PRE2022 3 Group1: Difference between revisions
(→Image recognition/sensors: Wrote on sensors) |
|||
(198 intermediate revisions by 6 users not shown) | |||
Line 23: | Line 23: | ||
|- | |- | ||
|Adrian Kondanari | |Adrian Kondanari | ||
| | |1624539 | ||
| | |BW | ||
|- | |- | ||
|Aron van Cauter | |Aron van Cauter | ||
Line 33: | Line 33: | ||
<br /> | <br /> | ||
== | ==Abstract== | ||
In this wiki our group examined the issue of man overboard (MOB) incidents and proposes a solution to increase the survival chances of victims. A state-of-the-art literature review was conducted, which explored various aspects of MOB incidents such as search area, search patterns, oceanic currents and temperatures, and current person in water detection technology such as drones. A proof of concept was then developed using an XY-rig, which incorporated a thermal camera connected to a Raspberry Pi. The results showed that the thermal camera was able to successfully locate a heat element in a bland environment, representing the ocean. In future work, the solution will be extended to a drone swarm, where multiple drones can be deployed to search for a victim. This solution has the potential to improve the effectiveness of search and rescue operations, and ultimately increase the survival rate of MOB victims. | |||
== | ==Introduction== | ||
Working on board a cargo ship is a dangerous occupation, with man overboard (MOB) incidents being a particularly serious concern. 91 fatalities were reported as a result of MOB events aboard cargo ships between 2014 and 2021<ref name=":9">ANNUAL OVERVIEW OF MARINE CASUALTIES AND INCIDENTS 2022. (2022). In ''EMSA'' (Ares(2022)8241169). European Maritime Safety Agency. from <nowiki>https://www.emsa.europa.eu/newsroom/latest-news/download/7362/4867/23.html</nowiki></ref>. Numerous factors, including; bad weather, unstable loads, and a lack of adequate safety equipment, are the main cause of such accidents. Unfortunately, locating a MOB victim in the ocean can be a challenging and complicated task. The search procedure can be affected by all sorts of elements such as the climate, ocean currents, and visibility. | |||
= | To better understand the scope of the issue, a 2020 study analyzed 100 MOB incidents using 114 parameters to generate a MOB event profile<ref name=":0">Gonel, O., & Çiçek, I. (2020, 25 december). ''STATISTICAL ANALYSIS OF MAN OVER BOARD (MOB) INCIDENTS''. ResearchGate. <nowiki>https://www.researchgate.net/publication/348266442_STATISTICAL_ANALYSIS_OF_MAN_OVER_BOARD_MOB_INCIDENTS</nowiki></ref>. 53 of the 100 events that were looked at occurred on cargo ships. In 88 cases, the casualty was deceased as a result of the MOB incident, from those 88 cases 34 were assumed dead and 54 were witnessed dead. From the witness deaths 18 died before the rescue and 31 after the rescue, in 5 cases it is unknown. The cause of death was indicated for 42 cases, the most common cause is identified as drowning (26), followed by trauma (9), cardiac arrest (4) and hypothermia (3). | ||
The | |||
Despite the challenges involved in locating MOB victims in the ocean, we believe that technology can play a crucial role in increasing the survival chances of those involved in such incidents. With this in mind, we aim to develop a device that can improve the efficiency of locating MOB victims on cargo ships. Specifically, we will focus on MOB incidents that occur on cargo ships, as the 2020 study indicated that over half of such incidents occur on these vessels. The end users of our product are personnel of cargo ships, shipping companies that own a fleet of cargo ships, ports, and possibly the military. | |||
It's worth noting that searching for a victim in the ocean can be a challenging and complex task, we therefore make several assumptions. First, we presume that both at the time of the accident and during the search and rescue effort, the weather conditions are mild. This means mild waves, a mild breeze, little rain, and average sea and air temperatures. We also assume that it is night-time at the time of the incident and that there is only one victim who is capable of maintaining afloat and does not have any major injuries. Additionally, we assume that the time of the incident is known, allowing for a general understanding of the search area. | |||
To develop our device, we will conduct a state-of-the-art literature study to investigate various factors that can impact the search process. This will include examining search areas, search patterns, oceanic currents and temperatures, and current person-in-water detection technology. | |||
We hope to increase the efficiency of the search process and further improve the survival chances of those involved in MOB incidents on big (cargo) ships. By developing this device, we believe that we can make a meaningful contribution to the safety of those who work at sea. | |||
==State-of-the-art literature study== | |||
Before we can get started with the project it is important to gather knowledge about the state-of-the-art (SotA) concerning safety measures and pre-existing technology that aids victims of a MOB incident. We therefore performed a SotA literature study. | |||
===Current search methods=== | |||
Search procedures for when a man goes overboard already exist. In the IAMSAR manual some of these procedures are explained. In this paragraph we discuss and analyze these procedures and go over some different search patterns used. | |||
====Current Procedures<ref>https://www.marineinsight.com/marine-safety/man-overboard-situation-on-ship-and-ways-to-tackle-it/#:~:text=1</ref>==== | |||
The existing procedures for people overboard on big cargo ships are quite elaborate. The procedure basically consists of a few key steps: it all starts when a victim goes overboard, from there the following will happen: | |||
Step 1: Notify staff on board | |||
Step 2: Mark the position the victim went overboard / release a marker | |||
Step 3: Turn the ship around | |||
=== | Step 4: Final search (finding the marker and victim) | ||
Step 5: Rescue | |||
At first this process seems quite efficient, but what should be taken into account is that Step 3, can take a long time for big ships<ref>https://content.iospress.com/download/international-shipbuilding-progress/isp23-260-02?id=international-shipbuilding-progress%2Fisp23-260-02<br /></ref> . For some ships this can take over 20 minutes while traveling a few kilometers. Then there are also some factors going into the step 4: For different environments and conditions different patterns are recommended. Some of these will be explained below.<ref>https://owaysonline.com/iamsar-search-patterns/</ref> | |||
=====Expanding Square Search===== | |||
The expanding square search is a method that can be used to systematically inspect a body of water for signs of an MOB victim. The search should start at the last known location of the victim, from that point the search starts and expands outwards with course alterations of 90°, as can be seen in the image provided. The course of expansion heavily depends on the specification of the device that will be used. Thermal imaging can be used to scan the area, looking for any signs of the victim such as floating debris or the person themselves. | |||
=====Sector Search===== | |||
Sector search is another technique that can be used to search for a victim in the ocean. The sector search technique involves dividing the search area into sectors or pie-shaped sections and systematically searching each sector. It again starts at the last known location of the victim. From there the search area is divided and each sector is carefully searched in a clockwise fashion. Sector search can be effective in searching large areas, especially in cases where the search area is relatively circular or symmetric. However, sector search may not be the most efficient or effective method in all situations, especially if the search area is irregular or if weather and ocean conditions make it difficult to maintain a consistent search pattern. | |||
=====Sweep search===== | |||
The sweep search pattern is another technique that can be used to search for a victim in the ocean. It involves moving the search vessel or search device in a back-and-forth sweeping motion across the search area.[[File:Expanding Square.png|thumb|Expanding Sqaure, IAMSAR search patterns<ref name=":4">O. (2021, 5 april). ''IAMSAR Search Patterns Explained with Sketches - Oways Online''. Oways Online. <nowiki>https://owaysonline.com/iamsar-search-patterns/</nowiki></ref>|left]] | |||
[[File:Sweep Search.png|thumb|Sweep Search, IAMSAR search patterns<ref name=":4" />]] | |||
[[File:Sector Search.png|center|thumb|Sector Search, IAMSAR search pattern<ref>O. (2021, 5 april). ''IAMSAR Search Patterns Explained with Sketches - Oways Online''. Oways Online. <nowiki>https://owaysonline.com/iamsar-search-patterns/</nowiki></ref>]] | |||
When the location of the MOB victim is known well, the Expanding Square or Sector Search is recommended. If the location of the accident is not accurately known different patterns are recommended such as Sweep Search. IAMSAR also mentions other factors that need to be taken into account during the search. If a person falls into the water they will for example be moved away by currents. | |||
===Search area=== | |||
A person floating in the ocean can drift up to 10 nautical miles per day, this is equal to 0.2 meters per second. If the current is unknown this will mean the search area would expand in a circle if the starting location would be known. If we define the search area as a circle with a radius ''r'', the radius of the circle will depend on time, we describe the search area with the following formula: ''r = 0.2 t''. Here ''t'' is the time since the person fell overboard. The following table shows the search area size compared to time. | |||
{| class="wikitable" | {| class="wikitable" | ||
| | |Time overboard (minutes) | ||
| | |Search area size (m²) | ||
|- | |- | ||
| | |1 | ||
| | |452 | ||
| | |- | ||
| | |5 | ||
| | |11310 | ||
| | |- | ||
| | |15 | ||
| | |101788 | ||
| | |} | ||
The table clearly shows the search problem can become quite big. Especially when the search takes a long time. This shows the importance of finding the victim quickly.<br />[[File:Shipping routes.png|thumb|Image showing the world's shipping routes on the Atlantic ocean<ref name=":3">[https://www.vox.com/2016/4/25/11503152/shipping-routes-map "Shipping routes are changing with the climate" by Brad Plumer, published on Vox on April 25, 2016 (https://www.vox.com/2016/4/25/11503152/shipping-routes-map).]</ref>]] | |||
===Influence of cold water and oceanic temperatures=== | |||
Having a good understanding on how factors such as water temperature influence the rescue mission is crucial in ensuring that the rescue mission is successful. Depending on the water temperature, the time frame of the rescue mission changes and different measures need to be taken into account. Ideally the victim should be rescued as soon as possible, but having a known time frame of within which the mission needs to be executed gives the crew members a good idea on how to approach the situation. | |||
Since we're dealing with people who have fallen in water, we need to consider their survival time. When being submerged in water, your greatest risk is developing hypothermia as your body cools down 25 times faster in water than in air of the same temperature. The effects of hypothermia go from confusion and exhaustion, to unconsciousness and coma, and in the end lead to death. It is thus of utmost importance to detect and rescue a person in the water before the severe effects of hypothermia set in. The following table shows the relation between the temperature of the water and the time frame of the effects of hypothermia. The time windows are wide as the time when the effects of hypothermia begin depend on several factors, like age and percentage of body fat.<ref name=":1">[https://aceboater.com/en-us/cold-water-immersion-and-hypothermia "Cold Water Immersion and Hypothermia" by Aceboater, published on their website on an unknown date (https://aceboater.com/en-us/cold-water-immersion-and-hypothermia).]</ref> | |||
{| class="wikitable" | |||
|+Relation between water temperature and hypothermia<ref name=":1" /> | |||
!Water temperature (C°) | |||
!Time until unconsciousness | |||
!Survival time | |||
|- | |||
|0 | |||
|<15 min | |||
|15 - 45 min | |||
|- | |||
|0 - 5 | |||
|15 - 30 min | |||
|30 - 90 min | |||
|- | |||
|5 - 10 | |||
|30 - 60 min | |||
|1 - 3 hours | |||
|- | |||
|10 - 15 | |||
|1 - 2 hours | |||
|1 - 6 hours | |||
|- | |||
|15 - 20 | |||
|2 - 7 hours | |||
|2 - 40 hours | |||
|- | |||
|20 - 25 | |||
|3 - 12 hours | |||
|3 - indefinite | |||
|}[[File:Water temp.png|thumb|Image showing oceanic temperatures on 22 March 2023<ref name=":2">[https://www.seatemperature.org/ "SeaTemperature.org - Sea Surface Temperature (SST) map for the world's oceans and seas" by SeaTemperature.org, accessed on April 9, 2023 (https://www.seatemperature.org/).]</ref>]]Next, oceanic temperatures need to be considered. The two figures on the right show the world's shipping routes on the Atlantic ocean, and oceanic temperatures respectively. From this we can deduce that there are busy shipping routes in the colder regions of the Atlantic ocean, ranging from 3 to 7 degrees Celsius, and also the occasional ship in water at 1 degrees Celsius. The temperatures were taken in late March so it might even be colder in the middle of winter. Since these ships will benefit most from a technology that might result in faster rescue times, it can be concluded at the most desirable performance of the system is to find a person in the water in less than 10 minutes, leaving enough time for the rescue operation to start.<ref name=":2" /><ref name=":3" /> | |||
<br /> | |||
===Possible detection methods=== | |||
There already exists technology that can detected a person in a body of water. Radars are frequently used to detect the distance and velocity of an object by sending electromagnetic waves out and detecting the echo that results from objects. In water environments, a radome is needed to protect the radar but let the electromagnetic waves through. However, even with a radome the signal strength already falls to a third in a wet environment compared to dry. Multiple radar technologies exist with each their different advantages and disadvantages. Pulse Coherent Radar (PCR) can pulse the transmitting signal so it only uses 1% of the energy. Another aspect of this radar is coherence. This means that the signal has a consistent time and phase so the measurements can be incredibly precise. It can also separate the amplitude, time and phase of the received signal to identify different materials. This is not necessarily needed in our use case. The 'Sparse' service is the best service for detecting objects since it samples waves every 6 cm. You don't want millimeter precise measurements in a rough environment like the ocean so these robust measurements are ideal.<ref>Frotan, D. F., & Moths, J. M. (2022). ''Human Body Presence Detection in Water Environments Using Pulse Coherent Radar'' [Bachelor Thesis]. Malmo University.</ref> | |||
