PRE2024 3 Group8
Margot Dijkstra, Llywelyn Vrouenraets, Sem Schreurs, Vladis Michail, Alessia-Maria Postelnicu, Sebastian Ciulacu
Approach, milestones and deliverables can be found on the first page of the logbook called Deadlines
Report progress:
Week 2: For the second week our main task was to refine our project based on the feedback we received at the Monday tutor session. We held a meeting to discuss the possible directions we can take our research in and settled on educational robots for vocabulary learning. Following this, we divided the work needed to update our report and worked in pairs again. The three main tasks were 1. Updating the problem statement and objectives, 2. Writing an ERB form to be sent for ethical approval, 3. Specifying our state of the art findings to be more specific about Robot-Assisted Language Learning (RALL).
Week 1: For the first week we all looked for relevant research articles and ended up with 25 in total. Then, each one of us summarized the articles with the most relevant bullet points and we had a one hour meeting to discuss on Friday 14th. At this meeting, we subdivided the work needed to update the wiki into sections and worked in pairs to accomplish that.
You can find the individual time contributions on the second page of the logbook called Time Use
Upcoming meeting agenda: Meeting agenda Monday 2025-02-24.docx
Week 3 Updates
Questionnaires to be used after experimental trial
Cognitive Load Questionnaire (Paas, 1992) - (1 question). We would like to see whether our participants experienced a difference in cognitive load based on which tutoring agent they received. We believe that the video recording of the robot may potentially cause extraneous cognitive load and in turn reduce the cognitive resources available to learner to integrate information - in this case new vocabulary - with existing knowledge structures. This is because virtually presented agents could add additional processing to the environment in terms of visual or audio distraction (Craig & Schroeder, 2017).
How much mental effort did you apply in completing the vocabulary learning task?
Please choose the category (1, 2, 3, 4, 5, 6, 7, 8, or 9) that applies to you:
9-point likert Scale (1 = very, very low mental effort; 2 = very low mental effort; 3 = low mental effort; 4 = rather low mental effort; 5 = neither low nor high mental effort; 6 = rather high mental effort; 7 = high mental effort/ 8 = very high mental effort; 9 = very, very high mental effort
Re-test Reliability= .90
Robot Social Presence (Chen et al., 2024) - (19 questions). We are interested in seeing whether our participants judge the social presence of the robot tutor differently to the social presence of the on-screen agent. Decreases in social presence have been negatively related to the impact and retention of presented information in online learning environments (Tu & McIsaac, 2002). Thus, for us it would be interesting to measure if physically embodying the tutor inside the robot will increase the judgement of social presence which could in turn potentially increase the retention of information.
Godspeed Questionnaire Series (Bartneck, 2008) - (24 items). We chose to include this questionnaire to measure the general impression that the participants had of the robot across the dimensions of Anthropomorphism, Animacy, Likeability, Perceived Intelligence and Perceived Safety. This will allow us to better understand whether the participants enjoyed the experience with either agent in the first place and whether there is a relationship between their judgement and performance on the learning task.
Getting acquainted with the NAO robot
A trial using the Choregraphe screen-based app was executed this week to get acquainted with the motions and the audio functions of the robot. The motions seem to be executed smoothly, but on the real-life robot, some mobility and equilibrium issues might be encountered. The audio did not function in the screen-based application, only a text bubble containing the assigned script wad displayed above the NAO robot, so the auditory aspect will be assessed on the physical robot.
Week 2 Updates
Problem statement and objectives:
It is no overseen issue that there is a shortage of teachers within education. School districts are scrambling to find certified teachers, especially in world languages (Hanford, 2017; Koerting, 2017; Motoko, 2015). This is a problem that the Technical University of Eindhoven faces as well. After a small investigation, the following came to light: International students who want to make use of the Dutch classes provided by the university are disappointed time and time again since the demand simply cannot be met. There are way too many students, and yet there is a huge shortage of opportunities for them to take the Dutch classes they want to take. This also means that if they manage to get into such a class, they are usually overfilled with students, often offering low one-to-one time with the tutor. Those who do not, however, end up being put on a waitlist with no clear indication of whether they will be able to successfully sign up for a language course in the desired quartile.
