more messages than desired but does not want to miss out on any potentially important messages. When a person no longer has to spend time on reading all seemingly unimportant messages or scan through them looking for important messages, they will have more time to spend on things they want to spend their time on. This is a positive effect of the product as this allows the user to focus on their core business. However, the product might also have different effects on the user. Scanning texts messages or text in general for relevant information can be a valuable skill to have, as it has also applications in other scenarios, such as scanning scientific articles or reports for important information. When an AI takes care of this tasks, users might lose this skill. This might hinder them in the other scenarios as described above, where the AI possible can not help them find the important information. Another negative consequence might occur when the AI does not work perfect, but the user trusts it to work perfect. In this scenario the user might miss an important message, which can have quite some consequences. In a work environment this can mean that the user does not get informed about a (changed) deadline or meeting. In a social environment this can lead to irritation or even a quarrel.
Society
When talking about society, it means all people - users and non-users of the product - combined and everything included that comes with that. To look at what impact the product might have on the society, it is researched how relations between individuals chance, as well as how the entire society together behaves. The consequences for users as described above can be extended to a society level. If people become more productive as described above, it certainly would benefit society, as more can be accomplished. The fact that people might lose the ability to quickly scan text to find important information can also have an impact on society. If an entire generation grows up like this, there will also nobody to teach it to younger generations, meaning that society as a whole will lose this skill. Now it can be questioned how relevant such a skill might still be in future society, but its a loss nonetheless. Another thing that might occur when a large public uses the product is that nobody longer reads all the seemingly unimportant messages. If nobody reads them anymore, those who write those messages will probably stop doing so, removing the purpose of the product.
Enterprise
Possible relevant enterprises might be those who are interested to buy the product. This could be either a company like WhatsApp themselves, who want to integrate it in their application themselves, or a third party that wants to publice it as an application on its own. The companies, especially a third party, would want to make profit of such an application. companies like WhatsApp could offer it as a free service to make sure users keep using their application and possible attract new users. Third party companies can not do this and would need to find another way to make profit of the application. An easy solution for this seems to make the application not free of charge.
Economy
The product will reduce costs for users. A lot of people do not have time or do not want to filter the most important information themselfs. For this they can use a personal assistant to take over this task. But the agent will be less expensive than a personal assistant. This will save money. A disadvantage of this is that personal assistants will have less work. If people use the product of this research instead of a personal assistant for this particular task, personal assistants are not needed for this task anymore. This causes that there is less work for personal assistants.
Desirability of possible features of the agent
In this section it is researched how desirable certain possible features of the agent are. These features would probably improve the performance of the agent, but might have negative ethical consequences.
First it is analysed what the effect of the agent having access to the university infrastructure or certain other application the users uses, such as its agenda is. When the agent is able to use the information available on those platforms, such as which courses the users follows currently or when a next meeting is scheduled, the agent can make a better decision on whether or not a message is relevant on that moment of time. But is it desirable that the agent has access to these types of information? It could be seen as an infringement to the users privacy. This argument can be tackled by the fact that the user would have to give consent before the agent can access the information, as well as the fact that no human other than the user would have access to the information when the agent uses it, as it operates locally on the users smartphone. The agent could spread personal information towards third parties, if it would automatically respond to some messages. When these responses contain personal information, the privacy of the user could be lost. However, it is planned to add such features to the agent, and therefore the privacy of the user will be guaranteed.
Next it is analyzed whether or not the agent could be seen as censorship. By hiding certain messages, the agent could influence the users opinion and behaviour. If the algorithm of the agent could be manipulated by third parties, to always block or show certain messages, it could be seen as a form of censorship. This would be a bad thing and certainly not desirable. Therefore it should be impossible for third parties to influence the agents algorithm. When the application works locally on the users phone, this should be the case. Furthermore, the agent only blocks or shows the notification about a message, and not the messages themselves. When the user opens the chat application, such as WhatsApp, the user can still read all the messages it received, including those of which it did not receive a notification. Therefore, in the occasion a third party could abuse the application to censor certain messages, it would only be partial censorship. Thus it can be concluded that the application will not lead to censorship.
Approach
To start of, research to the state-of-the-art will be done to acquire the knowledge to do a good study on what the desired product should be. Next an analysis will be made concerning the User, Society and Enterprise (USE) aspects with the coupled advantages and disadvantages. At this point the description of the prototype will be worked out in detail and the prototype will start to be build. At the same time research will be done to analyse the different approaches of filtering the incoming messages and the impact they give. The results of the research will be implemented in the prototype. When the prototype is complete, the goal of the project will be reflected upon and some more improvements of the prototype can be made.
State of the art
Personal assistants
Personal assistants already exists to a certain degree in many different forms, from really simple ones that collects and summarizes important information for small-scale fishers to automatic email filtering and voice controlled physical robots. Below is highlighted some of the already existing personal assistants and explain briefly how they work.
Firstly there are the email based personal assistants. An example of this is GmailValet, a service that manages your inbox to reduce the amount of (spam) email that you receive. Another example is SwiftFile, an intelligent assistant that classifies emails and sort them in different folders. The user can easily switch between folders, viewing the different categories of emails. RADAR, yet another email filter agent, uses a different approach based on machine learning. Experiments showed this approach worked well and the agent improved really fast. This also lead to an increase in the productivity of the user. There has also been some work done of personal email assistants that can respond automatically to certain emails, such as a notification when the user is on vacation, with great success.
Personal assistants can also be used to solve other problems common in an office environment. Planning a meeting with multiple people can be really time consuming, as all participating people need to agree on the final date and time. Using a personal assistant for this problem, it could plan such meeting for 10 participants in around 5 seconds, way faster than any human could. Other personal assistants use machine learning to learn the users scheduling preferences, and makes appointments based on that.
Research about personal assistants for other tasks has also been performed, such as a module based agent that can interact with files, other programs and handle databases. There also exists a patent for a personal assistant that can answer a phone call when the user is unable to do so. Based on previous conversations it can learn how to respond and predict what the user wants. Next there is the intelligent personal assistant robot BoBi. A form of a secretary that can handle tasks normally performed by a secretary, mainly intelligent meeting recording, multilingual interpretation and the ability to read papers.
Some more know already existing personal assistants are those build into current smartphones, such as Siri and Cortana. A research paper crowns Cortana currently as the best working agent in assisting the user.
There also exists personal assistants with a focus on a more specific target audience. To make sure visually impaired people can also make optimal use of current technology, a speech based personal assistant was designed. The communication would be bi-directional, meaning that the user can talk to the agent, and the agent can respond as well. The agent could be used to open programs on a computer, perform calculation or a google search. Another variant of this is voice controlled physical robots. Commands can be given via a smartphone to the robot, which can perform various tasks in the real world.
To help small scale fisherman a personal assistant named JarPi was designed that can run on cheap technology. JarPi would be used to collect information about the current location and the weather condition, and present in in a comprehensible manner. Normally such technology is quite expansive, rendering it unavailable for small scale fisherman. A more advanced agent would be a socially-aware robot assitant, or SARA for short. By analyzing the user via various inputs such as visually, vocally and verbally, the agent will be able to create its own visual, vocal and verbal behaviours. This can be used to create a appealing robot agent, for example at an event for recommendations.
Some general research about certain element of a personal assistant has also be performed. One of the big problems of creating a personal assistant is that an user model needs to be build, in order to really personalize the agent. A solution to this could be cognitive user model which comprises an user interest model, an user behavior model, an inference component and a collaboration component. Another problem occurs when analysing text messages, as abbreviations are often used in this medium. This can be solved by creating a dictionary of the used abbreviations, so they can be converted into normal text.
Lastly, some research has been done on the impact and effects of personal assistant agents on the user and society. Research has shown that for an user to like a personal assistant it has to be “human-like” and “professional”. The agent should be able to recognize the user’s voice and answer in a natural manner. It is also important to create a physically attractive interface for the user. When other stimuli are added, it works best to use an immersive 3d visual display.
For enterprises personal assistants also bring a change. AI personal assistants are being integrated in more and more aspects of our live, and can be used for example to shop or book a vacation. A company without such service might lose out on customers to a concurrent which does have it. At the same time, when by example a travel agency starts using a personal assistant agent, they might need less employees to plan and book vacations for its customers. Also, certain functions such as a management function might see drastic chances. Currently mangeners spent a lot of their time on administrative tasks, such as making schedules for the employees and fixing holes in the planning when somebody calls in sick. When these tasks can be carried out by an AI agent, been a manager would be a different job.
Text classification and filtering
Much research has also already been done on text classification and spam filtering. Most of these researches focus on filtering spam using different algorithms. Below will be highlighted some of the already existing spam filters and text classification algorithms.
Firstly spam can be tried to be filtered using many different algorithms. An method using an artificial neural network trained with the scaled conjugate gradient backpropagation algorithm showed great success, using little classification time and high accuracy. Another researched showed that using populair binary classification algorithms such as NB, SVM, LDA and NMF, combined with a non-binary classification algorithm such as K-means or NMF also leads to great results. Yet another study showed that a recurrent neural network can also be used to filter pre-processed spam. pre-processing means maken all letters lower cases, removing all special characters and stop words, since they contain no semantic information. With an accuracy of up to 98% this method also works. Spam could also be filtered using machine-learning and calculation of word weights, although this process can become more difficult when spam starts to look more like real text. Next, instead of using a global discrimination model, a local discrimination model could be build, personalized for the user. Although it is more challenging, it would certainly be useful. Another method is filtering based on keywords, using both a whitelist and a blacklist, to calculate the probability that a message is spam. When trying to filter email spam, one could also not only look at the message itself, but also at its header and possible attachments. When a mail for instance contains an .exe file, mainly used in spam email, it could automatically flag it as spam.
At the same time as researchers try to develop better spam detecting, spammers try to find new ways to elude spam filters. This way it keeps getting harder to make a fully functional spam detection algorithm.