Another detection method that does not yet exist is to send out a swarm of drones to search for victim. The research paper "Intelligent Drone Swarm for Search and Rescue Operations at Sea"<ref>Lomonaco, V., Trotta, A., Ziosi, M., De Dios Yáñez Ávila, J., & Díaz-Rodríguez, N. (2018b). Intelligent Drone Swarm for Search and Rescue Operations at Sea. ''HAL (Le Centre pour la Communication Scientifique Directe)''.</ref> presents a novel approach for using a swarm of autonomous drones to assist in search and rescue operations at sea. The authors propose a system that uses machine learning algorithms and computer vision techniques to enable the drones to detect and classify objects in real-time, such as a person in distress or a life raft. The paper describes the hardware and software architecture of the drone swarm system, which consists of a ground station, multiple drones equipped with cameras, and a cloud-based server for data processing and communication. The drones are programmed to fly in a coordinated manner and share information with each other to optimize the search process and ensure efficient coverage of the search area. The authors evaluate the performance of their system in various simulated scenarios, including detection of a drifting boat, a person in the water, and a life raft. The results show that the drone swarm system is capable of detecting and identifying objects accurately and efficiently, with a success rate of over 90%. The research paper presents a promising solution for improving search and rescue operations at sea, leveraging the capabilities of autonomous drones and artificial intelligence. For larger scale operations, drone swarm can be used to cover greater areas for search and rescue. This method is useful when other factors such as the wind, current etc. make finding the victim more difficult. The drone swarm would be implemented in such a way so that areas around the ship, or any search area for that matter, can be searched in a shorter time span, increasing the chances of finding the victim and increasing the chances of survival. When multiple drones are deployed, they all take up a specific search pattern in unison. Depending on the situation different search patterns will be used. If a man overboard is detected rather quickly, one search pattern may be implemented in order to optimize the time it takes in order to locate the victim. When other factors influence the case, such as wind or current, different search patterns will be used in order to adapt to the situation. | |||
There are multiple papers that discuss drone swarms as a method of detecting people on the ocean. The research paper "AutoSOS: Towards Multi-UAV Systems Supporting Maritime Search and Rescue with Lightweight AI and Edge Computing"<ref>Queralta, J. P., Raitoharju, J., Gia, T. N., Passalis, N., & Westerlund, T. (2020). AutoSOS: Towards Multi-UAV Systems Supporting Maritime Search and Rescue with Lightweight AI and Edge Computing. ''arXiv (Cornell University)''. <nowiki>https://arxiv.org/pdf/2005.03409.pdf</nowiki></ref> proposes a similar approach for using multiple unmanned aerial vehicles (UAVs) equipped with lightweight AI and edge computing to support maritime search and rescue (SAR) operations. The authors describe the AutoSOS system, which consists of a ground station, multiple UAVs, and a cloud-based platform for data processing and communication. The UAVs are equipped with cameras and other sensors to detect and classify objects in real-time, such as a person in the water or a life raft. The lightweight AI algorithms are designed to operate on the UAVs, minimizing the need for high-bandwidth communication with the cloud-based platform. The paper highlights the advantages of using a multi-UAV system for SAR operations, such as increased coverage area, improved situational awareness, and faster response times. The authors also discuss the technical challenges of designing and implementing the AutoSOS system, such as optimizing the UAV flight paths and ensuring the reliability of the communication and data processing systems. To demonstrate the effectiveness of the AutoSOS system, the authors present a case study of a simulated SAR mission in a coastal area. The results show that the system is capable of detecting and identifying objects accurately and efficiently, with a success rate of over 90%. | |||
Another paper that is of relevance is the "A Review on Marine Search and Rescue Operations Using Unmanned Aerial Vehicles"<ref>Yeong, S. P., King, L. M., & Dol, S. S. (2015). A Review on Marine Search and Rescue Operations Using Unmanned Aerial Vehicles. ''Zenodo (CERN European Organization for Nuclear Research)''. <nowiki>https://doi.org/10.5281/zenodo.1107672</nowiki></ref>. It provides a comprehensive review of the current state of research on using unmanned aerial vehicles (UAVs) for search and rescue (SAR) operations in marine environments. The paper discusses the main challenges of conducting SAR operations in marine environments, such as limited visibility, harsh weather conditions, and the vast and complex nature of the search areas. The authors then review the different types of UAVs and their applications in SAR operations, such as fixed-wing UAVs for long-range surveillance and multirotor UAVs for close-range inspection and search missions. The paper highlights the advantages of using UAVs for SAR operations, such as increased coverage area, improved situational awareness, and reduced risk to human rescuers. The authors also discuss the technical challenges of designing and operating UAVs in marine environments, such as optimizing the flight paths, ensuring reliable communication and data transmission, and complying with regulatory and ethical guidelines. To demonstrate the effectiveness of UAVs for SAR operations, the authors review several case studies of real-world applications, such as detecting and rescuing distressed vessels and locating missing persons in coastal areas. The results show that UAVs can provide valuable support to SAR operations, improving response times, and increasing the chances of successful rescue. | |||
Another paper, "Requirements and Limitations of Thermal Drones for Effective Search and Rescue in Marine and Coastal Areas"<ref>Burke, C., McWhirter, P. R., Veitch-Michaelis, J., McAree, O., Pointon, H. A. G., Wich, S. A., & Longmore, S. N. (2019). Requirements and Limitations of Thermal Drones for Effective Search and Rescue in Marine and Coastal Areas. ''Drones'', ''3''(4), 78. <nowiki>https://doi.org/10.3390/drones3040078</nowiki></ref>, explores the potential of thermal drones for search and rescue (SAR) operations in marine and coastal areas. The authors review the main features and capabilities of thermal drones, including their ability to detect heat signatures and identify objects in low light or night conditions. The paper highlights the key requirements and limitations of using thermal drones for SAR operations, such as the need for high-resolution thermal cameras, long flight time, and reliable communication systems. The authors also discuss the challenges of operating drones in harsh weather conditions and the importance of complying with regulatory and ethical guidelines. To demonstrate the effectiveness of thermal drones for SAR operations, the authors present a case study of a simulated rescue mission in a coastal area. The results show that thermal drones can provide valuable assistance in locating and identifying missing persons or distressed vessels, particularly in remote or inaccessible areas. The research paper provides a comprehensive overview of the potential of thermal drones for SAR operations in marine and coastal areas, while also highlighting the technical and operational challenges that need to be addressed to ensure their effective use. | |||
From these studies we conclude that the most efficient detection method is a drone swarm with thermal cameras attached to the drones. | |||
====Sensors==== | |||
The main sensor that will be used to locate a victim is a thermal imaging camera (TIC). Since the general search area is small relative to the complete ocean, temperatures of the water will all be relatively the same. The victim will have a much higher temperature than their surroundings, making it easy to detect them. There are a couple important requirements for the TIC. The camera needs a sufficiently high resolution so that we can clearly distinguish the victim from other warm object (think of the marine life). It also needs to have a high refresh rate. Our goal is to detect and locate a victim as soon as possible so that the survival chance is the highest, we therefore need to 'scan' the search area fast and that requires a sufficient refresh rate. For the same reason the thermal camera is required to have a large field of view (FOV). It also needs the right temperature sensitivity. | |||
Other sensors and actuators that we might want to include are a microphone and a loudspeaker. This would allow us to make contact with the victim. However, the issue with a microphone is that the sound of the ocean is most likely so loud that it is unlikely to understand the victim, trying to conversate with the victim might also fatigue them. A loudspeaker can be used to notify the victim rescue is on the way and help them calm down. | |||
====Thermal image recognition==== | |||
For the image recognition we can make use of TensorFlow, Google's Neural Network library. We can, as mentioned before, also choose to not do advanced image detection and instead as soon as a heat source that could reasonably be the victim is detected, send the camera images to the ship where a human operator can verify whether the heat source is actually the victim. | |||
Another option that could be looked into more would be using a hybrid of these two strategies. Namely, using the fast simple heat source detection until a heat source is found and then activating the more advanced AI image detection to verify if it is a human. This would then solve a part of the efficiency problem and would eliminate some need for human operators and higher data transfer requirements. | |||
===Communication Systems=== | |||
In a scenario where a drone needs to send data, such as thermal images, to a container ship on the open sea, there are several reliable methods of communication that can be used, including: | |||
#Satellite Communication: One of the most reliable methods of communication in remote areas, such as the open sea, is through satellite communication. The drone can use a satellite modem to transmit the data to a satellite in orbit, which then relays the data to a ground station or directly to the container ship. Satellite communication can support high data rates and can be used to transmit data over long distances. | |||
#Long-Range Wi-Fi: Another option for communication between the drone and the container ship is through long-range Wi-Fi. The drone can be equipped with a Wi-Fi transmitter that is capable of transmitting data over a long distance, and the container ship can be equipped with a Wi-Fi receiver that is capable of receiving the data. Long-range Wi-Fi can support high data rates and can be used to transmit data over several kilometers, depending on the power of the transmitter and receiver. | |||
#Cellular Communication: Depending on the location of the container ship and the availability of cellular coverage, the drone may be able to use cellular communication to transmit data to the ship. The drone can be equipped with a cellular modem that is capable of connecting to a cellular network, and the container ship can be equipped with a cellular receiver that is capable of receiving the data. Cellular communication can support moderate data rates and can be used to transmit data over several kilometers, depending on the strength of the cellular signal. | |||
#Radio Communication: Another option for communication between the drone and the container ship is through radio communication. The drone can be equipped with a radio transmitter that is capable of transmitting data over a long distance, and the container ship can be equipped with a radio receiver that is capable of receiving the data. Radio communication can support low to moderate data rates and can be used to transmit data over several kilometers, depending on the power of the transmitter and receiver. | |||
Overall, the choice of communication method will depend on the specific requirements of the scenario, such as the distance between the drone and the container ship, the data rate requirements, and the availability of communication infrastructure. Satellite communication is typically the most reliable and versatile option in remote areas, but it may also be the most expensive.<ref>Long-distance Video Streaming and Telemetry via Raw WiFi Radio<nowiki/>https://dev.px4.io/v1.11_noredirect/en/qgc/video_streaming_wifi_broadcast.html</ref> | |||
==Design== | |||
===Requirements=== | |||
{| class="wikitable" | |||
|+MoSCoW method | |||
!Must | |||
!Should | |||
!Could | |||
!Won't | |||
|- | |||
|Have sensors to detect a person within 10 minutes | |||
|Have life assist systems | |||
|Have communication between victim and ship | |||
|Have the ability to take the person to safety | |||
|- | |- | ||
| | |Have a communication system to communicate with the involved ship | ||
| | |Resist (bad) weather to a degree | ||
| | | | ||
| | | | ||
|- | |- | ||
| | |Be able to withstand an air temperature range of -3'''°'''C to 36'''°'''C'''.''' | ||
|A person recognition algorithm | |||
| | |||
| | | | ||
| | | | ||
|- | |- | ||
| | |Be able to operate in the dark | ||
| | | | ||
| | | | ||
| | | | ||
|- | |- | ||
| | |Be able to operate in the rain | ||
| | | | ||
| | | | ||
| | | | ||
|- | |- | ||
| | |Be able to withstand wind forces of 4 beaufort. | ||
| | | | ||
| | | | ||
| | | | ||
|} | |} | ||
====Drone requirements==== | |||
A couple of requirements need to be met in order for the drone to be functioning as intended. In this section we will discuss the possibilities of remote drone control and how it ties into our case. Different communication methods will be discussed and we have to choose the best one for our scenario. And the detection method using thermal imaging will also be discussed. This is also tied with the drone search speed, which also influences our approach. | |||
== | =====Remote Drone Control===== | ||