To tackle this issue, we will look towards HTI and robotics. We believe robotics can be a good tool to handle this aforementioned problem as it can be used to assist tutors in their teaching. With robotic assistance, tutors can divide their attention while the robot takes over certain tasks, increasing the opportunities for quality learning. The objectives will be to conduct research in the direction of providing students with a robotic tutor in hopes of seeing whether this could provide an adequate solution to this problem. We aim to help increase the opportunity for students to attend foreign language classes, even in times with high demand for such classes and a low supply of tutors.
Who are the users?
University students often face high cognitive loads throughout their studies, meaning that it is crucial to support them by providing efficient learning and retention strategies. In our problem statement, we mention international students at the TU/e who want to study Dutch, but this concept can be applied to a wide audience, consisting of all universities with a large number of foreign students. This means that the main user group that would benefit from our research findings, as well as the future development guidelines that may stem from it is more general. This would be any university student trying to study a foreign language other than their language of study.
What do they require?
The university students require opportunities to learn the language of the country that they are staying in. We believe that giving these students the opportunity to learn (predominantly vocabulary) through a robot, will increase the capacity of the university to meet this demand. In this way, more students would be able to learn the local language, aiding their integration into the culture.
The main requirements of the users that we aim to cater to in this research are to provide them with supervision and smaller groups. Supervision has been found to be very influential, as it increases the general effectiveness of learning (Holloway, 1992). It makes way for structured and repeated learning, giving the students a clear way of doing things, as well as a clear timeslot to do it in. This is why we expect robotic tutors to be more effective than language-learning apps, the like of Duolingo, which provide supervision by means of mobile notifications, rather than physical presence. Jones (2007) promotes smaller group sizes since it promotes active participation, and deep rather than surface learning. One of the problems, however, is that it states that small groups typically require greater investment in key resources such as staff and meeting rooms.
State of the art
In recent years, advancements in robotics have led to the development of social, language learning robots designed to enhance the way people learn new languages. Currently, the most prominent state-of-the-art robots of this kind have children and school-aged children in mind as the main user base. Among the most advanced in this field are EMYS, Elias, and SKoBots, each offering unique approaches to interactive language education.
EMYS is an expressive robot specifically designed for young, mainly bilangual, language learners, typically aged 3 to 7. It has a mechanized face with three moving discs, somewhat resembling a turtle, that allow it to display emotions. The robot comes with a set of cards that children can use, pictures of animals and such, to expand their vocabulary.
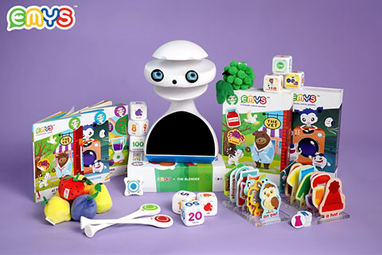
Elias Robot, on the other hand, is designed primarily for classroom environments and provides AI-powered conversational practice in multiple languages. Its build is essentially that of a Nao robot. It integrates with educational systems and tablets, allowing teachers to customize lessons and track student progress via a specialized app, in which educators can make the necessary choices. It appears to have more functions than EMYS as it can complete more language-learning oriented tasks, such as pronouncing out loud a specific sentence, and also has a range of physical movements that it can do, such as dancing. Still, it appears that the EMYS robot is more emotionally expressive, while Elias is more bodily expressive.