On the field of text categorization and classification, much research has also already been done. First up naive Bayes could be used in different variants to classify text messages. When adding preprocessing or incorporating additional features the efficiency did not increase nor decrease drastically. However, it does reduce the feature space of the classification algorithm, which is beneficial when working with limited resources. discriminative or generative recurrent neural networks can also be used for text classification. Both of them have their different uses, and are better depending on the scenario. The generative model is especially effective for so called zero-shot learning, which is about applying knowledge from different tasks to tametisks that the model did not see before. The discriminative model is however more effective on larger datasets. These kinds of text classifications can also be used to find recommendations for users, to to filter messages on their relevance. A learning personal agent can be used to find new relevant information. The agent both learns from the user what he deems relevant, en classifies text to find whether or not it is indeed relevant to said user. A different approach to text classification is a keywords-based approach. Filtering on text messages on relevant keywords, the amount of notification that needs to be send to the user can greatly be used, sending only notifications of those message that are marked urgent or important. This method is also quite effective. Lastly, to easy the text classification algorithms, preprocessing can be done. By removing words that are seemingly irrelevant to determine its classification, the classification is both faster and reduce the feature space. Different techniques can be used to remove the irrelevant words, all with their pros and cons.
State of the art sources
Sources - Personal assistant/email filtering
Understanding adoption of intelligent personal assistants: A parasocial relationship perspective[1]
The article is about intelligent personal assistants (IPA’s). IPA’s help for example with sending text messages, setting alarms, planning schedules, and ordering food. In the article is a review of existing literature on intelligent home assistants given. The writers say that they don’t know a study that analyzes factors affecting intentions to use IPA’s. They only know a few studies that have investigated user satisfaction with IPA’s. Furthermore is the parasocial relationship (PSR) theory presented. This theory says that a person responds to a character “similarly to how they feel, think and behave in real-life encounters” even though the character appears only on TV, according to the article. Lastly is there a lot about the study in the article. The hypotheses of this study are: H1. Task attraction perceived by a user of an IPA will have a positive influence on his or her PSR with the IPA. H2. Task attraction perceived by a user of an IPA will have a positive influence on his or her satisfaction with the IPA. H3. Social attraction perceived by a user of an IPA will have a positive influence on his or her PSR with the IPA. H4. Physical attraction perceived by a user of an IPA will have a positive influence on his or her PSR with the IPA. H5. Security/privacy risk perceived by a user of an IPA will have a negative influence on his or her PSR with the IPA. H6. A person’s PSR with an IPA will have a positive influence on his or her satisfaction with the IPA. H7. A person’s satisfaction with an IPA will have a positive influence on his or her continuance intention toward the IPA.
Personal assistant for your emails streamlines your life[2]
This article is about GmailValet, which is a personal assistant for emails. Normally is a personal assistant for turning an overflowing inbox into a to-do list only a luxury of the corporate elite. But the developers of GmailValet wanted to make this also affordable for less then $2 a day.
Everyone's Assistant[3]
This article is about “Everyone’s Assistant”, which is a California based service company for personal assistant services in Los Angeles and surrounding areas. The company makes personal assistant service affordable and accessible for everyone. The personal assistants cost $25 a hour and can be booked the same day or for future services.
Experience With a Learning Personal Assistant[4]
This article is about the potential of machine learning when it comes to personal software assistants. So the automatic creating and maintaining of customized knowledge. A particular learning assistant is a calancer manager what is calles Calendar APprentice (CAP). This assistant learns by experience what the user scheduling preferences are.
SwiftFile: An Intelligent Assistant for Organizing E-Mail[5]
This article is about SwiftFile, which is an intelligent assistant for organizing e-mail. It helps by classifying email by predicting the three folders that are most likely to be correct. It also provides shortcut buttons which makes selecting between folders faster.
An intelligent personal assistant robot: BoBi secretary[6]
This article is about an intelligent robot with the name BoBi secretary. Closed it is a box with the size of a smart phone, but it can be transformed to a movable robot. The robot can entertain but can also do all the work a secretary does. The three main functions are: intelligent meeting recording, multilingual interpretation and reading papers.
RADAR: A Personal Assistant that Learns to Reduce Email Overload[7]
This article discusses artificial learning agents that manage an email system. The problem described in the article is that overload of email causes stress and discomfort. A big question remains that it is not sure whether or not the user will accept an agent managing their email system. Nevertheless the agent improved really fast and improved the productivity of the user.
Intelligent Personal Assistant — Implementation[8]
This article does research to the best and most promising current Agents used by the major companies such as apple and microsoft. The conclusion of this paper states that cortana is currently the best working agent in assisting the user.
Intelligent Personal Assistant[9]
This article is about the current by speech driven agents that perform tasks for the user. In the paper this communication would become bi-directional and therefore will the agent respond back to the user. It will also store user preferences to have a better learning capacity
Voice mail system with personal assistant provisioning[10]
A patent that describes a PA that can be used to keep track of address books and to make predictions on what the user wants to do. The patent also suggests text-to-speech so that the user can listen to, rather than read the response. The PA should also remember previous commands and respond accordingly on related follow-up commands.
USER MODEL OF A PERSONAL ASSISTANT IN COLLABORATIVE DESIGN ENVIRONMENTS[11]
The article is about creating models of the users of PA’s and the different domains associated to the user and the PA. The article suggests four different user models, user interest model, user behavior model, inference component and collaboration component. According to the article the user should have the right to change the user model, since ‘the user model can be more accurate with the aid of the user.’ Two approaches are through periodically promoted dialogs or by giving the user the final word.
A Personal Email Assistant[12]
The paper is about Personal Email Assistants (PEA) that have the ability of processing emails with the help of machine-learning. The assistant can be used in multiple different email systems. Some key features of the PEA described in the paper are: smart vacation responder, junk mail filter and prioritization. The team members of the paper found the PEA good enough to be used in daily life.
Rapid development of virtual personal assistant applications[13]
This patent is about creating a platform for development of a virtual personal assistant (VPA). The patent works by having three ‘layers’, first the user interface that interacts with the user. Next is the VPA engine that analyses the user intent and also generates outputs. The last layer is the domain layer that contains domain specific components like grammar or language.
A Softbot-Based Interface to the Internet[14]
The article describes an early version of a PA that is able to interact with files, search databases and interact with other programs. The interface for the Softbot is build on four ideas: Goal oriented, Charitable, Balanced and Integrated. Furthermore, different modules could be created to communicate with the softbot in different ways, like speech or writing.
Socially-Aware Animated Intelligent Personal Assistant Agent[15]
The article describes a Socially-Aware Robot Assistant (SARA) that is able to analyse the user in other ways than normal input, for example the visual, vocal and verbal behaviours. By analysing these behaviours SARA is able to have its own visual, vocal and verbal behaviours. The goal of SARA is to create a personalized PA that, in case of the article, can make recommendations to the visitors of an event.
JarPi: A low-cost raspberry pi based personal assistant for small-scale fishermen[16]
This article describes how fisherman can also have a form of a personal assistant, that keeps track of the weather and current position on the sea. Normally such systems are really expensive and not available for small-scale fisherman, but using cheap technology such as the raspberry pi a great alternative can be created.
Solution to abbreviated words in text messaging for personal assistant application[17]
This article describes how a personal assistant that reads incoming text messages such as SMS-messages can handle abbreviations, which are commonly used in text based messaging. The study was performed with abbreviations common in the Indonesian language, based on a survey.
A voice-controlled personal assistant robot[18]
This article described the design and testing of a voice controlled physical personal assistant robot. commands can be given via a smartphone to the robot, which can perform various tasks.
Management Information Systems in Knowledge Economy[19]
AI Personal Assistants: How will they change our lives�[20]
How artificial intelligence will redefine management[21]
How can AI transform public administration?[22]
Sources - Spam Filters/Machine Learning
Intellert: a novel approach for content-priority based message filtering[23]
This article described how filtering text based on its content and keywords leads to great reduction in the amount of notification that has to be send, by only sending those messages that are marked urgent or important. The results look promising.
Content-based SMS spam filtering based on the Scaled Conjugate Gradient backpropagation algorithm[24]
Classification of english phrases and SMS text messages using Bayes and Support Vector Machine classifiers[25]
Generative and Discriminative Text Classification with Recurrent Neural Networks[26]
This article analyses the difference between discriminative and generative Recurrent Neural Networks (RNN) for text classification. The authors find that the generative model is more effective most of the time, while it does have a higher error rate. The generative model is especially effective for zero-shot learning, which is about applying knowledge from different tasks to tasks that the model did not see before. The discriminative model is more effective on larger datasets. The datasets that are tested range from two to fourteen classifications.
SMS spam filtering and thread identification using bi-level text classification and clustering techniques[27]
The problem that this article is addressing is the large amount of sms messages that are sent and that identifying spam or threads in these messages is difficult. First the spam is classified, which could be done with one of four popular text classifiers, NB, SVM, LDA and NMF. These are all binary classification algorithms that either work with hyper planes, matrices or probabilities to split up the classes. Next, the clustering is applied to construct the sms threads, which is done by either the K-means algorithm or NMF. The results of the article are that the choice of the algorithms is very important. The algorithms used in the experiment are SVM classification and NMF clustering which give good results.
Spam filtering using integrated distribution-based balancing approach and regularized deep neural networks[28]
This article is about creating a spam filter with the help of a Recurrent Neural Network. The spam filter is intended for both SMS and email. The network is tested on four spam datasets, Enron, SpamAssassin, SMS and Social Networking. The experiment starts by pre-processing the datasets such that there are only lower cases, no special characters and no stop words, since these contain no semantic information. The results of the experiment are compared with the following spam filters, Minimum description length, Factorial design analysis using SVM and NB, Incremental Learning, Random Forest, Voting and CNN. The results of the experiment are that the model is better on three of the four datasets by a small amount and the accuracy is around 98% for the three and 92% for the last one.
A Comparative Study on Feature Selection in Text Categorization[29]
This article researches five different techniques to categorize text.
A Learning Personal Agent for Text Filtering and Notification[30]
This article is about an agent that is used for managing notifications. This agent acts as a personal assistant. This agent learns the model of the user preferences in order to notify a user when relevant information becomes available.
Combining Collaborative Filtering with Personal Agents for Better Recommendations[31]
This article is about information filtering agents that identify which item a user finds worthwhile. This paper shows that Collaborative filtering can be used to combine personal Information filtering agents to produce better recommendations.
Spam filtering using integrated distribution-based balancing approach and regularized deep neural networks. [32]
This article is about anti-spam filters by using machine-learning and calculation of word weights. This categorizes spam and non-spam messages. This categorizing is more and more difficult because spammers use more legitimate words.
Robust personalizable spam filtering via local and global discrimination modeling[33]
There are two options of filtering: a single global filter for all users or a personalized filter for each user. In this article a personalized filter is presented and the challenges of it. They also present a strategy to personalize a global filter.