A lot of research has already gone into autonomous drone flight. Take a look at autonomous drone racing for example<ref>AlphaPilot: autonomous drone racing, Philipp Foehn, Dario Brescianini, Elia Kaufmann, Titus Cieslewski, Mathias Gehrig, Manasi Muglikar & Davide Scaramuzza | |||
https://link.springer.com/article/10.1007/s10514-021-10011-y</ref> . A lot of consumer drones also are capable of autonomous flight, for example DJI has a waypoint system based on GPS coordinates and their drones are also capable of tracking a moving person/object. DJI's waypoint system works by loading a set of GPS coordinates onto the drone, the drone will navigate itself to the first set coordinates. From there it goes on to the next. Until it has reached the final set of coordinates. The moving object tracking is a bit more complicated. This involves image recognition.<ref>Autonomous Waypoint-based Guidance Methods for Small Size Unmanned Aerial Vehicles, Dániel Stojcsics | |||
http://acta.uni-obuda.hu/Stojcsics_56.pdf</ref> | |||
< | However, the firmware on most of these commercial drones is not open to being interfaced with. This makes is much more difficult to make a more autonomous drone without building one from scratch. Another point is that drones, and especially the autonomous kind, are quite expensive. And due to the aforementioned lack of interfacing options with the flight controllers of commercial drones it is also not really possible to take a cheaper non-autonomous drone and hook it up to a computer that will act as a module to make the drone fly autonomously. | ||
=====Drone search speed===== | |||
[[File:Drone search speed.png|thumb|X(axis) = time in seconds, Y(axis) = area, the blue line is area to be searched and the yellow line is area searched. ]] | |||
The drone search speed will be limited by multiple things such as flight speed, sensor resolution and quality/speed of the detection algorithm. Since we still have some unknowns we would like to make some assumptions. Lets assume the algorithm is able to detect a human if they take up at least a space of 25 by 25 pixels. Lets also assume a human at sea takes up at least 25 by 25 cm. A good sensor to use for the detection of humans at sea in the night would be a heat camera. These cameras take images in the IR spectrum and thus aren't hindered by low light conditions. They have a field of view (FOV), a resolution and a refresh rate. The height the drone can fly will be determined by the FOV, and resolution. A typical FOV for Raspberry Pi cameras is about 60 degrees of horizontal<ref>https://www.raspberrypi.com/documentation/accessories/camera.htm</ref> and a typical resolution would be 1080*1920 pixels. Here 1920 is the horizontal direction. If the drone would have a sensor of these specs it would be able to fly about 15 meters high, here it would be able to scan a line of 19.2 meters wide. Lets assume the algorithm needs to have the human in shot for 5 seconds for it to be detected by the algorithm.<ref>https://www.researchgate.net/publication/350981551_Real-Time_Human_Detection_in_Thermal_Infrared_Images</ref> The vertical FOV of Raspberry Pi sensors is about 40 degrees, this would result in a distance of about 10 meters vertical to be seen by the drone. This means it can fly at about 2 m/s. The area the drone can covers is thus 20m*2m/s*''t'' where ''t'' is the time since dispatched. Another solution to the detection could be to not look for humans specifically, but for relatively hot areas in the image. This might be an option since out at sea there is a significant heat difference between a human with a body temprature of approximately 37 degrees celcius and the environment that consists mostly of cold water. When using this strategy it is however necessary to include some kind of verification in the system so that the drone will not signal that the victim is found when it, for example, finds a maritime animal close to the surface of the water. | |||
However, for our estimation of the search speed we will still use the option where an algorithm is used that actually detects a human since this might still be necessary and we prefer to work from the worst case scenario with regards to bounding the minimum required efficiency. In the graph on the right we see that after about 5 minutes the drone is not able to keep up with the search area anymore (even with a perfect search pattern). If we start taking dispatch time into account it would mean these 5 minutes would be about result in about 2.5 of effective search time) this would move the yellow line to the left, this would also mean that one drone is not quick enough. and we would likely need to use multiple drones in order to search fast enough.. | |||
===Components=== | |||
Sensor | |||
*Thermal imaging camera | |||
Communication Equipment | |||
*Wireless communication module | |||
Drone with autonomous/waypoint flight capabilities | |||
Small computer capable of: | |||
* | *Multithreading/multiprocessing | ||
*Running TensorFlow or other image recognition AI software | |||
*Interfacing with electronics (reading the camera image and possibly interfacing with servo motors) | |||
*Running autonomous flight control software | |||
====Drone==== | |||
For our real design we would like to use a drone that is able to fly accurately in mild wind and is able to carry some weight. Since the drone has to fly autonomously it will most likely be necessary to make a custom drone for this project since almost all commercially available drones don't allow interfacing with the flight controller in such a way that an external device/computer can control the drone. We have not really researched into how to make a drone from scratch since this is not really in the scope of the project, however for the autonomous flight a pixhawk flight controller can be used. The pixhawk is currently the most popular option for autonomous flight as far as we can tell. The pixhawk can then be interfaced with with a Raspberry Pi to controll the autonomous movement of the drone. | |||
We have also not researched into the details of the control of autonomous drones since we already found out pretty early that using an actual drone like this for the proof of concept would not be feasable. The details of the control software would not really contribute to the rest of the design so for our demo we assumed it was possible to transform carthesian waypoints to flight instructions for the drone. | |||
=== | ====Computer==== | ||
We have decided to use a Raspberry Pi as our computer due to it's computing power to size/weight ratio. The Raspberry Pi can do all the things we wish our computer to do so it is a great choice. | |||
=== | ===Search pattern=== | ||
[[File:Expanding square search.jpg|thumb|Expanding Square Search]] | |||
[[File:Adapted square search.jpg|thumb|Adapted Square Search]] | |||
[[File:Cone.jpg|thumb|Cone Search]] | |||
[[File:Two drone cone.jpg|thumb|Multi drone Cone Search]] | |||
[[File:Wait and sweep no target.jpg|thumb|Wait And Sweep Search]] | |||
A critical factor that can determine the effectiveness of a search operation and its ability to meet our requirements is the search pattern employed. We have identified three potential search patterns that could be utilized, and we plan to test each of these methods using a model to evaluate their effectiveness. The selection of an appropriate search pattern is crucial as it can have a significant impact on the chances of success in locating the person who has fallen overboard. By testing each of the proposed search patterns using a model, we can determine which method is best suited for the specific circumstances of the search and optimize our search efforts accordingly. | |||
One already existing search pattern is the expanding square search. This involves the drone moving outward from the last known location of the person in a square pattern, with each pass covering an increasing amount of area. This method is efficient and can cover a lot of ground quickly, but it does not take into account the direction of the current, so could in theory only be efficiently used in situations where the victim doesn’t drift. | |||
To address this issue, an improvement on the expanding square search is to incorporate the direction of the current. We call this Adapted Square Search. This involves adding a few repetitions in the direction of the current to increase the chances of finding the missing person. The amount of repetitions is determined by the strength of the current; No current would mean no repetitions (regular expanding square), a weak current one extra repetition in that direction, a bit stronger two repetitions, etc. This method can be especially useful when there is a strong current that could have carried the person further away from their original location. | |||
Another possible search pattern is the cone pattern. This involves the drone moving outward from a small distance before the last known location of the person in a cone-shaped pattern, with the width of the cone expanding in the direction of the current. This method could be the most efficient as it doesn’t return back to the initial position and doesn’t search against the current. | |||
Then there is also a different approach, since the current moves the victim will move, we can also wait for it to come to us. This method is called wait and sweep, here the drone does a sweep search pattern, but it sweep the same area over and over. | |||
== | ===Programming=== | ||
=== | All of the code used in this project is uploaded on the following GitHub repository: https://github.com/ThijsEgberts/ProjectRobotsEverywhere. | ||
===Proof of concept=== | |||
In order to prove the concept not all Moscow requirements need to be fulfilled. Since the most important part of our system is the detection, this needs to be shown working. The algorithms for both search and detection need to be proven and the hardware also needs to be shown functioning. However weather resistibility, does not have to be taken into account yet. The test also does not have to be done at full scale. | |||
====Designing and building==== | |||
Materials: | |||
*Plastic container 40cm x 60cm | |||
*2 Metal rods 8 mm x 100cm | |||
*Linear ball bearing | |||
*ABS (to print pieces) | |||
[[File:Slider.png|thumb|3D model of slider]] | |||
The design process of the prototype was done with Fusion 360. This program allows for detailed 3D-modeling and easy conversion to Prusaslicer which is the slicer program. After that the pieces that were designed are ready to be printed. The 3D-printer that was used is the Voron V0. We made a total of 4 corner attachment pieces, 2 sliders and 1 slider platform. The whole process required a lot of little design changes to properly fit and assemble the entire setup. Eventually the final assembly process was easily done with some screws and bolts. The search pattern was drawn on the bottom of the container to help the people moving the camera with following the movement instructions and make it more clear for the demonstration video what was going on.[[File:Corner piece.png|thumb|3D model of corner attachment piece|none]][[File:Hele setup.png|thumb|3D-model of entire setup|center]] | |||
[[File:Platform.png|thumb|3D model of slider platform|left]] | |||
<br /><br /><br /> | |||
<br /> | |||
=== | <br /> | ||
To | ====Demo==== | ||
A Raspberry Pi and a thermal camera were mounted on a rig to demonstrate the proof of concept. Ropes were used to manually modify the camera's position. To make it easier to follow the search path, a map of the search pattern was drawn on paper. A tiny tea light was used as a heat source to imitate the relevant scenario and provide a distinct heat signature. Also, a rope that was tied to the lamp served as an ocean current replica. The heat source was identified by following the search pattern according to instructions being output by the Raspberry Pi. | |||
==== | =====Thermal camera===== | ||
This | For our demo we used the MLX90640 thermal camera. This camera has a resolution of 32x24 pixels and a 55° field of view. The camera itself has a refresh rate of 60fps, however in our application this is limited due to the speed at which the I<sup>2</sup>C interface works. Therefore in our application we could process images for heat sources at about 7fps when not displaying the image and at about 1fps when displaying the image. This speed may in part be due the use of python but python did make the image manipulation and output much easier. | ||
=====Heating element===== | |||
For the experiment we needed to have a detectable heat signature. For this we chose the simplest possible solution, which was just having a candle. This gave us a detectable heat signature that we can work with. | |||
==== | ====Design of the Model==== | ||
In order to investigate how efficient the drone is at finding the victim a model was developed. The workings of the model will be explained here: | |||
The model simulates the victim moving in the water, for a few minutes before the drones arrive at the scene. After this dispatch time, it starts moving the drone(s) simultaneously to the victim. From here it checks if the victim is in view of the drone’s view every time the drone is moved to a new location. If this is the case, the victim is found and the search ends. | |||
Victim movement is simulated using some randomness. The victim is moved every second at a random angle, this angle is taken using a normal distribution (centered at the angle the current is at). The victim is also moved forward every second, this distance is uniformly varied between the a flow rate of 5cm per second below and above the current. The randomness is included into the victim movement in order to have every search be a unique one, and sufficiently test the search algorithms. | |||
Drone movement is a bit different from victim movement, this is totally not random. The drones are moved along a pre-determined search pattern. | |||
The full code of the model can be found on our programming Github | |||
== | <br /> | ||
==Results== | |||
In this section we will talk about our results surrounding tests with our design, namely our physical demo test and our model tests. | |||
====Demo results==== | |||
[[File:Adapted square.jpg|thumb|Model of Adapted Square Search]] | |||
Our main demo results are that it is possible to detect heat sources and follow a search pattern with a Raspberry Pi drone model. For the demo we only executed the adapted expanding square search pattern due to practicalities with our test setup, namely that our test rig was relatively small and rectangular. | |||
==== | ====Model results==== | ||
Different search patterns also influence the speed it takes to find the target. Multiple different patterns were investigated using a model made in Python. Using this model different search patterns were each tested for 10000 trials. A current of about 25 cm/s is used in the positive y direction. The drone has a field of view of 3 m by 2.4 m (this matches the FOV of the camera we used for the demo), and is moved at a max speed of 3m/s. 4 different patterns were investigated. These are Square Search, Adapted Square Search, Wait and Sweep, and a Cone search. The full results can be seen in the Appendix | |||