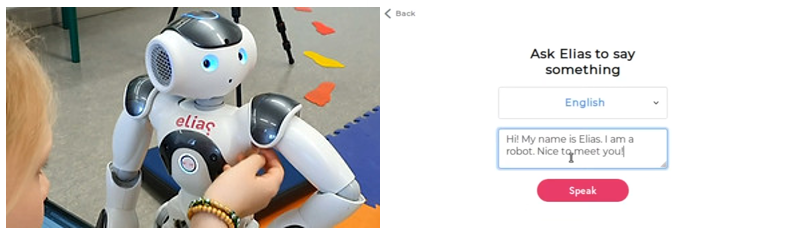
SKoBots represent a more niche, yet more exciting venture in language learning robots than the previous two projects. It is a 3D printed companion that can sit on the user’s shoulder and assist its mainly Native American user base with learning a Native American langage. This way, SKoBots cater to a wider range of users, including older students and independent learners. Most of the sample videos show the robots assisting Native American teens with strengthening their vocabulary and providing dynamic, real-time corrections. One of the admirable aspects of this robot is its unique design, ease of assembly and a low price point, making it accessible to marginalized communities.
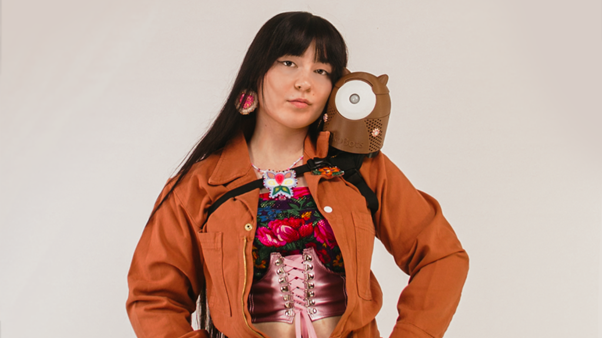
Van Den Berghe et al. (2018): In this review of Social Robots for Language Learning, the researchers suggest that children may be able to learn words from robotic agents equally well as from human teachers, or when receiving assistance from robotic agents of other children peers. For summary of the included study see below:
Zinina et al. (2022): In this study, university-aged linguistics students were asked to practice vocabulary learning in Latin (a language that was foreign to them) and were subsequently asked to evaluate their experience and the performance of the robot as an assistant tutor. The assisance entailed the robot giving the learners words in their native language, in this case Russian, that are most phonetically similar to the Latin words being asked to study. They judged to robot to give a positive impression, and reported increased motivation and desire to use robot-assisted learning in the future.
Deng, Qi et al. (2024): in this review of Robots Assisted Language Learning (RALL) for adults, the researchers suggest that interacting with robots allows learners to speak in a low-stress environment, improving fluency and confidence. Additionally, it was found that being taught by a physical robot, in contrast to one on video, enhanced performance on a vocabulary test and improved engagement. The study by Van den Berghe et al. (2021) used Brain-Computer Interfaces to provide adaptive feedback which the robot used to maintain the participant’s attention. However, there were some studies indicating that the introduction of social robots did not significantly improve learning outcomes. Lastly, using implicit teaching methods, such as conversational, could improve grammar and some studies found that it also improves pronunciation.
Week 1 Updates
Problem statement and objectives:
The nature of the world around us is everchanging, technology evolves exponentially and developments in computational power reshape the reality around us, therefore it is essential to understand how these changes affect us and how we can develop technology that contribute to flourishing of the human species. Scenarios that seemed to belong to Science Fiction novels started to be implemented among robotic technology developers. Understaffed fields that are less appealing to the broad public seem to benefit from the attention of these developers, applications such as care robots, educational assistant robots, and factory robots are popular topics of robotics enthusiasts. An aspect that is frequently overlooked lays in the depths of their interaction with people, where characteristics that are intrinsically humane, like social norms, trust, meaning, culture and emotions play a central role. Learning and education are a significant aspect of the human experience and contributes to the development of our species, therefore this study will focus on Human-Robot Interactions (HRI) in the context of education, with an emphasis on the way information is delivered to the human receivers. Being a good educator is complex and involves many underlying characteristics, therefore this exploration will tackle a superficial layer of the depths of what it takes to be a teacher, namely we will look at what embodiment of an agent that delivers a material provides the best recall of the content.
Who are the users?