Mail server probability spam filter[34]
This article is about a spam filter that uses a white list, black list, probability filter and keyword filter. The probability filter uses a general mail corpus and a general spam corpus to calculate the probability that the email is a spam.
The Art and Science of how spam filters work[35]
This article explains the principle of blacklists which analysis the header of a message to determine whether something is spam. Also messages that contain statistically dangerous files, such as .exe files, are often automatically blocked by content filters. The article end with a piece about Machine Learning in spam filters. Algorithms used in these filters try to find similar characteristics found in spam.
The Effects of Different Bayesian Poison Methods on the Quality of the Bayesian Spam Filter ‘SpamBayes’[36]
This article discusses how spammers try to elude spam filters. The principle works as follows: add a few words that are more likely to appear in non-spam messages in order to trick spam filters in believing the message is legitimate. This article illustrates that even spam evolves, and as a result filters have to evolve with them.
A review of machine learning approaches to Spam filtering[37]
This paper presents a review of currently existing approaches to spam filtering and how the researchers believe we could improve certain methods.
Prototype description
To get a good understanding of what kind of prototype is required for the described problem and the given user, a concrete goal needs to be described that will fulfill a good selection of the user requirements described in the section above. After a concrete goal is described a prototype design needs to be created to solve the problem described in the problem statement.
Goal
The goal that the prototype should fulfill is dependent on the user requirements that have been described. Since it is not possible to create a prototype that is able to achieve all requirements in the current planning, a selection of important requirements will be chosen that are to be implemented in the prototype. The rest of the requirements are going to be analysed and researched in a written manner to still be able to give insights in their importance to the user.
The requirements that are chosen for the prototype are the following:
- The system should run on pre-owned devices
- The system should filter important information out of incoming messages
- The system should tune its intrusiveness based on the users feedback
So the prototype will become a software module that can be implemented by existing messaging applications like Whatsapp, telegram or other messaging applications that can be used to send and receive messages between a large group of people. The module will output a binary value depending on whether the message is important or unimportant. To determine the grandiosity the system should base its reasoning on feedback that the user gives during setup or usage of the application.
Design
To achieve the goal described above, two prototype design variations will be created to be able to analyse their effectiveness. The first variation will be using keyword based filtering which has the advantage of having an understandable filtering process, since the keywords support the reasoning. The second variation will be using machine learning in the form of a recurrent neural network (RNN), which is often used for text based machine learning. These two subsystems will be integrated in a larger system that also involves the removal of clearly identifiable spam and the coupling of closely related messages in the form of threads.
Input Output interface
The required input for the filtering module should be as abstract as possible to support as many different messaging applications as possible. However, there should be consistency in the input format. Not only the message itself is important, but also the metadata like the date and time, the sender, whether a message is a response to a different message and whether any media like images is coupled with the message. The prototype will not be able to analyse any coupled media but the information of media being present can still be useful for filtering. Messages are inputted in batches, just like they are for unread notifications. The messages in a batch should all come from the same group chat since the messages could be coupled with each other. The module will then process this batch without taking other batches into account. The output of the filtering module will be a boolean value indicating for every individual message, whether the message should be shown to the user or should be discarded.
Spam filter
The first step to start analyzing the messages is to filter the spam out of the messages. The purpose of this is to cut out the messages that do not really have an influence on the context. For example the smiley’s are mostly not important. Therefore when there is a message with only smiley’s the program can categorize this as spam and thus filter it out. In this part the message is clearly looked at from a point that it only looks at what the actual text of a message is. To give an example, a message with a strange combination of letters would be filtered out. Thus the program does not pay attention to the meaning of a message but to the actual content of that particular message. Filtering out the spam before analyzing is important because the program would not have to analyze messages that have no influence in the first place.
Categorization
The messages will also be categorized in groups that are concerned with the structural meaning of the sentence. These are for example questions, answers or announcements. By extracting this information from the messages the program can give the user even more options to filter the incoming messages. A certain user might only be interested in announcements and not in questions. This can be indicated and the appropriate messages can be shown or discarded without going through the next layers of the program. When a user does not give a preference for a certain category the messages will be propagated to the next layer, which is the thread layer.
After the clearly identifiable spam messages have been discarded and the categories have been detected, the remaining messages can be coupled together in so called threads. This is done to retain important information that could be spread over multiple messages. A factor that could indicate a thread is for example the time of sending the messages, since messages sent in a short timespan will most likely involve the same subject. Another factor is the person that sends the messages, since information is most of the time coming from one person and is intended for all the others. The last factor is when a message is a reply on a different message. This is a feature that some messaging applications support and will link the messages that is being replied on to the new message. These two linked messages most likely need to be coupled together.
These coupled messages are then combined in such a way that the filtering in the next step will take the combined messages into account before determining the importance of the message.
Filtering
Now the program starts with categorizing the coupled messages in two groups. The first group is the important messages and the second are the unimportant messages. There are multiple ways of doing this, but the prototype will only involve two of them. Namely Keyword based filtering and Recurrent neural networks.
Keyword based filtering
The first method is keyword based filtering. This method makes use of a predefined list of important keywords. Every message is checked and given a score on how many important keywords are in that message. When a message has a higher score than a certain threshold the message will be placed in the group important messages.
Evaluation of messages will be done in a few steps. First the program checks if the message has one of the following words: Who, what, when, where. By checking these words the program already gains a lot of information about the message. The next step is to analyze what kind of word is stated after one of the W words. For example when there is a sentence that ends with who. It might not be as important as a sentence that starts with who. This is because the sentence that ends with who is not a question and thus might not have a much meaning as the other one. Also the message that ends with Who is not grammatically correct. This indicates that it has a low priority. In addition to that the length of the message is taken into account. The longer the message the more important it is most of the time.
Recurrent neural networks
The second method is recurrent neural networks. This method uses learning to categorize messages. Therefore it needs training. There are two ways of obtaining this training. The first one is to analyze messages by hand and use this to train the neural network. The second one is to give a set of messages to the user of the product and let the user categorize these messages. This creates personalized test data for all the users and thus will the neural network also be a personalized to a user when using this test data to learn. Combining these two methods of creating training data is the best thing to do. This is because then the neural network can have more training and it is not fully personalized. The fact that it is not fully personalized is a good thing because the user would otherwise fully rely on his categorization. When the user would not be able to categorize the messages the program would perform bad. Now with using both training data sources the program is optimized. Using a neural network gives a certain percentage of correct categorized messages. There option is there to make the user give a percentage to the program and that it keeps learning until this percentage is reached.
Recurrent neural networks have a simple structure with a built in feedback loop, which allows it to act as a forecasting motor. They are extremely versatile in their applications. In feedforward neural networks signals flow in only one direction from input to output, one layer at a time. In a recurrent net, the output of a layer is added to the next input and fed back into the same layer, which is typically the only layer in the network. A recurrent net can receive sequence as input, and can also send out a sequence as output, this ability increases the versatility of recurrent neural networks as opposed to feed forward loops. Typically an RNN is an extremely difficult net to train. Since the network uses backpropagation, one runs into the problem of the vanishing gradient. The vanishing gradient is exponentially worse for an RNN, the reason for this is that each time step is the equivalent of an entire layer in a feed forward network. i.e. training a RNN for 100 time steps is like training a one hundred layer feed forward net. This leads to exponentially small gradients and a decay of information through time. There are several ways to address this problem ,the most popular of this is gating. Gating is a technique with which the network decides the current input and when to remember it for future time steps.
Both options of filtering the messages can be used separately or combined. An analysis will be performed when both filters are finished and based on that analysis the evaluation function will be created, which is explained in the next section.
Evaluation function
The evaluation subsystem will evaluate the incoming messages with the results of the different filtering options. Based on the results of the filtering options a different evaluation function can be chosen. Some ideas for the evaluation function are only choosing a result of one of the filters; taking the average; taking the maximum or minimum or looking at the magnitude of the difference. The evaluation subsystem also allows for personalization, since the users can indicate a degree of how many messages need to be filtered out, which can be transformed into a threshold that can be compared to the result of the evaluation function. Furthermore, personalization can be applied in the form of asking feedback. Users will most likely not want to give feedback on every message that is filtered so the results of the two filtering options could be used to get an understanding of the certainty of the network in filtering that message. If, for example, the difference of two filtering options exceeds a value that can be indirectly set by the user, the program can show the message and ask whether it is useful.
Preprocessing
The input the program gets is most of the time a really raw input. When analyzing emails the input will be a perfectly fine piece of text without typo’s and strange non-important messages in between.On the contrary the program that is being build has to take into account that in whatsapp a lot of typo’s are made and a lot of different strange text messages will be sent that do not mean anything by first seeing them. When an user is more used to the Whatsapp languages he or she gets to know some abbreviations that do not exist in the normal speaking languages. Therefore preprocessing is necessary to make it the program a lot easier to “read” and interpret all the messages.
The idea that removes all the words like: “the”, “is”, “was”, “where” might be a good idea to implement. Generally those words are not important to the meaning of a message. Those words are called stopwords. This would be implementing with have a list of stopwords, the stoplist. Then all the messages would be scanned for those stopwords and then the stopwords would be removed from the message. The idea to remove the stopwords would be a benefit to the program because it would have less clutter and non important words to analyze. Nevertheless it would make it harder to identify questions, as it removes one of the most important parts of the message that would identifies it as a question. Therefore this preprocessing step would suit the program more when it is done after the messages are categorized, and thus be a processing step somewhere in the middle of the programm.
An addition to the preprocessing, that other research papers suggested, could be that all the verbs would be translated back to their root form. This is called stemming. When having a sentence with the word talking in it. It would be replace talking with talk. In addition to that all the different verbs of talk would also be replaced with talk. When doing this the set of words that have to be checked would be a lot smaller because the list would only need to have one word instead of five different verbs of that word.
Threads
To get more out of singular messages threads can be used to to couple multiple messages into threads. With this the program can analyze a conversation instead of a single message. In conversations the topic does not change much. Therefore in single messages there might be a topic that is not literally stated in that message. Looking the context, the other messages, most of the time there is a topic that is addressed in that message. This is why threads are a great tool to analyze messages.
When will messages be coupled together in a thread is the next question. The most important property that the program will take into account is time. When messages fall in the same time interval they will be coupled. This is a very basic but good implementation. The second property that will be implemented is checking who is the sender of a message. When the same person sends more messages right after each other. The chances are pretty high that those messages address the same topic. Thus the messages should be put into a thread.