<br /> | |||
==== | ======Single drone====== | ||
This | Square search is one of the most simple patterns. The patterns simply spiral out, in a square. This pattern is however not very applicable to our case. Since the target moves in (mostly) one direction, and is not standing still. This can also be seen in the simulation results. Of the 10000 trials done, only 1.4 % succeeded. With an average total time of 313 seconds including 4 minutes of dispatch time. | ||
Since the Square search did not seem to work very well, an adapted version of the algorithm was set up, this Adapted Square search puts more focus on the flow direction, by looping back on itself . This pattern saw serious improvement over the ‘normal’ square search. The success rate went up to 22.4% with a total time of 326 seconds including the dispatch time. [[File:Wait and sweep.jpg|thumb|Model of single drone Wait And Sweep]]Cone search is different from the previous two patterns. It only searches in one direction and sweeps with increasing width. For this pattern multiple different cones were used, one with an angle of ¼ pi radians, one with an angle of ½ pi radians, and finally one with an angle of ¾ pi radians. The success rate varied between these, with the highest of 57.7% corresponding to the ½ pi radians cone. The other success rates were 16.9% and 51.3% belonging to the ¼ pi radian and ¾ pi radian cones respectively. The average total time of success for the ½ pi cone was 343 seconds including the dispatch time. | |||
Wait and Sweep is different from the other patterns, this pattern does not focus on covering new areas it instead focuses on searching the same area over and over again, waiting for the victim to drift into the frame. The parameters going into this search are mainly the distance from the spot the victim dropped into the water and the sweep width. Multiple different distances (2.4 m, 7.2 m, 12 m, 16.8 m, and 24 m) were tried with both a 15 m and 30 m sweep width. A distance of 16.8 m with a sweep with of 15 meters resulted in the highest success rate of 70.0% with a success time of 428 seconds including dispatch time. | |||
<br />[[File:Multi drone cone search.jpg|thumb|Model of multi drone Cone Search]]One note here is that both Square search and Adapted Square search are a lot more efficient at lower flow rates. reducing the flow rates from 25 cm/s to 10 cm/s increases the success rates to 49.5% for normal square search and 92.5% for the adapted version. this is a very big improvement. While cone performs similarly and decrease in efficiency is seen for Wait and Sweep. | |||
==== | ======Multi drone====== | ||
Both Square Search algorithms were not tested for multiple drones since the ‘normal’ square search was not very efficient and the adapted version is difficult to scale for multiple drones. The cone and sweep and wait patterns were however tested for multiple drones. | |||
For both the cone and sweep and wait an increase in efficiency was found when implementing more drones. For both we saw more drones equal to a higher efficiency, however, for the sweep and wait the efficiency reaches a limit of about 95% (distance 15m, width 30m) while the cone also slowly gets more efficient, and reaches about the same limit of 95% (cone angle ½ pi radians). The cone has a 260-second average detection time while the sweep and wait takes a bit longer (288 seconds). These include the dispatch time. This means the cone will be a bit better on average. | |||
====Ethical concerns in rescue robotics: a scoping review | ==Ethical analysis== | ||
The paper "Ethical concerns in rescue robotics: a scoping review"<ref>Battistuzzi, L., Recchiuto, C.T. & Sgorbissa, A. Ethical concerns in rescue robotics: a scoping review. ''Ethics Inf Technol'' 23, 863–875 (2021). <nowiki>https://doi.org/10.1007/s10676-021-09603-0</nowiki></ref> describes seven ethical concerns regarding rescue robots. In this chapter, each ethical concern from the paper is summarized and then applied to our case. | |||
===Fairness and discrimination=== | |||
Hazards and benefits should be fairly distributed to avoid the possibility of some subjects incurring only costs while other subjects enjoy only benefits. This condition is particularly critical for search and rescue robot systems, e.g., when a robot makes decisions about prioritizing the order in which the detected victims are reported to the human rescuers or about which detected victim it should try to transport first. For our scenario, when there is a situation where multiple people have fallen over board, discrimination is something to look out for. When searching for people, discrimination can not take place because if the robot has found one person it will continue looking for the others and since it does not take people out of the water it does not need to make decisions about prioritizing people. However, we are also considering dropping lifebuoys and here prioritizing order does come into account. In the situation where the robot can only carry one life vest, we propose that it drops the lifebuoy one the first person it finds, then comes back to the ship to restock, and continues the search. This will be the most efficient and also prevents discrimination between multiple victims. | |||
=== | ===False or excessive expectations=== | ||
Stakeholders generally have difficulties with accurately assessing the capabilities of a rescue robot. May cause them to overestimate and rely too much on the robot and give false hopes for the robot to safe a victim. On the other hand, if a robot is underestimated, it may be underutilized in cases where it could have saved a person. For our scenario, it is important to accurately inform stakeholders about the capabilities and limitations of the rescue robot. | |||
===Labor replacement=== | |||
Robots replacing human rescuers might reduce the performance of human contact, situational awareness and manipulation capabilities. Robots might also interfere with rescuers attempt to provide medical advice and support. In our case it is important to not fully take the human aspect out of the equation, the robot should be equipped with speakers for people on the ship to talk to the person. They can check how he is responding and reassure the victim that they’re coming to help. This will most likely help to calm the person. When the rescue attempt is being made by the crew or other rescuers, the drone should fly away since it’s no longer useful and as to not interfere with the rescue attempt. | |||
=== | ===Privacy=== | ||
The | The use of robots generally leads to an increase in information gathering, which can jeopardize the privacy of personal information. This may be personal information about rescue workers, such as images or data about their physical and mental stress levels, but also about victims or people living or working in the disaster area. What this means for our scenario is that The information gathered by the robots is not shared with anyone outside professional rescue organizations and is exclusively used for rescue purposes. We should also try to limit the gathering of irrelevant data as much as possible. | ||
===Responsibility=== | |||
The use of (autonomous) rescue robots can lead to responsibility ascription issues in the case when for example accidents happen. What this means for us is that we clearly need to state who is (legally) responsible in the case accidents happen during rescue (like operator, manufacturer etc.) Proposal: To ensure trust in our product, we should claim full (legal) responsibility as a manufacturer when accidents happen caused by design flaws or decisions made by the autonomous system. | |||
=== | ===Safety=== | ||
Rescue missions necessarily involve safety risks. Certain of these risks can be mitigated by replacing operators with robots, but robots themselves, in turn, may determine other safety risks, mainly because they can malfunction. Even when they perform correctly, robots can still be harmful: they may, for instance, fail to identify and collide into a human being. In addition, robots can hinder the well-being of victims in subtler ways. For example, the authors argue, being trapped under a collapsed building, wounded and lost, and suddenly being confronted with a robot, especially if there are no humans around, can in itself be a shocking experience. Risks should be contained as much as possible, but we do acknowledge that rescue missions are never completely risk free. | |||
===Trust=== | |||
Trust depends on several factors; reputation and confidence being the most important once. Humans often lack confidence in autonomous robots as they find them unpredictable. What this means for us is that we need to ensure that the robot has a good reputation by informing people about successful rescue attempts and/or tests, and limit unpredictable behavior as much as possible. We can also ensure trust by claiming full (legal) responsibility as a manufacturer when accidents happen caused by design flaws or decisions made by the autonomous system. | |||
<br /> | <br /> | ||
==Conclusion== | |||
For the chosen scenario of search and rescue, the drones prove to be efficient tools in locating and helping victims. Specifically for man overboard situations, where the drone is able to cover large areas. These sorts of technologies prove to be efficient in increasing the rescue chances of such scenarios and can be expanded further than only maritime operations. | |||
As we assumed in the beginning of the wiki that the chosen scenario excludes extreme weather conditions. In our case, mild wind and waves are tolerable as long as these sorts of conditions don't affect the operations of the drone. Certain cargo ship pathways were also chosen such that we avoid extremely cold waters (which are not common paths for ships anyways as seen in the state-of-the-art literature study). | |||
When defining the search area, the current was also taken into account. Assuming that a person can drift up to 0.2 m/s, we calculated that the search area would be around 450 m². As for the search patterns, we can conclude that in the case where we know the exact direction a victim is drifting in, wait and sweep search is the most effective. However for a scenario where the exact drifting direction is less certain, the cone search is probably the best option. For single drone applications with a low drift rate the adapted square search pattern can also be useful. | |||
For the construction of the drone we can conclude that it is a good idea to take a custom drone with a flight controller like the Pixhawk and then control the autonomous flight with Raspberry Pi. The Raspberry Pi will be connected to a thermal camera with a decently good resolution and framerate. The images captured by the camera will then be analyzed with TensorFlow to find victims in the image. When victims are found the ship will be notified of the location over radio communication.<br /> | |||
==Discussion== | |||
During this project there were quite some things that could have been done better. In the beginning of the course the biggest issue was defining the goal of the project and narrowing down the case. The first couple of weeks were spent on deciding on what we would do for this project and defining the specific preferences, requirements and constraints. This left us with a lot less time to go through all of the necessary design phases. The scale of the project was also changing as the weeks were going by. Some things that we wanted to do just weren't feasible in the amount of time that was left. The main example here was that we found out that actually getting an autonomous drone to work turned out to be very hard and expensive given how specific that use case is. The main problem was a combination of us either having to find a very expensive (in the thousands of euros) drone that can be made autonomous or built our own drone from scratch, which would still be pretty expensive and take a lot of effort. | |||
Looking back on the course, the biggest issue that should have been tackled was the planning and making the decision to go for the vision that we had in the beginning. The weekly goal had to be more ambitious as we were left with little time for the project. The vision of the project should have been narrowed down for a specific case like the one that we have right now somewhere around the first week. After this a concrete plan on the whole approach should have been made rather than making up on the spot what needed to be done.<br />It might also have been a good idea to look into the feasibility of making a physical prototype earlier on in the project so that we could make better decisions surrounding this given that in the way it turned out, our physical demo was more a way of showing that the control software and automatic detection is possible instead of also testing our search patterns in real life. | |||
As for our simulations, they are definitely useful, but do not account for more complex movement of the victim or the influence of the weather on the drone. Therefore it might be useful for future research to try to more accurately model oceanic current and the influence of wind and rain on the movement and detection accuracy of the drone. | |||
==Appendix== | ==Appendix== | ||
===Full model results=== | |||
[[File:Square.jpg|thumb|Model of Square Search]] | |||
[[File:Multi drone wait and sweep, flaws.jpg|thumb|Model of multi drone Wait And Sweep]] | |||
[[File:Single drone cone search.jpg|thumb|Model of single drone Cone Search]] | |||
{| class="wikitable" | |||
|Source.Name | |||
|Count | |||
|Mean | |||
|STD | |||
|Median | |||
|Min | |||
|Max | |||
|Succes Ratio | |||
|Succes Speed | |||
|- | |||
|Sweep5_d5_w10.txt | |||
|9006 | |||
|306.4168332 | |||
|66.22582738 | |||
|280 | |||
|240 | |||
|590 | |||
|0.9006 | |||
|66.41683 | |||
|- | |||
|Sweep4_d5_w10.txt | |||
|8840 | |||
|313.3597285 | |||
|69.5844632 | |||
|290 | |||
|240 | |||
|590 | |||
|0.884 | |||
|73.35973 | |||
|- | |||
|Sweep3_d5_w10.txt | |||
|8448 | |||
|321.1316288 | |||
|71.79807856 | |||
|310 | |||
|240 | |||
|590 | |||
|0.8448 | |||
|81.13163 | |||
|- | |||
|cone5_halfpi.txt | |||
|8266 | |||
|261.1154125 | |||
|10.50518206 | |||
|260 | |||
|240 | |||
|310 | |||
|0.8266 | |||
|21.11541 | |||
|- | |||
|Cone_2_halfpi.txt | |||
|7900 | |||
|282.4898734 | |||
|40.08713795 | |||
|270 | |||
|240 | |||
|590 | |||
|0.79 | |||
|42.48987 | |||
|- | |||
|cone_3_halfpi.txt | |||
|7684 | |||
|267.8227486 | |||
|21.28586226 | |||
|260 | |||
|240 | |||
|560 | |||
|0.7684 | |||
|27.82275 | |||
|- | |||
|cone4_halfpi.txt | |||
|7494 | |||