The main user group that would benefit from our research findings, as well as the future development guidelines that may stem from it, is university students, as they are the primary subgroup of students gradually encountering more robot-assisted learning environments (e.g., Pepper being used as a teaching assistant at Carnegie Mellon University). University students often face high cognitive loads throughout their studies; therefore, it is crucial to support them by providing efficient learning and retention strategies. In the case of a robot teaching a student, such a strategy would be to assign the most suitable type of agent for the learning context.
What do they require?
For a university student it is important to have access to effective and adaptive learning strategies that can help them manage the high cognitive loads and improve their retention of information. In the context of robot-assisted education, this means that the robot that works with the student needs to maintain a high level of engagement while facilitating understanding and optimizing recall. The content and context of the environment in which the robot and student are based need to be taken into account as different situations will require different types of agents. Characteristics such as interaction style, verbal and non-verbal communication, and adaptability need to be clearly determined to best support learning. Additionally, students can benefit from personalized and interactive learning, in which case robots can adjust their approach based on the individual's learning needs.
Useful vocabulary and theories:
The voice is an expressive aural medium of communication, it can be viewed as the "how" of vocalizations. Nonverbal paralinguistic properties that characterize the voice, such as tone, loudness, pitch and, timbre, are called vocalis. Speech is the linguistic content of the voice, primarily consisting of words, grammar, syntax and phonetics (Seaborn et al., 2021).
The voice Effect assumes that people learn better when they are exposed to multimedia instruction that includes a human voice rather than a machine-synthesized one (Craig & Schroeder, 2017). Recorded human voices provide an experience that is easier to identify as a social interaction, thus promoting the active learning process. This can be explained using cognitive load as machine voices may cause extraneous cognitive load and reduce cognitive resources available to integrate information with existing knowledge structures. The cognitive load could also be increased because could add additional processing to the environment in terms of visual or audio distraction.
(- Voice: an expressive aural medium of communication. Is the “how” of vocalizations (Seaborn et al., 2021).
- Vocalics: nonverbal paralinguistic properties – tone, loudness, pitch, timbre and nonverbal prosodic properties – rhythm, intonation and stress. They characterise the voice (Seaborn et al., 2021).
- Speech: linguistic content of voice, primarily comprising words, grammar and syntax, and phonetics. Is the “what” of vocalizations (Seaborn et al., 2021).
- Voice Effect: Assumes that people learn better when they are exposed to multimedia instruction that includes a human voice rather than a machine voice (Dincer 2022).
Perspectives on why learning with a recorded human voice may be more effective than learning from a machine-synthesized one (Craig & Schroeder, 2017):
1. Cognitive load:
a. Machine voices may cause extraneous cognitive load and so reduce the cog resources available to learner to integrate information with existing knowledge structures.
b. Virtual humans could add additional processing to the environment in terms of visual or audio distraction.
2. Social agency:
a. Recorded human voice provides an experience that is easier to identify as a social interaction, thus promoting the active learning process.)
Auditory Encoding and Short-Term Recall
The study by Colle (1980) supports the central masking hypothesis, suggesting that auditory noise interferes with visual recall because the speech loop must pass through the preperceptual auditory store, where it gets masked by noise. This aligns with the idea that AI-generated speech, with its inconsistent flow and unnatural pauses, could function as a form of "structured noise," disrupting inner dialogue and reducing recall ability.
Topic Interest and Incidental Learning
Cancino’s (2019) research highlights how topic interest significantly influences vocabulary retention in incidental learning settings. This effect is mediated by cognitive processing depth and dictionary use.
Auditory vs. Visual Short-Term Memory
Tillmann & Caclin (2021) provide evidence that auditory memory generally outperforms visual memory, especially for materials with a clear auditory contour. This suggests that structured auditory stimuli might enhance recall, whereas less structured sounds (like AI speech with unnatural intonations) could have the opposite effect. A comparison between human and AI voices could further validate this.
Auditory Similarity Effects in Recall
The study by Connor & Hoyer (1967) reinforces the idea that phonological (auditory) similarity affects recall more than visual similarity. This suggests that if AI-generated speech has distortions or inconsistencies, it might interfere with phonological encoding, reducing recall accuracy.