The paper suggest the usage of K-Means or NMF to cluster the messages. K means works well when the shape of the clusters are hyper-spherical. For the algorithm constructed in this research the clusters are not hyper-spherical. This is not the case in the implementation of clustering messages. Also for both K-means and NMF the number of clusters have to be predefined. In the case of clustering messages the number of clusters is not defined before clustering. A third algorithm to cluster is hierarchical clustering. This algorithm starts with giving every instance its own cluster. Then the algorithm starts combining clusters until it converges. This works well for this implementation because in hierarchical clustering there is not a predefined amount of clusters. However with hierarchical clustering a depth limit has to be specified. This could be a disadvantage for this implementation.
Clustering based on time is fairly easy because every timestamp is a number and the program can cluster on the distance between messages using the euclidean distance function or another distance function. In which the distance is the difference in time for the mean of two clusters. Clustering based on who sent the message is harder. There is not a way to do clustering on contacts using an euclidean or other similar distance measures, thus therefore a different method needed to be implemented to work with categories instead of numbers, which is described below.
Distance function for clustered categories
To compute a reasonable distance measure for categories that are uniformly distributed, meaning the individual differences are equal, inspiration from the Levenshtein distance was taken. The reason why the Levenshtein distance itself is not completely what is desired for the distance of categories, like the sender is because it also takes the order and the length into account. If the order or length is different between two clusters containing the same two senders the distance could be greater than the distance between two equal length clusters having different senders.
The next step was to make a sketch of multiple clusters with a different length and sender configuration. Some general rules were established to get an idea of which cluster pairs should receive a higher distance than others. For example two equal clusters should have a distance of 0 and two completely different clusters should have a distance of 1. Then the cluster ABB was compared with clusters AB, AC and C with the desired order of increasing distance: AB, AC, C. To establish the distance, fractions are made with the denominator equal to the sum of the two clusters together and the numerator equal to the sum of different senders in both clusters. As can be seen in the table below, this gives the desired result. To balance this distance function out with the other possible distance functions a multiplication factor has been added that can scale the distance depending on the importance of the clustering categories.
clusterDistance(c1, c2): diffNumber = #{c1 - c2} + #{c2 - c1} totalNumber = #{c1} + #{c2} return diffNumber / totalNumber
A | B | C | AB | AC | ABB | |
---|---|---|---|---|---|---|
A | 0 | 1 | 1 | 1/3 | 1/3 | 2/4 |
B | x | 0 | 1 | 1/3 | 1 | 1/4 |
C | x | x | 0 | 1 | 1/3 | 0/4 |
AB | x | x | x | 0 | 2/4 | 0/5 |
AC | x | x | x | x | 0 | 3/5 |
ABB | x | x | x | x | x | 0 |
Classic Naive Bayes
In order to design a new technique to classify relevance of messages, it is necessary to first look at established techniques that approximate the goal of a Whatsapp spam filter. The first technique that comes to mind is the use of Bayes classifiers. Naïve Bayes classifiers are a popular technique In use for e-mail filtering. Typically spam is filtered using a bag of words technique, where words are used as tokens to calculate the probability according to Bayes Theorem that an e-mail is spam or not spam(ham).
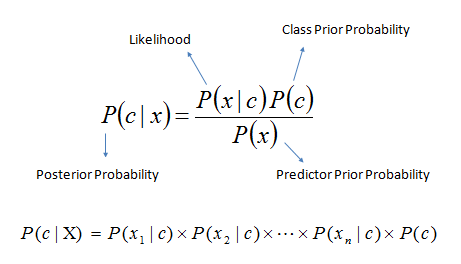
To demonstrate how a naïve bayes spam filter might work, consider the example of a database of a random number X spam messages and 2X ham messages. It is now our task to classify new e-mails as they arrive, based on the currently existing objects. Since the amount of ham messages is twice the size of the spam messages, a new (still unobserved) message is twice as likely to be a member of ham than to be a member of spam. In Bayesian theorem, this probability is known as prior probability. These probabilities are solely based on previous observations. With the priors formulated, the program is ready to classify a new message. The message is broken up into words and each word is ran through the conditional probability table of all words in the database. Through this process the likelihood of the message being spam or ham is calculated. Finally, the posterior probability of the messages belonging to either class is calculated and whichever is higher is the class the message will be assigned to.
Words have a certain probability of occurring in either spam or ham. The filter does not know these probabilities in advance, it needs to be trained first so they can be built up. For instance, the spam probability of words like “Sex” or “Nigerian” are generally higher than the probabilities of names of family members and friends. When the agent is trained, the likelihood functions are used to compute the chance of an e-mail with a particular set of words belongs to either the spam or the ham class. One of the biggest advantages of Bayesian spam filtering is the fact that it is possible to train the filtering to each user, creating a personal spam filter. This training is possible because the spam a user receives correlates with that users activities. Eventually a Bayesian spam filter will assign a higher probability based on the user’s patterns. This property makes the use of a Bayesian classifier particularly attractive for Whatsapp spam filtering as the types of messages a user receives vary widely for users of the app. Bayesian Classifiers might also assign accurate probabilities from messages received from different groups as the group name can be used as a token as well.
Research questions
Feedback before using the prototype
The easiest and probably most obvious way to get personal data from the user is to give them a form to fill in. This form would consist of some general questions like: “Are you a student?”, “If so, where are you studying?”, “Do you consider positive enforcing messages important? (think of a confirmation or a compliment)” and so on. Giving the user such a form to fill in has the advantages that the program would already be able to be personalized when it will do its job in the beginning. Also during the improvement of the programm while the user is using it, it would need less feedback from the user because it already got a lot. The disadvantages of using such a form is that when an user answers such a question, the program makes an assumption based on the answer. For example when the user says that is has football as hobby, the program takes a certain list of words with all the keywords for football in it and gives the user a notification when one of those keywords is sent. But it might be the case that the user is not interested what happens in the champions league at all, but it plays football as a hobby. Another disadvantage of using a from might be that the user does not like to take the time to fill it in. Furthermore when the user fills in that he or she likes everything that is in the form the program will not do anything. Considering this the choice has been made to not include a form in the beginning.
Contact biasses
For making the program more personal, considering the contacts of the user is a very important aspect in doing this. This can be done in a couple of different ways.
The first option on how to consider contacts is to label a contact with a tag which would for example be: “Peer”, “Teacher” or “Brother”. In reality there would be a lot of different tags. Also when the contact is not in one of the categories of the tags the user would be able to create a new tag and give that tag a importance rate. The user would give this tag to a sender when he receives a messages from him or her. This tagging would only be done once and that would be the first time when the user receives a message from the sender. The advantage of this is that the program can use the information from the tag to get a better view of the importance of a message. The biggest disadvantage is that the user would have to do a lot of tagging the the beginning.
The other option would be to consider if the user has the contact in his or her phone. And according to this give a priority to the contact. There are three priorities for a contact. These priorities are low, medium and high. When a the number of the contact is already in the phone of the user. The contact is set to a medium priority. When the contact’s number is not in the phone of th user the contact is set to low. The user is able to manually adjust the priority of a contact in the user interface. This solution would be good because the user does not have to do a lot of work in the beginning. In addition to that the program would be able to consider the contact in determining the importance of a message. Also the user would be able to customize the priorities when desired.
By taking both options into consideration the choice has been made to implement the second option. This is because the the advantages of being able to customize when desired and not having to do a lot of work are decisive.
Feedback while using prototype
Getting feedback from the user is always important to consider. An user is more satisfied most of the time when something or someone cares about their opinion. From an use aspect making a feature that takes the opinion of the user into account would be a great thing to do. Then the question arises in what way would it be best to do this.
The easiest thing to do would be to give the user the option to give their opinion whether or not a message is useful after every message. This would be an easier option to implement but a rather annoying one for the user. The program would get a lot of information to learn and would be able to filter better probably. On the contrary the user would have to give a lot of feedback. Imagine geting 100 messages in an hour in a groupschat. Then the user would get the question 100 times whether or not the program did good. This is a huge disadvantage and does outweigh the advantages of this solution.
The next solution to this problem would require significantly less feedback from the user. For classification of the messages the program uses different algorithms. When the different algorithems do not give a matching answer. Then there will be asked for feedback from the user. In this way the program can learn from the things that are unclear to the program and the user would not have to give a lot of feedback. Also because the program would improve itself, it would ask for less feedback over time. This solution should in theory work much better than the first one. Therefore the choice has been made to implement this solution. It will fit the use aspect really well as the user will be giving feedback and wont be annoyed by the amount of feedback it has to give.
Communicate with the university infrastructure
In the the designed prototype, the only action the agent can do which impacts the user is to show or hide messages. however, it could prove advantageous to have the agent operate in more ways than just that. if for example, the user would receive a lot of messages about an upcoming group meeting and the agent has access to the users timetable, the agent could easily filter these messages into one category. The other way around could be that if a few group members schedule an appointment and invite the user over whatsapp, the agent could introduce an event in the users calendar. A very useful tool for people who are more forgetful of actually scheduling planned meetings. These new ways to act could also have a downside because they introduce complexity into the agent as for example, each course a user takes would have different keywords that are relevant and the dataset should then contain keywords for each course, this could show the agent down. This challenge will not be tackled in this study, but research into it could be useful for future studies.
Respond on the users behalf
An important message does not necessarily require a very complex action, if these actions could be handed over to a robotic PA the user need not spent as much time replying with simple “yes” and “no” answers. In the event of for example a question being asked to the user the PA would recognize this as such and respond appropriately. Simply said, the user is saved the time of having to respond to these messages. Furthermore, in the event where the PA is synchronized with the agenda of the user, automatic ‘do not disturb’ or ‘unavailable right now’ messages could be dispersed whenever the user is prompted to reply to a message. Of course, being able to disable these features is part of the system of the PA.
Having described these features there is something to be said for its disadvantages. A PA letting someone know that you are unavailable might lead to sharing information you never wanted to share. Perhaps an extreme example of this is someone with malicious intentions ‘pinging’ your PA to know whether you’re in a meeting or not, which could potentially signal that you’re not home.