|261.1956232 | |||
|11.1747414 | |||
|260 | |||
|240 | |||
|350 | |||
|0.7494 | |||
|21.19562 | |||
|- | |||
|Sweep2_d5_w10.txt | |||
|7417 | |||
|328.5371444 | |||
|71.95174355 | |||
|310 | |||
|240 | |||
|590 | |||
|0.7417 | |||
|88.53714 | |||
|- | |||
|Cone_2_3quarterpi.txt | |||
|7303 | |||
|316.4042174 | |||
|73.25601744 | |||
|280 | |||
|240 | |||
|570 | |||
|0.7303 | |||
|76.40422 | |||
|- | |||
|Sweep1_d7_w5.txt | |||
|7007 | |||
|428.0034251 | |||
|85.79344366 | |||
|420 | |||
|240 | |||
|590 | |||
|0.7007 | |||
|188.0034 | |||
|- | |||
|Sweep1_d5_w5.txt | |||
|6940 | |||
|335.2247839 | |||
|73.24696142 | |||
|320 | |||
|240 | |||
|590 | |||
|0.694 | |||
|95.22478 | |||
|- | |||
|Cone3_3quarterpi.txt | |||
|6801 | |||
|284.2067343 | |||
|42.58235158 | |||
|270 | |||
|240 | |||
|590 | |||
|0.6801 | |||
|44.20673 | |||
|- | |||
|cone4_3quarterpi.txt | |||
|6644 | |||
|268.385009 | |||
|22.70430898 | |||
|260 | |||
|240 | |||
|580 | |||
|0.6644 | |||
|28.38501 | |||
|- | |||
|cone5_3quarterpi.txt | |||
|6350 | |||
|262.5905512 | |||
|15.23111931 | |||
|260 | |||
|240 | |||
|430 | |||
|0.635 | |||
|22.59055 | |||
|- | |||
|Cone_1_ halfpi.txt | |||
|5777 | |||
|342.7367146 | |||
|83.92058695 | |||
|320 | |||
|240 | |||
|590 | |||
|0.5777 | |||
|102.7367 | |||
|- | |||
|Cone_1_3quarterpi.txt | |||
|5125 | |||
|357.8887805 | |||
|85.33027697 | |||
|330 | |||
|240 | |||
|590 | |||
|0.5125 | |||
|117.8888 | |||
|- | |||
|Sweep1_d7_w10.txt | |||
|4534 | |||
|430.8557565 | |||
|87.55154123 | |||
|440 | |||
|240 | |||
|590 | |||
|0.4534 | |||
|190.8558 | |||
|- | |||
|Sweep1_d5_w10.txt | |||
|3661 | |||
|364.1873805 | |||
|73.35725043 | |||
|340 | |||
|240 | |||
|590 | |||
|0.3661 | |||
|124.1874 | |||
|- | |||
|Sweep1_d3_w5.txt | |||
|2635 | |||
|288.2049336 | |||
|46.36198948 | |||
|280 | |||
|240 | |||
|540 | |||
|0.2635 | |||
|48.20493 | |||
|- | |||
|cone5_quarterpi.txt | |||
|2396 | |||
|258.6978297 | |||
|10.86522538 | |||
|260 | |||
|240 | |||
|300 | |||
|0.2396 | |||
|18.69783 | |||
|- | |||
|cone4_quarterpi.txt | |||
|2242 | |||
|258.8492417 | |||
|11.08224585 | |||
|260 | |||
|240 | |||
|300 | |||
|0.2242 | |||
|18.84924 | |||
|- | |||
|Adapted_SQ.txt | |||
|2236 | |||
|325.8184258 | |||
|71.30174178 | |||
|350 | |||
|240 | |||
|590 | |||
|0.2236 | |||
|85.81843 | |||
|- | |||
|Cone3_quarterpi.txt | |||
|2060 | |||
|257.7669903 | |||
|10.84412396 | |||
|260 | |||
|240 | |||
|290 | |||
|0.206 | |||
|17.76699 | |||
|- | |||
|Cone_1_quarterpi.txt | |||
|1689 | |||
|279.2243931 | |||
|37.61462698 | |||
|270 | |||
|240 | |||
|580 | |||
|0.1689 | |||
|39.22439 | |||
|- | |||
|Cone_2_quarterpi.txt | |||
|1514 | |||
|257.3051519 | |||
|11.50892696 | |||
|260 | |||
|240 | |||
|330 | |||
|0.1514 | |||
|17.30515 | |||
|- | |||
|Sweep1_d3_w10.txt | |||
|1436 | |||
|288.6699164 | |||
|70.27055499 | |||
|240 | |||
|240 | |||
|590 | |||
|0.1436 | |||
|48.66992 | |||
|- | |||
|Sweep1_d10_w5.txt | |||
|968 | |||
|528.7086777 | |||
|40.5658764 | |||
|530 | |||
|340 | |||
|590 | |||
|0.0968 | |||
|288.7087 | |||
|- | |||
|Sweep1_d10_w10.txt | |||
|530 | |||
|533.1320755 | |||
|50.86772116 | |||
|560 | |||
|430 | |||
|590 | |||
|0.053 | |||
|293.1321 | |||
|- | |||
|Sweep1_d1_w5.txt | |||
|194 | |||
|271.4948454 | |||
|45.12885953 | |||
|240 | |||
|240 | |||
|440 | |||
|0.0194 | |||
|31.49485 | |||
|- | |||
|Square.txt | |||
|141 | |||
|313.9007092 | |||
|52.69552773 | |||
|300 | |||
|240 | |||
|460 | |||
|0.0141 | |||
|73.90071 | |||
|} | |||
<br /> | |||
===Logbook=== | ===Logbook=== | ||
{| class="wikitable mw-collapsible mw-collapsed" | {| class="wikitable mw-collapsible mw-collapsed" | ||
Line 307: | Line 717: | ||
| rowspan="6" |1 | | rowspan="6" |1 | ||
|Geert Touw | |Geert Touw | ||
| | |Group setup etc (2h), Subject brainstorm (2h) | ||
| | |4h | ||
|- | |- | ||
|Luc van Burik | |Luc van Burik | ||
| | |Group setup etc (2h), Subject brainstorm (2 h) | ||
| | |4h | ||
|- | |- | ||
|Victor le Fevre | |Victor le Fevre | ||
| | |Group setup etc (2h), Subject brainstorm (2h) | ||
| | |4h | ||
|- | |- | ||
|Thijs Egbers | |Thijs Egbers | ||
| | |Group setup etc (2h), Subject brainstorm (2h) | ||
| | | | ||
|- | |- | ||
|Adrian Kondanari | |Adrian Kondanari | ||
| | |Group setup etc (2h), Subject brainstorm (3h) | ||
| | |5h | ||
|- | |- | ||
|Aron van Cauter | |Aron van Cauter | ||
| | |Group setup etc (2h) | ||
| | | | ||
|- | |- | ||
| rowspan="6" |2 | | rowspan="6" |2 | ||
|Geert Touw | |Geert Touw | ||
| | |Meeting 1 (1h), Meeting 2(1h), Subject brainstorm (1h) | ||
| | |3h | ||
|- | |- | ||
|Luc van Burik | |Luc van Burik | ||
| | |Meeting 1 (1h), Meeting 2(1h), Subject brainstorm (2h) | ||
| | |4h | ||
|- | |- | ||
|Victor le Fevre | |Victor le Fevre | ||
| | |Meeting 1 (1h), Meeting 2(1h), Subject brainstorm (1h) | ||
| | |3h | ||
|- | |- | ||
|Thijs Egbers | |Thijs Egbers | ||
| | |Meeting 1 (1h), Meeting 2(1h), Subject brainstorm (2h) | ||
| | |4h | ||
|- | |- | ||
|Adrian Kondanari | |Adrian Kondanari | ||
| | |Meeting 1 (1h), First concept elaboration (2h) | ||
| | |3h | ||
|- | |- | ||
|Aron van Cauter | |Aron van Cauter | ||
| | |Meeting 1 (1h) | ||
| | | | ||
|- | |- | ||
| rowspan="6" |3 | | rowspan="6" |3 | ||
|Geert Touw | |Geert Touw | ||
| | |Meeting 1 (1h), meeting 2 (1h), Oceanic weather + hypothermia and water + shipping routes research (3h) | ||
| | |5h | ||
|- | |- | ||
|Luc van Burik | |Luc van Burik | ||
| | |Meeting 2 (1h), IAMSAR reading (3.5h), Drone navigation research (1.5h) | ||
| | |6h | ||
|- | |- | ||
|Victor le Fevre | |Victor le Fevre | ||
| | |Meeting 1 (1h), Meeting 2 (1h), Made the wiki look a bit more coherent (1h) | ||
| | |||
Wrote the problem statement and objective, users, scenario and added points to the MoSCoW (1.5h) | |||
Read Sensor and Image Recognition papers (1h) | |||
|5.5h | |||
|- | |- | ||
|Thijs Egbers | |Thijs Egbers | ||
| | |Meeting 1 (1h), meeting 2 (1h), sea communication research (2h) | ||
| | |4h | ||
|- | |- | ||
|Adrian Kondanari | |Adrian Kondanari | ||
| | |Meeting 1 (1h), requirements (2h), MOB protocols (2h) | ||
| | |5h | ||
|- | |- | ||
|Aron van Cauter | |Aron van Cauter | ||
Line 382: | Line 796: | ||
| rowspan="6" |4 | | rowspan="6" |4 | ||
|Geert Touw | |Geert Touw | ||
| | |Meeting 1 (1h), Meeting 2(1h), Ethics (4h) | ||
| | |6h | ||
|- | |- | ||
|Luc van Burik | |Luc van Burik | ||
| | |Meeting 1 (1h), Meeting 2(1h), Search area (1.5hr), Drone search speed (1.5 hr), Wiki writing (0.5 hr) | ||
| | |5.5h | ||
|- | |- | ||
|Victor le Fevre | |Victor le Fevre | ||
| | |Meeting 1 (1h), Meeting 2(1h), looked at sensors and image recognition (2h) | ||
| | |4h | ||
|- | |- | ||
|Thijs Egbers | |Thijs Egbers | ||
| | |Meeting 1 (1h), Meeting 2(1h), drone controll research (3h) | ||
| | | | ||
|- | |- | ||
|Adrian Kondanari | |Adrian Kondanari | ||
| | |Meeting 1 (1h), Meeting 2(1h), sensor research (2h) | ||
| | |5h | ||
|- | |- | ||
|Aron van Cauter | |Aron van Cauter | ||
Line 407: | Line 821: | ||
| rowspan="6" |5 | | rowspan="6" |5 | ||
|Geert Touw | |Geert Touw | ||
| | |Meeting 1(1h), Made a search pattern with Victor (2h) | ||
| | |3h | ||
|- | |- | ||
|Luc van Burik | |Luc van Burik | ||
| | |Meeting 1(1h), Working on Image detection(2.5h), meeting 2 (1h), Square search (2.5h), | ||
| | adapted square search(WIP) (1h) | ||
|8 h | |||
|- | |- | ||
|Victor le Fevre | |Victor le Fevre | ||
| | |Meeting 1(1h), designed and tried tried to make an xy-rig (3h), worked on the wiki (2h) | ||
| | |6h | ||
|- | |- | ||
|Thijs Egbers | |Thijs Egbers | ||
| | |Meeting 1(1h), meeting 2 (1h), worked on getting the raspi setup (3h), | ||
| | try to get the camera and soldering (0.5h) | ||
|5.5h | |||
|- | |- | ||
|Adrian Kondanari | |Adrian Kondanari | ||
| | |Meeting 1(0.5h), communication methods research (4h) | ||
| | |4.5h | ||
|- | |- | ||
|Aron van Cauter | |Aron van Cauter | ||
| | |Meeting 1(1h) | ||
| | | | ||
|- | |- | ||
| rowspan="6" |6 | | rowspan="6" |6 | ||
|Geert Touw | |Geert Touw | ||
| | |Meeting 1 (1h), Meeting 2 (1h), Worked on wiki (2h) | ||
| | |4h | ||
|- | |- | ||
|Luc van Burik | |Luc van Burik | ||
| | |meeting 2 (1h), Concert search pattern to work inside rest of the program (3h), programming more patterns(2h) | ||
| | |6h | ||
|- | |- | ||
|Victor le Fevre | |Victor le Fevre | ||
| | |Meeting 1 (1h), Meeting 2 (1h), Worked on wiki (4h) | ||
| | |6h | ||
|- | |- | ||
|Thijs Egbers | |Thijs Egbers | ||
| | |Meeting 1 (1h), Meeting 2 (1h), get the camera setup (1.5h), try to optimise camera reading (1h), | ||
| | convert search pattern to work inside the rest of the program (3h) | ||
|7.5h | |||
|- | |- | ||
|Adrian Kondanari | |Adrian Kondanari | ||
| | |Heating element (5h) | ||
| | |5h | ||
|- | |- | ||
|Aron van Cauter | |Aron van Cauter | ||
Line 457: | Line 874: | ||
| rowspan="6" |7 | | rowspan="6" |7 | ||
|Geert Touw | |Geert Touw | ||
| | |Meeting 2 (demo) (4h), Wiki (1h) | ||
| | |5h | ||
|- | |- | ||
|Luc van Burik | |Luc van Burik | ||
| | |Meeting 2 (demo) (4h), finishing touches test program (2h), presentation (3 h) | ||
| | |9h | ||
|- | |- | ||
|Victor le Fevre | |Victor le Fevre | ||
| | |Meeting 2 (demo) (4h), Wiki (1h) | ||
| | |5h | ||
|- | |- | ||
|Thijs Egbers | |Thijs Egbers | ||
| | |Meeting 1 (1h), meeting 2/video making (4h), finishing touches test program (2h), | ||
make presentation (WIP) | |||
| | | | ||
|- | |- | ||
|Adrian Kondanari | |Adrian Kondanari | ||
| | |wiki (2h) | ||
| | |2h | ||
|- | |- | ||
|Aron van Cauter | |Aron van Cauter | ||
Line 482: | Line 900: | ||
| rowspan="6" |8 | | rowspan="6" |8 | ||
|Geert Touw | |Geert Touw | ||
| | |Presentation (0.5h), Short meeting to prepare for presentations (0.5h), wiki (1.5) | ||
| | |2.5h | ||
|- | |- | ||
|Luc van Burik | |Luc van Burik | ||
| | |presentations (2.5 h), Short meeting before presentations (.5h), working on wiki (6h) | ||
| | |9h | ||
|- | |- | ||
|Victor le Fevre | |Victor le Fevre | ||
| | |Finalizing wiki (5h) | ||
| | |5h | ||
|- | |- | ||
|Thijs Egbers | |Thijs Egbers | ||
| | |Presentations (2.5h), short meeting with Luc (0.25h), working on the wiki (4h) | ||
| | | | ||
|- | |- | ||
|Adrian Kondanari | |Adrian Kondanari | ||
| | |wiki (4h) | ||
| | |4h | ||
|- | |- | ||
|Aron van Cauter | |Aron van Cauter | ||
| | | | ||
| | | | ||
|} | |}<br /> | ||
<br /> | |||
===References=== | ===References=== | ||
<references /> | <references /><br /><references /> | ||
Latest revision as of 22:39, 10 April 2023
Name | Student number | Major |
---|---|---|
Geert Touw | 1579916 | BAP |
Luc van Burik | 1549030 | BAP |
Victor le Fevre | 1603612 | BAP |
Thijs Egbers | 1692186 | BCS |
Adrian Kondanari | 1624539 | BW |
Aron van Cauter | 1582917 | BBT |
Abstract
In this wiki our group examined the issue of man overboard (MOB) incidents and proposes a solution to increase the survival chances of victims. A state-of-the-art literature review was conducted, which explored various aspects of MOB incidents such as search area, search patterns, oceanic currents and temperatures, and current person in water detection technology such as drones. A proof of concept was then developed using an XY-rig, which incorporated a thermal camera connected to a Raspberry Pi. The results showed that the thermal camera was able to successfully locate a heat element in a bland environment, representing the ocean. In future work, the solution will be extended to a drone swarm, where multiple drones can be deployed to search for a victim. This solution has the potential to improve the effectiveness of search and rescue operations, and ultimately increase the survival rate of MOB victims.
Introduction
Working on board a cargo ship is a dangerous occupation, with man overboard (MOB) incidents being a particularly serious concern. 91 fatalities were reported as a result of MOB events aboard cargo ships between 2014 and 2021[1]. Numerous factors, including; bad weather, unstable loads, and a lack of adequate safety equipment, are the main cause of such accidents. Unfortunately, locating a MOB victim in the ocean can be a challenging and complicated task. The search procedure can be affected by all sorts of elements such as the climate, ocean currents, and visibility.
To better understand the scope of the issue, a 2020 study analyzed 100 MOB incidents using 114 parameters to generate a MOB event profile[2]. 53 of the 100 events that were looked at occurred on cargo ships. In 88 cases, the casualty was deceased as a result of the MOB incident, from those 88 cases 34 were assumed dead and 54 were witnessed dead. From the witness deaths 18 died before the rescue and 31 after the rescue, in 5 cases it is unknown. The cause of death was indicated for 42 cases, the most common cause is identified as drowning (26), followed by trauma (9), cardiac arrest (4) and hypothermia (3).
Despite the challenges involved in locating MOB victims in the ocean, we believe that technology can play a crucial role in increasing the survival chances of those involved in such incidents. With this in mind, we aim to develop a device that can improve the efficiency of locating MOB victims on cargo ships. Specifically, we will focus on MOB incidents that occur on cargo ships, as the 2020 study indicated that over half of such incidents occur on these vessels. The end users of our product are personnel of cargo ships, shipping companies that own a fleet of cargo ships, ports, and possibly the military.
It's worth noting that searching for a victim in the ocean can be a challenging and complex task, we therefore make several assumptions. First, we presume that both at the time of the accident and during the search and rescue effort, the weather conditions are mild. This means mild waves, a mild breeze, little rain, and average sea and air temperatures. We also assume that it is night-time at the time of the incident and that there is only one victim who is capable of maintaining afloat and does not have any major injuries. Additionally, we assume that the time of the incident is known, allowing for a general understanding of the search area.
To develop our device, we will conduct a state-of-the-art literature study to investigate various factors that can impact the search process. This will include examining search areas, search patterns, oceanic currents and temperatures, and current person-in-water detection technology.
We hope to increase the efficiency of the search process and further improve the survival chances of those involved in MOB incidents on big (cargo) ships. By developing this device, we believe that we can make a meaningful contribution to the safety of those who work at sea.
State-of-the-art literature study
Before we can get started with the project it is important to gather knowledge about the state-of-the-art (SotA) concerning safety measures and pre-existing technology that aids victims of a MOB incident. We therefore performed a SotA literature study.