AI Voices and Multimedia Learning
Mayer (2014) emphasizes that human voices enhance learning more than machine voices, as they foster a sense of social presence. However, McGinn & Torre’s (2020) study found that high-quality AI voices can be indistinguishable from human voices and do not necessarily impact learning outcomes. This is corroborated by Craig and Schroeder (2017) as well as Dinçer (2022), the latter specifically finding no cognitive load differences when using a modern synthetic voice and human speech.
Embodiment and Perception in Human-Robot Interaction
Studies by Wainer et al. (2006) and Seeger et al. (2018) show that physical embodiment enhances social presence and perception. However, the effect is nuanced since nonverbal cues alone can decrease perceived anthropomorphism due to the uncanny valley effect. If AI-generated speech is paired with a robotic presence, the combination of physical embodiment and voice type could influence recall.
Embodiment and learning
The presence of pedagogical agents increases learning outcomes against no-embodiment conditions (static agents and/or no-agent conditions) and no-agent conditions. Embodied agents, those that posses human-like characteristics such as facial expression, gestures, lip synchronization, and body sway significantly increase retention scores (Davis et al., 2022). Further support comes from Mayer and DaPra (2012), who found that learners performed better on a transfer test when a human-voiced agent displayed human-like gestures, facial expression, eye gaze, and body movement than when the agent did not, yielding an embodiment effect. The participants in the study by Fiorini et al. (2024) reported greater arousal and dominance when interacting with embodied robots compared to voice-only interfaces. Perhaps in the same vain, the study by Dennler et al. (2024) suggests that embodiment increases perceived capability, which may affect information retention. These findings therefore indicate that higher expectations may lead to increased engagement but also potential disappointment if unmet. More broadly speaking, in their meta-analysis, Ouyang and Xu (2024) argue that instead of using robots to directly convey knowledge, instructors should utilize educational robotics to facilitate students’ learning experience and work as facilitators to provide guidance and support students.
Social Cues in Multimedia and Human-Robot Interaction
Mayer’s (2014) research also suggests that social cues like conversational tone and embodiment enhance learning, aligning with Admoni & Scassellati’s (2017) findings that gaze cues improve engagement and trust in robots. This could imply that AI voices in robots might be more effective if combined with gaze behavior and facial expressions, as suggested by Schömbs et al. (2023).
Body movements and tone of voice:
Velentza et al. (2021) found that robots with a cheerful personality and expressive body movements are more engaging and desirable for educational interactions. They also caution that overly friendly storytelling can reduce engagement, as it may come off as unnatural or excessive. Additionally, embodied robots using naturalistic gestures lead to higher perceived emotional engagement (Fiorini et al., 2024). These findings highlight the importance of synchronized verbal and non-verbal cues in improving communication effectiveness. Furthermore, users tend to expect more human-like behavior from robots with a physical body compared to virtual ones (Dennler et al., 2024). In regards to pitch, Suzuki et al. (2003) found that humans are sensitive to even the slightest changes in synthetic voice pitch and that they can view these changes as either confirmation or negation, which can be an important factor for problem solving and a consideration for effective learning environments. Still, it is important that the voice isn't too cute, as that can hinder learning outcomes (Jing et al., 2024).
What we know about human preferences for robot voices:
Masculine voice agents are perceived as more "informative" (Seaborn et al., 2021), and social presence is rated higher when a robot’s perceived gender matches its voice (Seaborn et al., 2021). This is important because higher perceived social presence is associated with improved learning outcomes (Craig & Schroeder, 2017). Additionally, both feminine and masculine voices are considered appropriate for educational settings (Seaborn et al., 2021). More specifically, the Nao robot with a masculine voice was perceived as friendlier, more trustworthy, and that the masculine voice was a better overall fit for it (Seaborn et al., 2021). Additionally, the use of vocal fillers tends to enhance user experiences with voice agents. When robots utilized hedges and discourse markers, such as vocal fillers, people responded to them similarly to how they would respond to humans (Seaborn et al., 2021).
The full list of the 25 studies can be found here