Another flaw of a PA is that it isn’t personal enough. The PA responding to messages might make the sender of the message feel like he or she is talking to a robot instead of having a personal conversation with the person the message was intended for. Finally, and this might be more on the user than the PA itself, your agenda is not always fully up to date. The PA might think that you’re in a meeting right now and respond with a do-not-disturb, while in reality that meeting was cancelled yesterday and you just forgot to remove the meeting from your agenda.
Since the overall feeling was that the disadvantages outweigh the advantages, the decision was made not to include this kind of functionality in the PA.
User Survey
Since the team themselves are potential users of the product, they already have a general idea about what the user wants. However, the team only consits of 6 people, and opinions may vary greatly. To get a better idea of what other users would want from the product, a survey was created. The survey has been sent to other students of TU/e, therefore, almost all responses are from other students. This can have influence on the results, but since the product is also targeted towards these students, the responses still seem to be representative for the potential users.42 people filled in the survey. The survey itself can be found here: Survey Regarding WhatsApp Spam Filtering The goal of the first question was to see how big of a problem the problem the team tries to solve actually is. The amount of people who are not very annoyed by WhatsApp notifications is quite large. 20 out of the 42 people (47,6%) answered with a 4 or lower. This is the same amount of people that answered with a 6 or higher. Overall it can concluded that the problem, although less than initially thought, is indeed present to a certain degree among students of the TU/e. The full result can be seen below:
Next up was aksed how interested people were in the presented solution to the problem. Most people (21 out of the 42 people, 50%) said that they would maybe use the application. 11 people (26,2%) answered yes and 10 people (23,8%) answered no. If half of the people who answered maybe and all of the people who answered yes would end up using the product, half of the respondents would use the product. Therefore it can be concluded that there exists a market for the product.
In question 3 and 4 it was what the respondents thought about the presented idea to receive feedback from the user, which is required to personalize the application. Many people (22 people, 52,4%) thought the idea where the user can give explicit feedback to the application by marking whether or not a message was indeed important was a good idea. 11 people (26,2%) answered maybe and 9 people (21,4%) answered no. Surprisingly, way less people answered yes on the follow-up question whether or not they would actually use the feedback function. Here only 13 people (31%) answered yes, while the amount of people who said they would probably not use the feature grew to 17 (40,5%). The remaining 12 people (28,6%) answered maybe. So although many people liked the idea, it is questionable whether or not it will generate enough feedback to fully personalize the application, as is desirable.
To end the survey, an open question was presented to the respondents, where they could write feedback, tips or other general remarks regarding the problem. Many people mentioned that WhatsApp already have functions to manage notifications. Although this is indeed true, it does not fully solve this problem, since when you mute a chat, you will receive no notifications of the chat at all, even when an important message is send in that chat. Others mentioned that other programs have options were the sender of the message can mark a message as important. This solution however imposes 2 problems: first of it lays the work of marking a message as important not by the receiver, but by the sender. Secondly, what one finds important differs from person to person. When a sender marks a message as important, the receiver might not find it important at all, or he might want to see a message that was not marked as important by the sender.
Some responders also came with other useful feedback. First of it was suggested to not only let the users give feedback whether or not a message marked as important was indeed important, but also whether or not a message marked as unimportant was indeed unimportant. This way the application can also learn when it misses something important. It was also suggested to use a scale to give feedback instead of a yes or no question, to get a better understanding of how important a message was. Next up was the suggestion to use implicit feedback instead of explicit. This can be done by checking for example how long it took the user to read the message or if the user ignored the notification, and whether or not the user responded to the message.
Many people also mentioned privacy in their response. They were concerned about WhatsApp (or the application) filtering messages for them, in a form of censorship. They also mentioned they did not want other people or WhatsApp to know what they found important. This is a legit concern and it should be carefully noted how the application uses certain information, and who can have access to it.
Prototype progress
The following section will show the progress of the prototype over multiple iterations. Each iteration is approximately one week and will contain the actions done in bullet points as well as a written summary of the implementation with occasional images.
Iteration 1
- Created structure with class diagram
- Implemented the base structure from the class diagram in java
- Started on question sentence detection for categorization
- Started on thread layer with a hierarchical clustering algorithm
- Started on UI
This iteration is the start of the creation of the prototype so the first action done was to create a good structure that is flexible enough to change the order of filters and other layers in the prototype later on. For this, a class diagram is made that is inspired from the prototype structure made for the prototype design. The class diagram shows that an abstract layer class is the parent of all of the layers in the prototype, which enables the use of restructuring the layers on the go when necessary. A layer class is very basic and only has a child layer and some methods for processing the messages and propagating them through to the child layer. The filter layer extends from the layer class and has an extra ‘alternative layer’ that is used to feed the messages to that got filtered out. Again there is an abstract method that should handle the filtering and which can be implemented by the subclasses which are the spam filter and the categorization filter for now. Next up there is a thread layer that is able to make use of different clustering algorithms for coupling related messages. For now only the hierarchical clustering algorithm is implemented with the properties time and sender but different algorithms could be implemented to see which works best. The evaluation layer is used to create an abstract structure that can be used to utilize multiple different evaluation methods for determining the degree of importance. The keyword evaluation is the evaluation that is going to be implemented next. After all evaluation methods have processed the messages an evaluation function needs to merge the results in a single value and determine whether the message is important or not. The last layer is the output layer which catches all messages that are outputted at different layers like the spam filter or the evaluation layer and returns the collected messages in order with the addition of an importance result.
The complete structure that can be seen in the image is already implemented in the programming language Java. This language is chosen since Java is also used for the Android operating system which is very open and could allow the prototype to be inserted and read from the incoming notifications. Furthermore Java is well known by the team. The prototype is already able to process messages created by hand, since dummy implementations have been made for all the layers. This allows implementation of some layers while the other layers might not work as intended yet. The layers that do have some implementation are the categorization filter and the thread layer.
Categorization filter
For the categorization filter, the detection of questions has been started on as the first category. The way that the question categorization works for now is to have a list of words that often indicate a question sentence when these words are placed at the beginning of the sentence. Different words receive a different amount of points, since some words always indicate a question sentence and other words occasionally. Furthermore a message can have multiple sentences from which only one is a question. To be able to detect this each individual sentence is processed and if there is a word indicating a question at the start, it will be detected. This works better than only looking at the first word of the message, since for some questions a small sentence might be before it to introduce the question. An example can be seen in the results table for the message sent at time 6. The last feature that is detected is a question mark at the end of a sentence, which also gives some points to the sentence showing a higher resemblance to a question. After the detection is done the points are compared with a threshold and if the number of points is greater than the threshold, the sentence is classified as a question. In next iterations the classification will be improved to work with other forms of question sentences and other categories will also be added. Below are the results of fifteen sentences of which are five questions. Four out of five questions are correctly classified as a question.
Time | Sender | Message | Question categorization | Expected answer |
---|---|---|---|---|
0 | John | test | No | No |
1 | John | spam | No | No |
2 | Jane | this is spam | No | No |
3 | Jan | real message | No | No |
4 | Henk | real good message | No | No |
5 | John | Is this good? | Yes | Yes |
6 | Henk | hello! shall we go to the beach? | Yes | Yes |
10 | John | Are you attending the lecture? | Yes | Yes |
11 | Jane | Yes, I am! | No | No |
12 | Henk | Yes, I am too! | No | No |
14 | Jan | No, I am on holiday | No | No |
16 | John | When will you be back? | Yes | Yes |
19 | Jan | I will be back tomorrow | No | No |
21 | Jane | Any of you know the answer to question 5? | No | Yes |
30 | Jane | ??? | No | No |
Thread layer
The layer responsible for coupling of related messages is also started on with the addition of a clustering algorithm called hierarchical clustering. The hierarchical clustering algorithm starts with each message as a separate cluster and looks for each iteration which messages have the least ‘distance’ between them and combines them into one cluster. This distance is determined by the euclidean distance function with the properties time and sender. The property time is used by computing the difference between each pair of messages, while the sender distance is determined by the distance function described in Distance function for clustering categories. For the hierarchical clustering algorithm a depth is expected which indicates the amount of iterations to cluster the messages. If this value is too low, very few messages will be clustered meaning no extra information while a high value will result in many questions clustered in the same cluster which is practically the same as not using clustering at all. A good depth is thus required to ensure a high entropy while the entropy will be low both if the depth is too high or low. From testing on the dataset below it is determined that expressing the depth in the amount of messages works better than giving a hard value. Furthermore, the depth worked best with a factor of three fourth. However, further refining is required on different datasets and when adding extra properties to the distance function. The results in the table show that there are two threads created. Especially the thread with index 2, since the differences in time are not as close as other messages, which shows that the sender distance function also does its work.
Time | Sender | Message | Thread id |
---|---|---|---|
0 | John | test | 0 |
1 | John | spam | -1 |
2 | Jane | this is spam | -1 |
3 | Jan | real message | 0 |
4 | Henk | real good message | 0 |
5 | John | Is this good? | 0 |
6 | Henk | hello! shall we go to the beach? | 0 |
10 | John | Are you attending the lecture? | 2 |
11 | Jane | Yes, I am! | 2 |
12 | Henk | Yes, I am too! | 2 |
14 | Jan | No, I am on holiday | 2 |
16 | John | When will you be back? | 2 |
19 | Jan | I will be back tomorrow | 2 |
21 | Jane | Any of you know the answer to question 5? | -1 |
30 | Jane | ??? | -1 |
GUI Design
To make for a pleasant way of interacting with the PA prototype a GUI was designed in parallel with the actual implementation of the PA.
For the first iteration the functionality of the GUI was kept fairly limited. The user is able to import chats as .txt files through the file manager of the OS, which the GUI then shows in a text area. The user is then given the choice which filters he/she want to apply to this chat. The last bit of interactivity this GUI offers is the actual button to run the PA on the imported chat with the selected filters. This has yet to be implemented in a future version of the prototype.
What follows then is a pop up dialog that notifies the user that the analysis has been completed successfully. Furthermore, a random score is generated and shown to the user to further give an idea of how the GUI should function.