Current search methods
Search procedures for when a man goes overboard already exist. In the IAMSAR manual some of these procedures are explained. In this paragraph we discuss and analyze these procedures and go over some different search patterns used.
Current Procedures[3]
The existing procedures for people overboard on big cargo ships are quite elaborate. The procedure basically consists of a few key steps: it all starts when a victim goes overboard, from there the following will happen:
Step 1: Notify staff on board
Step 2: Mark the position the victim went overboard / release a marker
Step 3: Turn the ship around
Step 4: Final search (finding the marker and victim)
Step 5: Rescue
At first this process seems quite efficient, but what should be taken into account is that Step 3, can take a long time for big ships[4] . For some ships this can take over 20 minutes while traveling a few kilometers. Then there are also some factors going into the step 4: For different environments and conditions different patterns are recommended. Some of these will be explained below.[5]
Expanding Square Search
The expanding square search is a method that can be used to systematically inspect a body of water for signs of an MOB victim. The search should start at the last known location of the victim, from that point the search starts and expands outwards with course alterations of 90°, as can be seen in the image provided. The course of expansion heavily depends on the specification of the device that will be used. Thermal imaging can be used to scan the area, looking for any signs of the victim such as floating debris or the person themselves.
Sector Search
Sector search is another technique that can be used to search for a victim in the ocean. The sector search technique involves dividing the search area into sectors or pie-shaped sections and systematically searching each sector. It again starts at the last known location of the victim. From there the search area is divided and each sector is carefully searched in a clockwise fashion. Sector search can be effective in searching large areas, especially in cases where the search area is relatively circular or symmetric. However, sector search may not be the most efficient or effective method in all situations, especially if the search area is irregular or if weather and ocean conditions make it difficult to maintain a consistent search pattern.
Sweep search
The sweep search pattern is another technique that can be used to search for a victim in the ocean. It involves moving the search vessel or search device in a back-and-forth sweeping motion across the search area.
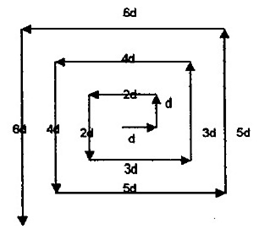


When the location of the MOB victim is known well, the Expanding Square or Sector Search is recommended. If the location of the accident is not accurately known different patterns are recommended such as Sweep Search. IAMSAR also mentions other factors that need to be taken into account during the search. If a person falls into the water they will for example be moved away by currents.
Search area
A person floating in the ocean can drift up to 10 nautical miles per day, this is equal to 0.2 meters per second. If the current is unknown this will mean the search area would expand in a circle if the starting location would be known. If we define the search area as a circle with a radius r, the radius of the circle will depend on time, we describe the search area with the following formula: r = 0.2 t. Here t is the time since the person fell overboard. The following table shows the search area size compared to time.
Time overboard (minutes) | Search area size (m²) |
1 | 452 |
5 | 11310 |
15 | 101788 |
The table clearly shows the search problem can become quite big. Especially when the search takes a long time. This shows the importance of finding the victim quickly.
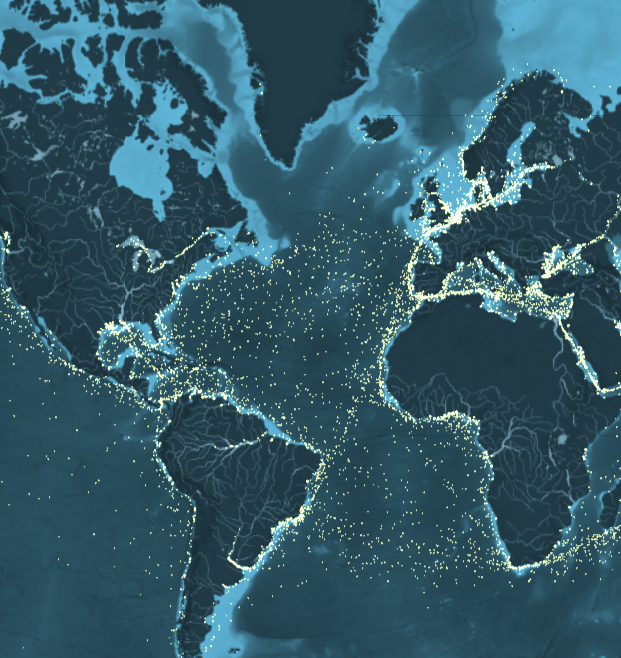
Influence of cold water and oceanic temperatures
Having a good understanding on how factors such as water temperature influence the rescue mission is crucial in ensuring that the rescue mission is successful. Depending on the water temperature, the time frame of the rescue mission changes and different measures need to be taken into account. Ideally the victim should be rescued as soon as possible, but having a known time frame of within which the mission needs to be executed gives the crew members a good idea on how to approach the situation.
Since we're dealing with people who have fallen in water, we need to consider their survival time. When being submerged in water, your greatest risk is developing hypothermia as your body cools down 25 times faster in water than in air of the same temperature. The effects of hypothermia go from confusion and exhaustion, to unconsciousness and coma, and in the end lead to death. It is thus of utmost importance to detect and rescue a person in the water before the severe effects of hypothermia set in. The following table shows the relation between the temperature of the water and the time frame of the effects of hypothermia. The time windows are wide as the time when the effects of hypothermia begin depend on several factors, like age and percentage of body fat.[9]
Water temperature (C°) | Time until unconsciousness | Survival time |
---|---|---|
0 | <15 min | 15 - 45 min |
0 - 5 | 15 - 30 min | 30 - 90 min |
5 - 10 | 30 - 60 min | 1 - 3 hours |
10 - 15 | 1 - 2 hours | 1 - 6 hours |
15 - 20 | 2 - 7 hours | 2 - 40 hours |
20 - 25 | 3 - 12 hours | 3 - indefinite |
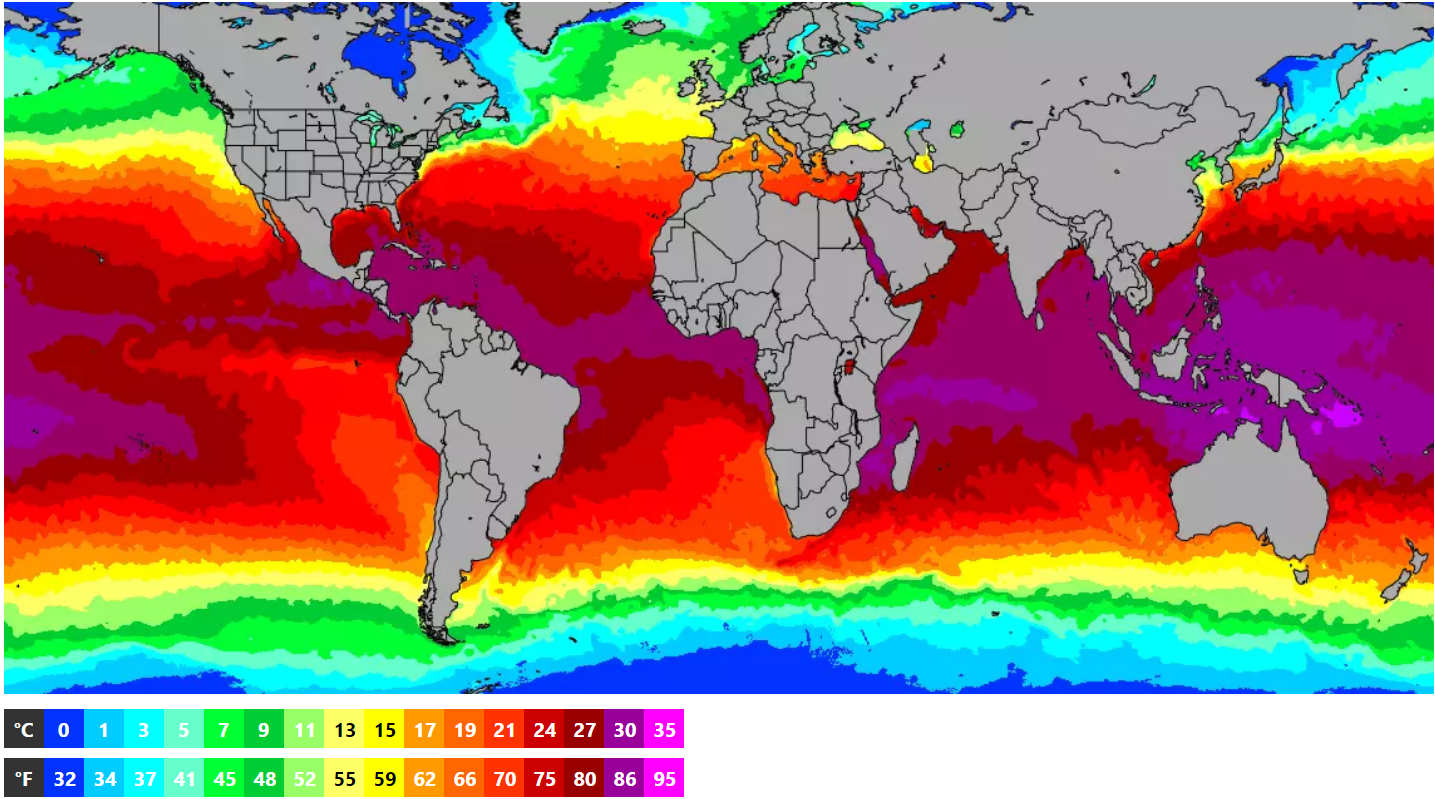
Next, oceanic temperatures need to be considered. The two figures on the right show the world's shipping routes on the Atlantic ocean, and oceanic temperatures respectively. From this we can deduce that there are busy shipping routes in the colder regions of the Atlantic ocean, ranging from 3 to 7 degrees Celsius, and also the occasional ship in water at 1 degrees Celsius. The temperatures were taken in late March so it might even be colder in the middle of winter. Since these ships will benefit most from a technology that might result in faster rescue times, it can be concluded at the most desirable performance of the system is to find a person in the water in less than 10 minutes, leaving enough time for the rescue operation to start.[10][8]
Possible detection methods
There already exists technology that can detected a person in a body of water. Radars are frequently used to detect the distance and velocity of an object by sending electromagnetic waves out and detecting the echo that results from objects. In water environments, a radome is needed to protect the radar but let the electromagnetic waves through. However, even with a radome the signal strength already falls to a third in a wet environment compared to dry. Multiple radar technologies exist with each their different advantages and disadvantages. Pulse Coherent Radar (PCR) can pulse the transmitting signal so it only uses 1% of the energy. Another aspect of this radar is coherence. This means that the signal has a consistent time and phase so the measurements can be incredibly precise. It can also separate the amplitude, time and phase of the received signal to identify different materials. This is not necessarily needed in our use case. The 'Sparse' service is the best service for detecting objects since it samples waves every 6 cm. You don't want millimeter precise measurements in a rough environment like the ocean so these robust measurements are ideal.[11]
Another detection method that does not yet exist is to send out a swarm of drones to search for victim. The research paper "Intelligent Drone Swarm for Search and Rescue Operations at Sea"[12] presents a novel approach for using a swarm of autonomous drones to assist in search and rescue operations at sea. The authors propose a system that uses machine learning algorithms and computer vision techniques to enable the drones to detect and classify objects in real-time, such as a person in distress or a life raft. The paper describes the hardware and software architecture of the drone swarm system, which consists of a ground station, multiple drones equipped with cameras, and a cloud-based server for data processing and communication. The drones are programmed to fly in a coordinated manner and share information with each other to optimize the search process and ensure efficient coverage of the search area. The authors evaluate the performance of their system in various simulated scenarios, including detection of a drifting boat, a person in the water, and a life raft. The results show that the drone swarm system is capable of detecting and identifying objects accurately and efficiently, with a success rate of over 90%. The research paper presents a promising solution for improving search and rescue operations at sea, leveraging the capabilities of autonomous drones and artificial intelligence. For larger scale operations, drone swarm can be used to cover greater areas for search and rescue. This method is useful when other factors such as the wind, current etc. make finding the victim more difficult. The drone swarm would be implemented in such a way so that areas around the ship, or any search area for that matter, can be searched in a shorter time span, increasing the chances of finding the victim and increasing the chances of survival. When multiple drones are deployed, they all take up a specific search pattern in unison. Depending on the situation different search patterns will be used. If a man overboard is detected rather quickly, one search pattern may be implemented in order to optimize the time it takes in order to locate the victim. When other factors influence the case, such as wind or current, different search patterns will be used in order to adapt to the situation.
There are multiple papers that discuss drone swarms as a method of detecting people on the ocean. The research paper "AutoSOS: Towards Multi-UAV Systems Supporting Maritime Search and Rescue with Lightweight AI and Edge Computing"[13] proposes a similar approach for using multiple unmanned aerial vehicles (UAVs) equipped with lightweight AI and edge computing to support maritime search and rescue (SAR) operations. The authors describe the AutoSOS system, which consists of a ground station, multiple UAVs, and a cloud-based platform for data processing and communication. The UAVs are equipped with cameras and other sensors to detect and classify objects in real-time, such as a person in the water or a life raft. The lightweight AI algorithms are designed to operate on the UAVs, minimizing the need for high-bandwidth communication with the cloud-based platform. The paper highlights the advantages of using a multi-UAV system for SAR operations, such as increased coverage area, improved situational awareness, and faster response times. The authors also discuss the technical challenges of designing and implementing the AutoSOS system, such as optimizing the UAV flight paths and ensuring the reliability of the communication and data processing systems. To demonstrate the effectiveness of the AutoSOS system, the authors present a case study of a simulated SAR mission in a coastal area. The results show that the system is capable of detecting and identifying objects accurately and efficiently, with a success rate of over 90%.