Iteration 2
- Start on user preferences
- Preprocessing and normalization
- Bayesian network evaluation
- Recurrent Neural Network evaluation
- Prototype structure improvements
- Create results summary
- Reading of chat data
User preferences
To give the user the ability to express their own preferences regarding the degree of blocking notifications, creating threads and receiving feedback a user preferences object is created that stores all the preferences of the user. These preferences and settings can either be set by the user or can be altered by means of learning from feedback. The thread depth factor is an example of the latter, since the depth itself is not saying anything to the user. The user can however indicate that messages are coupled wrong in the sense of too few coupling or too many. With this answer the depth factor can be fine-tuned. Furthermore the preferences of the categorization layer are already present. These preferences indicate whether for example a question needs to be always blocked or allowed or needs to be automatically processed by the evaluation layer. This preference can be useful when a lot of questions are asked in a group that are not aimed at the user. The user preferences object can keep track of even more upcoming preferences of other layers in the future.
Preprocessing and normalization
The preprocessing that is done in the program consists of several different parts. Each part is described below. Some of the parts have to be done after classifying what kind of sentence it is, for example the removal of punctuation since it is important for question classification. Because of this the preprocessing is split up into two layers. The first one is the preprocessing layer and the second one is the normalization layer. The normalization layer is executed after the classification layer is done. This makes it so that the preprocessing is still done before determining the importance of a message but after the classification of what kind of sentence it actually is.
Messages contain a lot of meaningless words. They give the sentence structure but they do not have any influence on the meaning of the message. These words can thus be removed from the sentence before analyzing the importance of the message. The words that are meant here are for example: “the”, “a”, “an” or “to”. All these words are put into an array, then the sentence is checked whether or not it contains words of this array. When the sentence contains one or more of these words then they are deleted from the sentence and the leftover of the sentence is propagated to the next step of the preprocessing.
Translating verbs to their base form is part of the normalization. This will make evaluating a lot easier. The first step is to split the sentences up in words then the words will be checked whether or not it is a verb and then the verb will be put in its base form. To do this a library named JAWS is being used. In combination with the dictionary from wordnet the JAWS library is able to convert a verb to their base form. The next step is finding out how to find a verb in a sentence. This could be done using the Stanford pos tagger. When processing a sentence with this library every word in the sentence will be tagged. This tag will say what kind of word it is. For example a noun or a verb. But by doing this the program needs a lot of computation time since this database is very big. Therefore this is not implemented in the prototype. Because this did not work out for the program another solution had to be found. This solution was pretty simple after all. Because when the program processes every word in a sentence with the JAWS library it only changes the words that are actually verbs. The other words in the sentence are untouched. When the processing is done the only thing that is left to do is to put the words back in a sentence so they actually form a message again.
Translating the numbers to words is also a part of the preprocessing of the prototype and is done by using an existing class that translates numbers to words. The only thing left to do is to detect where the numbers are in a sentence and replacing them by calling the function in the existing class. The numbers in the sentence are found with a regular expression in java. This is a tool to find special characters or numbers really easy. When the numbers are replaced the sentence will be returned and put through the next step of the preprocessing.
Most of the punctuation in a sentence do not indicate the importance of a message, therefore it is good to remove the punctuation before evaluating the message. Punctuation is however important for determining whether or not messages are questions for example. Therefore this step of preprocessing will be done after classifying the message but before evaluating the importance. Removing the punctuation is a very simple task because the regular expressions in java can easily remove all the punctuation. When this is done the sentence will be propagated to the next step of the program.
Bayesian network evaluation
The first evaluation method that has been created is the naïve bayesian network evaluation. The library used to create a bayesian network is the Classifier4j library and is implemented as follows. The evaluation class consists of a Bayesian classifier and a word data source. While training the bayesian network the text of all messages is being teached to the classifier depending on whether the message is spam or not. The classifier will then process the text and keep track of the number of occurrences in spam and non-spam for that word. The evaluation of messages works by computing the probability of the message being spam or not depending on these saved occurrences by the training method. The storing and loading of the word data source is not supported by the library and is thus created. The storing, loading and training functionalities are elaborated on more below. The results of the bayesian network are shown in the table below and from these results the bayesian network evaluation seems very promising, since all but one sentence is classified correctly. This is however still on the dummy messaging data and in the next iteration real data from a Whatsapp group will be used that is classified by hand. The results are generated by the network with the following structure: first a pre-processing layer followed by a thread layer, a categorization filter and a normalization layer. Then comes the evaluation layer with the bayesian evaluation method. The sentence that is classified wrongly as spam is: “is this good?” which is similar to the sentence “real good message”. More training data would resolve the issue but could also cause the network to function less good since Whatsapp messages are generally very short without good grammatical structure.
Time | Sender | Message | Classified answer | Expected answer |
---|---|---|---|---|
0 | John | test | spam | spam |
1 | John | spam | spam | spam |
2 | Jane | this is spam | spam | spam |
3 | Jan | real message | spam | spam |
4 | Henk | real good message | spam | spam |
5 | John | Is this good? | spam | good |
6 | Henk | hello! shall we go to the beach? | spam | spam |
10 | John | Are you attending the lecture? | good | good |
11 | Jane | Yes, I am! | good | good |
12 | Henk | Yes, I am too! | good | good |
14 | Jan | No, I am on holiday | good | good |
16 | John | When will you be back? | good | good |
19 | Jan | I will be back tomorrow | good | good |
21 | Jane | Any of you know the answer to question 5? | good | good |
30 | Jane | ??? | spam | spam |
TP | 7 | FP | 0 |
FN | 1 | TN | 7 |
Total | 15 | ||
Precision | 1.0 | ||
Recall | 0.88 | ||
Specificity | 1.0 | ||
Accuracy | 0.93 |
Recurrent Neural Network evaluation
Furthermore, for the second visualization method Recurrent Neural Networks (RNN) have been looked at for the viability and while the training might be time consuming, neural networks have proved themselves to be able to analyze sequences of text or music very well. For this iteration a library for neural networks has already been chosen and a partial implementation is also already made. The library that is chosen is the DeepLearning4j library which supports a wide variety of neural networks for the Java programming language. While the library has a steep learning curve there are some good examples that show the implementation of a RNN on reviews where the network needs to categorize positive and negative reviews. The library works by setting up a network that expects so called word vectors. From these vectors the network is able to train and evaluate whether a message is spam or not. To be able to input the messages in the network, they first need to be transformed to word vectors, followed by a mapping onto the data structure that is expected by the library. The example uses a pre-trained database by Google of words to vectors, which is called the Google News dataset that ‘contains 300-dimensional vectors for 3 million words and phrases.’ This file is however 1.5GB of size and also requires a lot of memory to run the prototype. While testing 3GB of memory gave a out of memory exception and since it is desirable that the prototype can run locally on mobile devices this option is not possible. The next option was then to create a custom word to vector database that is aimed at the grammatical structure of messages. Since the structure is simpler and the vocabulary is much smaller in these messages, this custom database can be much smaller. For now the database is trained with a piece of text called ‘warpiece’, since the reading and classifying of chats is not done yet. With this custom dataset the network is able to run and only one message out of the fifteen could not be mapped to vectors, which is probably the message with only question marks. The network is now also able to be trained and evaluated but further work is needed to receive results out of the network.
Prototype structure improvements
This iteration the prototype structure is again improved, since it previously was difficult to read and change the structure of the layers because they needed to be written out from output to input. To solve this issue a class has been created that can receive layers in chronological processing order and the class itself will then link the individual layers. Furthermore the class also has easy to use methods that make tinkering with the structure very easy. This last improvement also comes into play when looking at how to save, load and train the complete prototype. Of course all layers need to be able to process the messages to get output but the saving, loading and training might differ from layer to layer. To solve this, layers can implement interfaces that indicate the storing feature or the training feature. When one of these methods are then called on the prototype, only the layers that can perform the saving, loading or training will actually do this.
Results summary
To be able to easily analyze the results of a chat evaluation some important numbers are calculated that express the performance of the prototype depending on the amount of true and false positives and negatives. These include the precision, recall, specificity and accuracy for now but can easily be extended to gain extra information.
Reading of chat data
Iteration 3
- Preprocessing and Normalization
- Recurrent Neural Networks Evaluation
- Integrate chat file parser
- Intermediate results
Preprocessing and Normalization
In this iteration the preprocessing is extended. A feature that replaces abbreviations with their full form is added. This is done by having all the abbreviations that are used in whatsapp in an excel file. Then this file is read by the program. The program uses Apache POI library to read the excel file. The messages come into the preprocessing and get split up. Then every word is checked whether or not it is in the abbreviation list. When it is it will get replaced by its full text. Then all the words in the message are put back together. The full messages will be propagated to the next part.
Recurrent Neural networks evaluation
The recurrent neural networks evaluation method has also been improved in such a way that the dummy data used earlier can be processed and gives correct results as output. However, when reading data in from real chats the evaluation method does not work flawlessly. This probably has to do with unstructured or unexpected messages that the prototype cannot cope with yet. The neural network itself can also be stored and loaded now. The results of the dummy data on the neural network are with an accuracy of 100%, which means that all 15 messages could be classified correctly after training. This is of course a small dataset but is an improvement on the bayesian network results.
Feedback structure
The way of giving feedback to the choice made by the network has also been created in this iteration. The way it works for message feedback is that there is a certain ‘uncertainty’ around the switching threshold of messages that are notification worthy and messages that are not. If the score of a message falls in this uncertainty range the message will be included in a feedback request that will be sent to the user. There are multiple options for the user to receive the feedback, such as through a notification, through a provided application on the phone or together with a batch of other feedback requests. Each layer in the network can listen for answered feedback requests of different types and will only take action when a predefined type is received. The types of feedback that are implemented for now are: message importance feedback, number of feedback requests, amount of blocked messages and the number of threads. Some of these feedback requests can be sent out autonomously from a layer in the network, while a different feedback request might be sent out on a timely basis. In the figure to the right the feedback structure can be seen. If for example a message falls in the uncertainty range during the evaluation it will be send to the feedback manager which in turn will propagate the message feedback request towards the user. After the user gave the feedback, the message will be received by the feedback manager and will be send to the evaluation layer where all evaluation methods can train and process the given feedback. In case feedback other than message importance is received by the feedback manager, it will propagate the feedback results towards the preferences. The preferences contain all hyperparameters that can be improved by learning from the user.
Integrate chat file parser
The Whatsapp chat file parser has also been integrated into the prototype. The parser can read Whatsapp chats that are exported from Whatsapp through the ‘send chat by email’ option. This will send a chat in a text (.txt) format containing the date and time, the sender of the message and the message itself. Since the date format is different for different languages and regions the parser should also be able to cope with the different formats. For now the parser supports the United States, United Kingdom and the Dutch format which are the most common formats for the group chats that are analyzed for this prototype. Furthermore the parser also keeps track of a ‘contact book’ since the prototype wants to know which messages are coming from the same contact. This is especially important for the Thread layer.
Intermediate results
In this section the intermediate results of the current iteration prototype are shown. For now there are two English chats that are classified by hand and can thus be used to train and evaluate the prototype. The first dataset consists of 178 messages with 146 notification worthy and 32 not notification worthy. The second and larger dataset consists of 1505 messages of which 959 notification worthy and 546 not notification worthy. There are more chats present but these need to be classified by hand first to say something about the results.
The network that is used to generate the following results is the following:
Preprocessing -> Threads -> Categorization -> Normalization -> Evaluation (Bayesian) -> Output
The following hyperparameters are used:
Batch Size | 200 |
Thread depth | 0.75 |
Evaluation Threshold | 0.5 |
Evaluation Uncertainty | 0.0 |
The first small dataset processed on a network trained on the same dataset took 5.3 seconds to train and process:
TP | 145 | FP | 5 |
FN | 1 | TN | 27 |
Total | 178 | ||
Precision | 0.97 | ||
Recall | 0.99 | ||
Specificity | 0.84 | ||
Accuracy | 0.97 |
Although the network is trained on the same dataset, these results show that the bayesian network can definitely distinguish between messages. The fact that the number of false positives is relatively high is not too worrying since a higher percentage false positives is better than a high percentage of false negatives. People would rather receive messages that are not too important than miss out on important messages.
The larger dataset processed on a network trained on the same dataset took 24.3 seconds to train and process:
TP | 929 | FP | 171 |
FN | 30 | TN | 375 |
Total | 1505 | ||
Precision | 0.84 | ||
Recall | 0.97 | ||
Specificity | 0.68 | ||
Accuracy | 0.87 |
These results show that the network is performing a little bit less on a larger dataset while it has been trained on the same large dataset. It is however still more desirable to have more false positives than false negatives.
The small dataset processed on a network trained on the large dataset took 3.9 seconds to process:
TP | 132 | FP | 7 |
FN | 14 | TN | 25 |
Total | 178 | ||
Precision | 0.95 | ||
Recall | 0.90 | ||
Specificity | 0.78 | ||
Accuracy | 0.88 |
Compared to the results on the trained small dataset network this test did perform a little bit worse, which is understandable since the network did not see the 178 messages ever before. For this reason these results are still very promising and with even more fine tuning they could be improved even more. What can be seen from the data is that especially the number of false negatives increased which is not desirable for reasons described earlier in this subsection.
Iteration 4
- Recurrent Neural Networks Evaluation
- GUI
- Android App
Recurrent Neural Networks Evaluation
This iteration the RNN evaluation has been improved and problems have been resolved. The problem that caused the RNN evaluation not to work with parsed chat data was caused by having more messages in a chat compared to the number that could fit in a batch. This should normally not be a problem but the library used does not like this. Furthermore a problem was caused by the varying number of words in a single message. This caused the results to be not very accurate. After both of these problems were solved the network could train and process the parsed chats. The network then was trained on the large dataset that is used before. The number of epochs that the network is trained on is 60 which already took a long time. The accuracy for the trained model was then around 80% for the same dataset as the network was trained on. At this point it was decided that the focus would shift to the user interface since the performance of the messages evaluation is too slow to run and will be even slower on mobile phones. Furthermore the performance of the bayesian evaluation already proved to be more accurate. With more tweaking the Recurrent Neural Network could probably achieve a better accuracy and could overtake the bayesian variant by also looking at the contextual meaning and threads of messages.
GUI
To be able to present the working prototype to users or other stakeholders a user interface needs to be made that can clearly show the performance, actions and features of the prototype. With this in mind the main focus of the user interface was to build a program that can run on the PC. To test and show the performance on mobile phones an android app has been made. Both interfaces are described below.
PC application
The PC application is of course able to open and process chats and display whether the prototype evaluated a message as notification worthy or not notification worthy. This is done by giving the messages a red or green background. To show the accuracy for each message, a colored box has been added to the left of the date to resemble the result evaluated by hand. This box conceals itself in the background color if the message has been evaluated right and will show a different colored box if the message has been classified wrongly. To the left of this box a number is present that indicates the thread index. The messages with the same number belong to the same thread and messages with negative one as thread index do not belong to any thread. The messages themselves are displayed in a usual way with the date at the front followed by the name of the sender and finally the message text itself.
These messages are selectable. When a message is selected the user is able to give feedback by clicking the button “useful” or “not useful”. These buttons are located in the bottom right of the gui. The program takes this feedback into account. When it is ran again, the program improves its message importance classification. When the program is not sure about a message it asks for feedback. This is done using a popup window. The popup window displays the message and for each message a “useful” and “not useful” button. When the user clicks either one of those boxes the message will disappear from the window and the feedback is taken into account.
Android App
The android app does not have all functionalities as the PC application like giving feedback but has been made as a proof of concept to show that the prototype is able to run on a mobile phone and intercept notifications and show only the useful ones. The user interface for the messages looks very similar to the one on the PC with the thread index at the start, followed by the box showing the classification by hand followed by the real message. An additional feature is that a user can enter its own new messages without loading a chat to check the results of the prototype. When the messages have been classified the background gets set to the same colors as for the PC application and unimportant messages themselves can be hidden from the list. The last additional feature that is experimental and needs to be enabled separately is to process real Whatsapp notifications while they are coming in in real-time. This feature resembles how the prototype would work when it is implemented as an actual product.
User evaluation of the prototype
In order to receive real world feedback from people that where actually members of a certain Whatsapp group. A survey has been conducted where recipients were asked to classify 32 messages by hand. Because the original group only consisted of 4 people, including one of the members from this research group (in order to obtain unbiased results that person did not fill out the survey), the number of responses was also very limited. Only 2 people filled out the survey, so there isn’t much value in the results. When calculating accuracy of the classifier, the occurrence of conflicting results heavily influences the outcome. E.g. the program only classifies each message as spam or ham, whereas messages where one person regarded the message as spam and the other as ham results in a value of 0.5. this should be regarded as a false positive if the message is classified as ham by the program because the classification is wrong, but it also results in a false negative if the message would be regarded as spam. In order to overcome this, these conflicting survey results will be regarded as one half true positive and one half false positive (in the case of ham classification by the designed classifier).
Results The accuracy test of both survey results combined yielded:
TP = 14.0, FP = 7.0, T +P = 32.0
Accuracy = 75%
When only one participant was used(no conflicting results) the highest accuracy test yielded:
TP = 19.0, FP = 2.0, T+P = 32.0
Accuracy = 90.6%
Conclusion Overall when the results of the evaluation of the classifier are reviewed according to all responses to the survey they might not be that high. The researchers believe that the cause of this is most likely the amount of influence the initial classification of the training data had on the results of the classifier. This initial classification was done by hand which by default is subjective (relevance of important messages is quite subjective, especially when only performed by one person). When initial classification would be averaged over multiple persons classifying the same data by hand, this would decrease the subjective nature because of the principle of the “wisdom of the crowd”. The response with the highest accuracy shows that the program at least seems to have got it right for one person. If there would have been more evaluation results the degree of belief in this might be higher, for now no clear conclusions can be made.
Final Deliverables and Results
Java Application
The final deliverable for the java application is a program that is able to run the personal assistant and by which the user is able to visualize, interact and process different chats and see the created message threads and performance of the prototype. The prototype has the feedback feature implemented by which users can give feedback and the prototype can learn from this. The source code for this java application can be found here: Personal Assistant GitHub
Android App
The android app does have the feedback feature but has some other features. The android app does have a feature to read the incoming notifications. The app is able to run the program on these real-time notifications and then gives a notification where necessary. The android app also supports a feature which makes it possible to type your own messages and classify them. The app will then determine whether or not a notification would be given for this message. The apk file for this android app can be found here: Personal Assistant Android App GitHubPersonal Assistant APK GitHub
Results
To see how well the program functioned, it was trained and tested on various WhatsApp chats. In total there were 5 different chats used, varying in size. The shortest chat contained 178 messages, the longest 2619 messages. In total, 6461 messages were evaluated.
The accuracy varied based on which chat the program was trained. Although the accuracy achieved on each chat individually heavily depended on which chat the program was trained on, the total accuracy varied less. When trained on the shortest chat the accuracy was logically speaking the lowest, ranging from 0.704 to 0.966. The accuracy of 0.966 was achieved when the same chat that was used for the training was evaluated by the program. The average accuracy was 0.806 The highest accuracy was achieved when the program was trained on a combination of multiple chats. In this case it ranged from 0.809 to 0.912. The average accuracy was 0.867.
For the processing times, the program on average takes 0.011 seconds to classify a single message and for the model with the combined training chats that performed best the average time it takes to classify a single message is 0.013 seconds rounded to 3 decimals. This comes down to processing around 90 messages per second on average and 77 for the best performing model both on a single thread. The processing times are determined on a Intel Core i7-6700HQ Processor.
The processing times for the prototype on a mobile device are 0.027 seconds for the model with the best performance. This comes down to about 37 messages per second on a single thread which should be enough for most if not all people in the target group. Furthermore the processing of messages could probably be improved in terms of processing times. The prototype test on a mobile device has been tested on a Snapdragon 845.