Another paper that is of relevance is the "A Review on Marine Search and Rescue Operations Using Unmanned Aerial Vehicles"[14]. It provides a comprehensive review of the current state of research on using unmanned aerial vehicles (UAVs) for search and rescue (SAR) operations in marine environments. The paper discusses the main challenges of conducting SAR operations in marine environments, such as limited visibility, harsh weather conditions, and the vast and complex nature of the search areas. The authors then review the different types of UAVs and their applications in SAR operations, such as fixed-wing UAVs for long-range surveillance and multirotor UAVs for close-range inspection and search missions. The paper highlights the advantages of using UAVs for SAR operations, such as increased coverage area, improved situational awareness, and reduced risk to human rescuers. The authors also discuss the technical challenges of designing and operating UAVs in marine environments, such as optimizing the flight paths, ensuring reliable communication and data transmission, and complying with regulatory and ethical guidelines. To demonstrate the effectiveness of UAVs for SAR operations, the authors review several case studies of real-world applications, such as detecting and rescuing distressed vessels and locating missing persons in coastal areas. The results show that UAVs can provide valuable support to SAR operations, improving response times, and increasing the chances of successful rescue.
Another paper, "Requirements and Limitations of Thermal Drones for Effective Search and Rescue in Marine and Coastal Areas"[15], explores the potential of thermal drones for search and rescue (SAR) operations in marine and coastal areas. The authors review the main features and capabilities of thermal drones, including their ability to detect heat signatures and identify objects in low light or night conditions. The paper highlights the key requirements and limitations of using thermal drones for SAR operations, such as the need for high-resolution thermal cameras, long flight time, and reliable communication systems. The authors also discuss the challenges of operating drones in harsh weather conditions and the importance of complying with regulatory and ethical guidelines. To demonstrate the effectiveness of thermal drones for SAR operations, the authors present a case study of a simulated rescue mission in a coastal area. The results show that thermal drones can provide valuable assistance in locating and identifying missing persons or distressed vessels, particularly in remote or inaccessible areas. The research paper provides a comprehensive overview of the potential of thermal drones for SAR operations in marine and coastal areas, while also highlighting the technical and operational challenges that need to be addressed to ensure their effective use.
From these studies we conclude that the most efficient detection method is a drone swarm with thermal cameras attached to the drones.
Sensors
The main sensor that will be used to locate a victim is a thermal imaging camera (TIC). Since the general search area is small relative to the complete ocean, temperatures of the water will all be relatively the same. The victim will have a much higher temperature than their surroundings, making it easy to detect them. There are a couple important requirements for the TIC. The camera needs a sufficiently high resolution so that we can clearly distinguish the victim from other warm object (think of the marine life). It also needs to have a high refresh rate. Our goal is to detect and locate a victim as soon as possible so that the survival chance is the highest, we therefore need to 'scan' the search area fast and that requires a sufficient refresh rate. For the same reason the thermal camera is required to have a large field of view (FOV). It also needs the right temperature sensitivity.
Other sensors and actuators that we might want to include are a microphone and a loudspeaker. This would allow us to make contact with the victim. However, the issue with a microphone is that the sound of the ocean is most likely so loud that it is unlikely to understand the victim, trying to conversate with the victim might also fatigue them. A loudspeaker can be used to notify the victim rescue is on the way and help them calm down.
Thermal image recognition
For the image recognition we can make use of TensorFlow, Google's Neural Network library. We can, as mentioned before, also choose to not do advanced image detection and instead as soon as a heat source that could reasonably be the victim is detected, send the camera images to the ship where a human operator can verify whether the heat source is actually the victim.
Another option that could be looked into more would be using a hybrid of these two strategies. Namely, using the fast simple heat source detection until a heat source is found and then activating the more advanced AI image detection to verify if it is a human. This would then solve a part of the efficiency problem and would eliminate some need for human operators and higher data transfer requirements.
Communication Systems
In a scenario where a drone needs to send data, such as thermal images, to a container ship on the open sea, there are several reliable methods of communication that can be used, including:
- Satellite Communication: One of the most reliable methods of communication in remote areas, such as the open sea, is through satellite communication. The drone can use a satellite modem to transmit the data to a satellite in orbit, which then relays the data to a ground station or directly to the container ship. Satellite communication can support high data rates and can be used to transmit data over long distances.
- Long-Range Wi-Fi: Another option for communication between the drone and the container ship is through long-range Wi-Fi. The drone can be equipped with a Wi-Fi transmitter that is capable of transmitting data over a long distance, and the container ship can be equipped with a Wi-Fi receiver that is capable of receiving the data. Long-range Wi-Fi can support high data rates and can be used to transmit data over several kilometers, depending on the power of the transmitter and receiver.
- Cellular Communication: Depending on the location of the container ship and the availability of cellular coverage, the drone may be able to use cellular communication to transmit data to the ship. The drone can be equipped with a cellular modem that is capable of connecting to a cellular network, and the container ship can be equipped with a cellular receiver that is capable of receiving the data. Cellular communication can support moderate data rates and can be used to transmit data over several kilometers, depending on the strength of the cellular signal.
- Radio Communication: Another option for communication between the drone and the container ship is through radio communication. The drone can be equipped with a radio transmitter that is capable of transmitting data over a long distance, and the container ship can be equipped with a radio receiver that is capable of receiving the data. Radio communication can support low to moderate data rates and can be used to transmit data over several kilometers, depending on the power of the transmitter and receiver.
Overall, the choice of communication method will depend on the specific requirements of the scenario, such as the distance between the drone and the container ship, the data rate requirements, and the availability of communication infrastructure. Satellite communication is typically the most reliable and versatile option in remote areas, but it may also be the most expensive.[16]
Design
Requirements
Must | Should | Could | Won't |
---|---|---|---|
Have sensors to detect a person within 10 minutes | Have life assist systems | Have communication between victim and ship | Have the ability to take the person to safety |
Have a communication system to communicate with the involved ship | Resist (bad) weather to a degree | ||
Be able to withstand an air temperature range of -3°C to 36°C. | A person recognition algorithm | ||
Be able to operate in the dark | |||
Be able to operate in the rain | |||
Be able to withstand wind forces of 4 beaufort. |
Drone requirements
A couple of requirements need to be met in order for the drone to be functioning as intended. In this section we will discuss the possibilities of remote drone control and how it ties into our case. Different communication methods will be discussed and we have to choose the best one for our scenario. And the detection method using thermal imaging will also be discussed. This is also tied with the drone search speed, which also influences our approach.
Remote Drone Control
A lot of research has already gone into autonomous drone flight. Take a look at autonomous drone racing for example[17] . A lot of consumer drones also are capable of autonomous flight, for example DJI has a waypoint system based on GPS coordinates and their drones are also capable of tracking a moving person/object. DJI's waypoint system works by loading a set of GPS coordinates onto the drone, the drone will navigate itself to the first set coordinates. From there it goes on to the next. Until it has reached the final set of coordinates. The moving object tracking is a bit more complicated. This involves image recognition.[18]
However, the firmware on most of these commercial drones is not open to being interfaced with. This makes is much more difficult to make a more autonomous drone without building one from scratch. Another point is that drones, and especially the autonomous kind, are quite expensive. And due to the aforementioned lack of interfacing options with the flight controllers of commercial drones it is also not really possible to take a cheaper non-autonomous drone and hook it up to a computer that will act as a module to make the drone fly autonomously.
Drone search speed
The drone search speed will be limited by multiple things such as flight speed, sensor resolution and quality/speed of the detection algorithm. Since we still have some unknowns we would like to make some assumptions. Lets assume the algorithm is able to detect a human if they take up at least a space of 25 by 25 pixels. Lets also assume a human at sea takes up at least 25 by 25 cm. A good sensor to use for the detection of humans at sea in the night would be a heat camera. These cameras take images in the IR spectrum and thus aren't hindered by low light conditions. They have a field of view (FOV), a resolution and a refresh rate. The height the drone can fly will be determined by the FOV, and resolution. A typical FOV for Raspberry Pi cameras is about 60 degrees of horizontal[19] and a typical resolution would be 1080*1920 pixels. Here 1920 is the horizontal direction. If the drone would have a sensor of these specs it would be able to fly about 15 meters high, here it would be able to scan a line of 19.2 meters wide. Lets assume the algorithm needs to have the human in shot for 5 seconds for it to be detected by the algorithm.[20] The vertical FOV of Raspberry Pi sensors is about 40 degrees, this would result in a distance of about 10 meters vertical to be seen by the drone. This means it can fly at about 2 m/s. The area the drone can covers is thus 20m*2m/s*t where t is the time since dispatched. Another solution to the detection could be to not look for humans specifically, but for relatively hot areas in the image. This might be an option since out at sea there is a significant heat difference between a human with a body temprature of approximately 37 degrees celcius and the environment that consists mostly of cold water. When using this strategy it is however necessary to include some kind of verification in the system so that the drone will not signal that the victim is found when it, for example, finds a maritime animal close to the surface of the water.
However, for our estimation of the search speed we will still use the option where an algorithm is used that actually detects a human since this might still be necessary and we prefer to work from the worst case scenario with regards to bounding the minimum required efficiency. In the graph on the right we see that after about 5 minutes the drone is not able to keep up with the search area anymore (even with a perfect search pattern). If we start taking dispatch time into account it would mean these 5 minutes would be about result in about 2.5 of effective search time) this would move the yellow line to the left, this would also mean that one drone is not quick enough. and we would likely need to use multiple drones in order to search fast enough..
Components
Sensor
- Thermal imaging camera
Communication Equipment
- Wireless communication module
Drone with autonomous/waypoint flight capabilities
Small computer capable of:
- Multithreading/multiprocessing
- Running TensorFlow or other image recognition AI software
- Interfacing with electronics (reading the camera image and possibly interfacing with servo motors)
- Running autonomous flight control software
Drone
For our real design we would like to use a drone that is able to fly accurately in mild wind and is able to carry some weight. Since the drone has to fly autonomously it will most likely be necessary to make a custom drone for this project since almost all commercially available drones don't allow interfacing with the flight controller in such a way that an external device/computer can control the drone. We have not really researched into how to make a drone from scratch since this is not really in the scope of the project, however for the autonomous flight a pixhawk flight controller can be used. The pixhawk is currently the most popular option for autonomous flight as far as we can tell. The pixhawk can then be interfaced with with a Raspberry Pi to controll the autonomous movement of the drone.
We have also not researched into the details of the control of autonomous drones since we already found out pretty early that using an actual drone like this for the proof of concept would not be feasable. The details of the control software would not really contribute to the rest of the design so for our demo we assumed it was possible to transform carthesian waypoints to flight instructions for the drone.
Computer
We have decided to use a Raspberry Pi as our computer due to it's computing power to size/weight ratio. The Raspberry Pi can do all the things we wish our computer to do so it is a great choice.
Search pattern
A critical factor that can determine the effectiveness of a search operation and its ability to meet our requirements is the search pattern employed. We have identified three potential search patterns that could be utilized, and we plan to test each of these methods using a model to evaluate their effectiveness. The selection of an appropriate search pattern is crucial as it can have a significant impact on the chances of success in locating the person who has fallen overboard. By testing each of the proposed search patterns using a model, we can determine which method is best suited for the specific circumstances of the search and optimize our search efforts accordingly.
One already existing search pattern is the expanding square search. This involves the drone moving outward from the last known location of the person in a square pattern, with each pass covering an increasing amount of area. This method is efficient and can cover a lot of ground quickly, but it does not take into account the direction of the current, so could in theory only be efficiently used in situations where the victim doesn’t drift.
To address this issue, an improvement on the expanding square search is to incorporate the direction of the current. We call this Adapted Square Search. This involves adding a few repetitions in the direction of the current to increase the chances of finding the missing person. The amount of repetitions is determined by the strength of the current; No current would mean no repetitions (regular expanding square), a weak current one extra repetition in that direction, a bit stronger two repetitions, etc. This method can be especially useful when there is a strong current that could have carried the person further away from their original location.
Another possible search pattern is the cone pattern. This involves the drone moving outward from a small distance before the last known location of the person in a cone-shaped pattern, with the width of the cone expanding in the direction of the current. This method could be the most efficient as it doesn’t return back to the initial position and doesn’t search against the current.
Then there is also a different approach, since the current moves the victim will move, we can also wait for it to come to us. This method is called wait and sweep, here the drone does a sweep search pattern, but it sweep the same area over and over.
Programming
All of the code used in this project is uploaded on the following GitHub repository: https://github.com/ThijsEgberts/ProjectRobotsEverywhere.
Proof of concept
In order to prove the concept not all Moscow requirements need to be fulfilled. Since the most important part of our system is the detection, this needs to be shown working. The algorithms for both search and detection need to be proven and the hardware also needs to be shown functioning. However weather resistibility, does not have to be taken into account yet. The test also does not have to be done at full scale.
Designing and building
Materials:
- Plastic container 40cm x 60cm
- 2 Metal rods 8 mm x 100cm
- Linear ball bearing
- ABS (to print pieces)
The design process of the prototype was done with Fusion 360. This program allows for detailed 3D-modeling and easy conversion to Prusaslicer which is the slicer program. After that the pieces that were designed are ready to be printed. The 3D-printer that was used is the Voron V0. We made a total of 4 corner attachment pieces, 2 sliders and 1 slider platform. The whole process required a lot of little design changes to properly fit and assemble the entire setup. Eventually the final assembly process was easily done with some screws and bolts. The search pattern was drawn on the bottom of the container to help the people moving the camera with following the movement instructions and make it more clear for the demonstration video what was going on.