In general we can say that when the program is trained on more messages, it will perform better on average. If it is however trained on a smaller set of messages, it is better at classifying certain specific messages, but it will perform less overall. The full results can be seen in the table below:
Chat | Total messages | Important messages | Not important messages |
---|---|---|---|
Chat 1 | 2619 | 2192 | 427 |
Chat 2 | 178 | 146 | 32 |
Chat 3 | 378 | 278 | 100 |
Chat 4 | 1505 | 959 | 546 |
Chat 5 | 1776 | 1437 | 339 |
Processed on | Trained on | Process time | True Positives | False Positives | False Negatives | True Negatives | Accuracy |
---|---|---|---|---|---|---|---|
Chat 1 | Chat 4 | 32.27s | 2027 | 213 | 165 | 214 | 0.856 |
Chat 2 | Chat 4 | 1.90s | 133 | 6 | 13 | 26 | 0.893 |
Chat 3 | Chat 4 | 4.02s | 237 | 27 | 41 | 73 | 0.820 |
Chat 4 | Chat 4 | 16.16s | 943 | 185 | 16 | 361 | 0.866 |
Chat 5 | Chat 4 | 19.98 | 1317 | 165 | 120 | 174 | 0.840 |
Chat 1 | Chat 2 | 30.63 | 2005 | 225 | 187 | 202 | 0.843 |
Chat 2 | Chat 2 | 1.92s | 145 | 5 | 1 | 27 | 0.966 |
Chat 3 | Chat 2 | 3.98s | 239 | 46 | 39 | 54 | 0.775 |
Chat 4 | Chat 2 | 16.12s | 884 | 370 | 75 | 176 | 0.704 |
Chat 5 | Chat 2 | 18.92s | 1306 | 175 | 131 | 164 | 0.828 |
Chat 1 | Chat 3 | 33.03s | 1958 | 179 | 234 | 248 | 0.842 |
Chat 2 | Chat 3 | 1.97s | 131 | 6 | 15 | 26 | 0.882 |
Chat 3 | Chat 3 | 4.07s | 265 | 12 | 13 | 88 | 0.934 |
Chat 4 | Chat 3 | 16.34s | 810 | 270 | 149 | 276 | 0.722 |
Chat 5 | Chat 3 | 19.88s | 1257 | 148 | 180 | 191 | 0.815 |
Chat 1 | Chat 1 | 33.15s | 2176 | 228 | 16 | 199 | 0.907 |
Chat 2 | Chat 1 | 1.84s | 137 | 13 | 9 | 19 | 0.876 |
Chat 3 | Chat 1 | 4.07s | 251 | 51 | 27 | 49 | 0.794 |
Chat 4 | Chat 1 | 16.18s | 929 | 412 | 30 | 134 | 0.706 |
Chat 5 | Chat 1 | 23.09s | 1387 | 221 | 50 | 118 | 0.847 |
Chat 1 | Chat 1 + Chat 3 | 35.17s | 2175 | 214 | 17 | 213 | 0.912 |
Chat 2 | Chat 1 + Chat 3 | 2.01s | 138 | 9 | 8 | 23 | 0.904 |
Chat 3 | Chat 1 + Chat 3 | 4.01s | 251 | 45 | 27 | 55 | 0.810 |
Chat 4 | Chat 1 + Chat 3 | 16.98s | 946 | 275 | 13 | 271 | 0.809 |
Chat 5 | Chat 1 + Chat 3 | 24.63s | 1374 | 189 | 64 | 150 | 0.858 |
Chat 1 | Chat 5 | 29.19s | 2119 | 251 | 73 | 176 | 0.876 |
Chat 2 | Chat 5 | 1.84s | 137 | 12 | 9 | 20 | 0.882 |
Chat 3 | Chat 5 | 3.80s | 247 | 50 | 31 | 50 | 0.786 |
Chat 4 | Chat 5 | 15.74s | 913 | 413 | 46 | 133 | 0.695 |
Chat 5 | Chat 5 | 19.30s | 1429 | 158 | 13 | 181 | 0.904 |
Conclusion
To conclude, the research done into a personal assistant for managing notifications showed that messages can be analyzed for relevance with a decent accuracy. The average accuracy of 0.867 shows that there is some structure in messages that are relevant and ones that are not. These results are achieved by using a Bayesian classifier which is fairly straightforward to setup and to train on the incoming messages. Furthermore it is also fairly fast both on a pc and on a mobile phone as has been discussed in the results section. The implementation of a Recurrent Neural Network proved to be a little bit harder and while it did work in the end, the performance and the accuracy did not exceed that of the Bayesian classifier. This is why it was decided to leave the RNN out of the prototype structure. A survey done to analyze the target audience showed that there is a reasonable group of students that would like a feature like our prototype in their messaging application. Of course the results of the evaluation on messages that are classified by the same people as the training messages, are not the only performance metric to be analyzed and thus a survey was setup that showed that one participant had a very good accuracy while a different participant had a lower accuracy on the same messages. Since not many participants responded to the survey no further conclusions could be made. Finally, the real-world application of the prototype has been implemented as an option for the Android app that will analyze incoming message notifications of any messaging platform and will determine whether it is notification worthy or not. The feature will hide all notifications of the messaging platform, like Whatsapp, and will show its own notifications if an important message has been sent.
Potential Improvements for the Future
So while satisfied with the results, a lot of improvements can still be made to the PA in its current form. At the start of this project the PA had many more elaborate features that proved to be difficult or not time-feasible to implement. This section will answer the question: ‘how could the PA be better’? The obvious answer would of course be to make it run faster and smoother, but during the process a lot of ideas were considered but deemed too difficult or not important enough for now. The most interesting ideas are discussed below.
As mentioned before, the PA was originally meant to take over tasks of the user, such as responding to messages or automatically adding appointments to the user’s agenda. This is still an interesting feature to consider, especially since the processing already goes through all of the Whatsapp text messages. Being able to recognize simple questions in the form of ‘what time will you be home?’ and responding to them based on what’s in the agenda of the user is still something that seems useful and without major downsides. It speaks for itself that this can be turned off in the event that the user does not want this.
Something that we struggled with immensely was taking into account the context of messages when classifying messages. We often discussed context with regard to the relationship between sender and receiver. It was fairly clear that a message received from the lecturer is considered more important than a message that was sent to you by the average student. However actually incorporating this into our project proved to be too difficult, both in implementation and defining these relations. Hence we decided to limit ourselves to threads that dealt with time differences between messages instead of looking at the sender/receiver relationship.
While a good working Java GUI and Android app were developed and fully working, these served mainly as chat analyzers. Not a lot of implementation was devoted to it being incorporated into Whatsapp itself, with the green/yellow/red color scheme that was present in both the Java GUI and Android App. Simply put, Whatsapp with these systems integrated would perhaps have been a more elegant final product. Nevertheless, the group is confident that with the current systems available this is definitely feasible.
Finally, evaluation of chats is currently done by a Bayesian Network only. At one point, both a Recurrent Neural Network (RNN) and a Bayesian Network were up and running, with the goal of combining them into one evaluation function. However, while the Bayesian Network generally gave very good results of around ~90% accuracy, the RNN did notably worse at around ~75-80% accuracy. Consequently, the decision was made not to include the RNN into the final product, as this would cause a regression in the quality of chat evaluation. It is still an interesting concept to consider for future implementations, as we strive for the highest possible accuracy when evaluation is done.
References
- ↑ https://www.emeraldinsight.com/doi/full/10.1108/IMDS-05-2017-0214
- ↑ https://www.sciencedirect.com/science/article/pii/S0262407913600925
- ↑ https://search.proquest.com/docview/1704945627/50DE051B4E904379PQ/4?accountid=27128
- ↑ https://www.ri.cmu.edu/pub_files/pub1/mitchell_tom_1994_2/mitchell_tom_1994_2.pdf
- ↑ http://www.aaai.org/Papers/Symposia/Spring/2000/SS-00-01/SS00-01-023.pdf
- ↑ https://ieeexplore.ieee.org/document/8273196/
- ↑ http://www.aaai.org/Papers/Workshops/2008/WS-08-04/WS08-04-004.pdf
- ↑ https://link.springer.com/chapter/10.1007/978-1-85233-842-8_10
- ↑ http://ijifr.com/pdfsave/10-05-2017475IJIFR-V4-E8-060.pdf
- ↑ https://patents.google.com/patent/US6792082B1/en
- ↑ http://papers.cumincad.org/data/works/att/d5b5.content.06921.pdf
- ↑ https://s3.amazonaws.com/academia.edu.documents/39232090/0c9605226714eeedad000000.pdf?AWSAccessKeyId=AKIAIWOWYYGZ2Y53UL3A&Expires=1524910399&Signature=BjYwD6z0FRQMoazG%2BSJHjHc8nZQ%3D&response-content-disposition=inline%3B%20filename%3DA_personal_email_assistant.pdf
- ↑ https://patents.google.com/patent/US20140337814A1/en
- ↑ https://homes.cs.washington.edu/~weld/papers/cacm.pdf
- ↑ http://www.aclweb.org/anthology/W16-3628
- ↑ https://ieeexplore.ieee.org/document/8279618/
- ↑ https://ieeexplore.ieee.org/document/8251876/
- ↑ https://ieeexplore.ieee.org/document/7150798/
- ↑ https://books.google.nl/books?id=sRqlLLOboagC&lpg=PA246&dq=artificial%20intelligence%20personal%20assistant%20administrative%20tasks&hl=nl&pg=PR2#v=onepage&q=artificial%20intelligence%20personal%20assistant%20administrative%20tasks&f=false
- ↑ https://www.fungglobalretailtech.com/research/ai-personal-assistants-will-change-lives/
- ↑ https://hbr.org/2016/11/how-artificial-intelligence-will-redefine-management
- ↑ http://www.icdk.us/aai/public_administration
- ↑ https://ieeexplore.ieee.org/document/7940206/
- ↑ https://ieeexplore.ieee.org/document/7382023/
- ↑ https://ieeexplore.ieee.org/document/5090166/
- ↑ https://arxiv.org/abs/1703.01898
- ↑ http://journals.sagepub.com/doi/pdf/10.1177/0165551515616310
- ↑ https://link.springer.com/content/pdf/10.1007/s10489-018-1161-y.pdf
- ↑ http://www.surdeanu.info/mihai/teaching/ista555-spring15/readings/yang97comparative.pdf
- ↑ http://citeseerx.ist.psu.edu/viewdoc/download;jsessionid=B2FB1F51D66DC0D90FA785BB9D087172?doi=10.1.1.44.562&rep=rep1&type=pdf
- ↑ http://www.aaai.org/Papers/AAAI/1999/AAAI99-063.pdf
- ↑ https://search.proquest.com/docview/2017545987/B4CAA5405B794CA8PQ/1?accountid=27128
- ↑ https://search.proquest.com/docview/1270351132/B4CAA5405B794CA8PQ/3?accountid=27128
- ↑ https://patents.google.com/patent/US7320020B2/en
- ↑ https://securityintelligence.com/the-art-and-science-of-how-spam-filters-work/
- ↑ https://www.cs.ru.nl/bachelorscripties/2009/Martijn_Sprengers___0513288___The_Effects_of_Different_Bayesian_Poison_Methods_on_the_Quality_of_the_Bayesian_Spam_Filter_SpamBayes.pdf
- ↑ https://www.sciencedirect.com/science/article/pii/S095741740900181X