Demo
A Raspberry Pi and a thermal camera were mounted on a rig to demonstrate the proof of concept. Ropes were used to manually modify the camera's position. To make it easier to follow the search path, a map of the search pattern was drawn on paper. A tiny tea light was used as a heat source to imitate the relevant scenario and provide a distinct heat signature. Also, a rope that was tied to the lamp served as an ocean current replica. The heat source was identified by following the search pattern according to instructions being output by the Raspberry Pi.
Thermal camera
For our demo we used the MLX90640 thermal camera. This camera has a resolution of 32x24 pixels and a 55° field of view. The camera itself has a refresh rate of 60fps, however in our application this is limited due to the speed at which the I2C interface works. Therefore in our application we could process images for heat sources at about 7fps when not displaying the image and at about 1fps when displaying the image. This speed may in part be due the use of python but python did make the image manipulation and output much easier.
Heating element
For the experiment we needed to have a detectable heat signature. For this we chose the simplest possible solution, which was just having a candle. This gave us a detectable heat signature that we can work with.
Design of the Model
In order to investigate how efficient the drone is at finding the victim a model was developed. The workings of the model will be explained here:
The model simulates the victim moving in the water, for a few minutes before the drones arrive at the scene. After this dispatch time, it starts moving the drone(s) simultaneously to the victim. From here it checks if the victim is in view of the drone’s view every time the drone is moved to a new location. If this is the case, the victim is found and the search ends.
Victim movement is simulated using some randomness. The victim is moved every second at a random angle, this angle is taken using a normal distribution (centered at the angle the current is at). The victim is also moved forward every second, this distance is uniformly varied between the a flow rate of 5cm per second below and above the current. The randomness is included into the victim movement in order to have every search be a unique one, and sufficiently test the search algorithms.
Drone movement is a bit different from victim movement, this is totally not random. The drones are moved along a pre-determined search pattern.
The full code of the model can be found on our programming Github
Results
In this section we will talk about our results surrounding tests with our design, namely our physical demo test and our model tests.
Demo results
Our main demo results are that it is possible to detect heat sources and follow a search pattern with a Raspberry Pi drone model. For the demo we only executed the adapted expanding square search pattern due to practicalities with our test setup, namely that our test rig was relatively small and rectangular.
Model results
Different search patterns also influence the speed it takes to find the target. Multiple different patterns were investigated using a model made in Python. Using this model different search patterns were each tested for 10000 trials. A current of about 25 cm/s is used in the positive y direction. The drone has a field of view of 3 m by 2.4 m (this matches the FOV of the camera we used for the demo), and is moved at a max speed of 3m/s. 4 different patterns were investigated. These are Square Search, Adapted Square Search, Wait and Sweep, and a Cone search. The full results can be seen in the Appendix
Single drone
Square search is one of the most simple patterns. The patterns simply spiral out, in a square. This pattern is however not very applicable to our case. Since the target moves in (mostly) one direction, and is not standing still. This can also be seen in the simulation results. Of the 10000 trials done, only 1.4 % succeeded. With an average total time of 313 seconds including 4 minutes of dispatch time.
Since the Square search did not seem to work very well, an adapted version of the algorithm was set up, this Adapted Square search puts more focus on the flow direction, by looping back on itself . This pattern saw serious improvement over the ‘normal’ square search. The success rate went up to 22.4% with a total time of 326 seconds including the dispatch time.
Cone search is different from the previous two patterns. It only searches in one direction and sweeps with increasing width. For this pattern multiple different cones were used, one with an angle of ¼ pi radians, one with an angle of ½ pi radians, and finally one with an angle of ¾ pi radians. The success rate varied between these, with the highest of 57.7% corresponding to the ½ pi radians cone. The other success rates were 16.9% and 51.3% belonging to the ¼ pi radian and ¾ pi radian cones respectively. The average total time of success for the ½ pi cone was 343 seconds including the dispatch time.
Wait and Sweep is different from the other patterns, this pattern does not focus on covering new areas it instead focuses on searching the same area over and over again, waiting for the victim to drift into the frame. The parameters going into this search are mainly the distance from the spot the victim dropped into the water and the sweep width. Multiple different distances (2.4 m, 7.2 m, 12 m, 16.8 m, and 24 m) were tried with both a 15 m and 30 m sweep width. A distance of 16.8 m with a sweep with of 15 meters resulted in the highest success rate of 70.0% with a success time of 428 seconds including dispatch time.
One note here is that both Square search and Adapted Square search are a lot more efficient at lower flow rates. reducing the flow rates from 25 cm/s to 10 cm/s increases the success rates to 49.5% for normal square search and 92.5% for the adapted version. this is a very big improvement. While cone performs similarly and decrease in efficiency is seen for Wait and Sweep.
Multi drone
Both Square Search algorithms were not tested for multiple drones since the ‘normal’ square search was not very efficient and the adapted version is difficult to scale for multiple drones. The cone and sweep and wait patterns were however tested for multiple drones.
For both the cone and sweep and wait an increase in efficiency was found when implementing more drones. For both we saw more drones equal to a higher efficiency, however, for the sweep and wait the efficiency reaches a limit of about 95% (distance 15m, width 30m) while the cone also slowly gets more efficient, and reaches about the same limit of 95% (cone angle ½ pi radians). The cone has a 260-second average detection time while the sweep and wait takes a bit longer (288 seconds). These include the dispatch time. This means the cone will be a bit better on average.
Ethical analysis
The paper "Ethical concerns in rescue robotics: a scoping review"[21] describes seven ethical concerns regarding rescue robots. In this chapter, each ethical concern from the paper is summarized and then applied to our case.
Fairness and discrimination
Hazards and benefits should be fairly distributed to avoid the possibility of some subjects incurring only costs while other subjects enjoy only benefits. This condition is particularly critical for search and rescue robot systems, e.g., when a robot makes decisions about prioritizing the order in which the detected victims are reported to the human rescuers or about which detected victim it should try to transport first. For our scenario, when there is a situation where multiple people have fallen over board, discrimination is something to look out for. When searching for people, discrimination can not take place because if the robot has found one person it will continue looking for the others and since it does not take people out of the water it does not need to make decisions about prioritizing people. However, we are also considering dropping lifebuoys and here prioritizing order does come into account. In the situation where the robot can only carry one life vest, we propose that it drops the lifebuoy one the first person it finds, then comes back to the ship to restock, and continues the search. This will be the most efficient and also prevents discrimination between multiple victims.
False or excessive expectations
Stakeholders generally have difficulties with accurately assessing the capabilities of a rescue robot. May cause them to overestimate and rely too much on the robot and give false hopes for the robot to safe a victim. On the other hand, if a robot is underestimated, it may be underutilized in cases where it could have saved a person. For our scenario, it is important to accurately inform stakeholders about the capabilities and limitations of the rescue robot.
Labor replacement
Robots replacing human rescuers might reduce the performance of human contact, situational awareness and manipulation capabilities. Robots might also interfere with rescuers attempt to provide medical advice and support. In our case it is important to not fully take the human aspect out of the equation, the robot should be equipped with speakers for people on the ship to talk to the person. They can check how he is responding and reassure the victim that they’re coming to help. This will most likely help to calm the person. When the rescue attempt is being made by the crew or other rescuers, the drone should fly away since it’s no longer useful and as to not interfere with the rescue attempt.
Privacy
The use of robots generally leads to an increase in information gathering, which can jeopardize the privacy of personal information. This may be personal information about rescue workers, such as images or data about their physical and mental stress levels, but also about victims or people living or working in the disaster area. What this means for our scenario is that The information gathered by the robots is not shared with anyone outside professional rescue organizations and is exclusively used for rescue purposes. We should also try to limit the gathering of irrelevant data as much as possible.
Responsibility
The use of (autonomous) rescue robots can lead to responsibility ascription issues in the case when for example accidents happen. What this means for us is that we clearly need to state who is (legally) responsible in the case accidents happen during rescue (like operator, manufacturer etc.) Proposal: To ensure trust in our product, we should claim full (legal) responsibility as a manufacturer when accidents happen caused by design flaws or decisions made by the autonomous system.
Safety
Rescue missions necessarily involve safety risks. Certain of these risks can be mitigated by replacing operators with robots, but robots themselves, in turn, may determine other safety risks, mainly because they can malfunction. Even when they perform correctly, robots can still be harmful: they may, for instance, fail to identify and collide into a human being. In addition, robots can hinder the well-being of victims in subtler ways. For example, the authors argue, being trapped under a collapsed building, wounded and lost, and suddenly being confronted with a robot, especially if there are no humans around, can in itself be a shocking experience. Risks should be contained as much as possible, but we do acknowledge that rescue missions are never completely risk free.
Trust
Trust depends on several factors; reputation and confidence being the most important once. Humans often lack confidence in autonomous robots as they find them unpredictable. What this means for us is that we need to ensure that the robot has a good reputation by informing people about successful rescue attempts and/or tests, and limit unpredictable behavior as much as possible. We can also ensure trust by claiming full (legal) responsibility as a manufacturer when accidents happen caused by design flaws or decisions made by the autonomous system.
Conclusion
For the chosen scenario of search and rescue, the drones prove to be efficient tools in locating and helping victims. Specifically for man overboard situations, where the drone is able to cover large areas. These sorts of technologies prove to be efficient in increasing the rescue chances of such scenarios and can be expanded further than only maritime operations.
As we assumed in the beginning of the wiki that the chosen scenario excludes extreme weather conditions. In our case, mild wind and waves are tolerable as long as these sorts of conditions don't affect the operations of the drone. Certain cargo ship pathways were also chosen such that we avoid extremely cold waters (which are not common paths for ships anyways as seen in the state-of-the-art literature study).
When defining the search area, the current was also taken into account. Assuming that a person can drift up to 0.2 m/s, we calculated that the search area would be around 450 m². As for the search patterns, we can conclude that in the case where we know the exact direction a victim is drifting in, wait and sweep search is the most effective. However for a scenario where the exact drifting direction is less certain, the cone search is probably the best option. For single drone applications with a low drift rate the adapted square search pattern can also be useful.
For the construction of the drone we can conclude that it is a good idea to take a custom drone with a flight controller like the Pixhawk and then control the autonomous flight with Raspberry Pi. The Raspberry Pi will be connected to a thermal camera with a decently good resolution and framerate. The images captured by the camera will then be analyzed with TensorFlow to find victims in the image. When victims are found the ship will be notified of the location over radio communication.
Discussion
During this project there were quite some things that could have been done better. In the beginning of the course the biggest issue was defining the goal of the project and narrowing down the case. The first couple of weeks were spent on deciding on what we would do for this project and defining the specific preferences, requirements and constraints. This left us with a lot less time to go through all of the necessary design phases. The scale of the project was also changing as the weeks were going by. Some things that we wanted to do just weren't feasible in the amount of time that was left. The main example here was that we found out that actually getting an autonomous drone to work turned out to be very hard and expensive given how specific that use case is. The main problem was a combination of us either having to find a very expensive (in the thousands of euros) drone that can be made autonomous or built our own drone from scratch, which would still be pretty expensive and take a lot of effort.
Looking back on the course, the biggest issue that should have been tackled was the planning and making the decision to go for the vision that we had in the beginning. The weekly goal had to be more ambitious as we were left with little time for the project. The vision of the project should have been narrowed down for a specific case like the one that we have right now somewhere around the first week. After this a concrete plan on the whole approach should have been made rather than making up on the spot what needed to be done.
It might also have been a good idea to look into the feasibility of making a physical prototype earlier on in the project so that we could make better decisions surrounding this given that in the way it turned out, our physical demo was more a way of showing that the control software and automatic detection is possible instead of also testing our search patterns in real life.
As for our simulations, they are definitely useful, but do not account for more complex movement of the victim or the influence of the weather on the drone. Therefore it might be useful for future research to try to more accurately model oceanic current and the influence of wind and rain on the movement and detection accuracy of the drone.
Appendix
Full model results
Source.Name | Count | Mean | STD | Median | Min | Max | Succes Ratio | Succes Speed |
Sweep5_d5_w10.txt | 9006 | 306.4168332 | 66.22582738 | 280 | 240 | 590 | 0.9006 | 66.41683 |
Sweep4_d5_w10.txt | 8840 | 313.3597285 | 69.5844632 | 290 | 240 | 590 | 0.884 | 73.35973 |
Sweep3_d5_w10.txt | 8448 | 321.1316288 | 71.79807856 | 310 | 240 | 590 | 0.8448 | 81.13163 |
cone5_halfpi.txt | 8266 | 261.1154125 | 10.50518206 | 260 | 240 | 310 | 0.8266 | 21.11541 |
Cone_2_halfpi.txt | 7900 | 282.4898734 | 40.08713795 | 270 | 240 | 590 | 0.79 | 42.48987 |
cone_3_halfpi.txt | 7684 | 267.8227486 | 21.28586226 | 260 | 240 | 560 | 0.7684 | 27.82275 |
cone4_halfpi.txt | 7494 | 261.1956232 | 11.1747414 | 260 | 240 | 350 | 0.7494 | 21.19562 |
Sweep2_d5_w10.txt | 7417 | 328.5371444 | 71.95174355 | 310 | 240 | 590 | 0.7417 | 88.53714 |
Cone_2_3quarterpi.txt | 7303 | 316.4042174 | 73.25601744 | 280 | 240 | 570 | 0.7303 | 76.40422 |
Sweep1_d7_w5.txt | 7007 | 428.0034251 | 85.79344366 | 420 | 240 | 590 | 0.7007 | 188.0034 |
Sweep1_d5_w5.txt | 6940 | 335.2247839 | 73.24696142 | 320 | 240 | 590 | 0.694 | 95.22478 |
Cone3_3quarterpi.txt | 6801 | 284.2067343 | 42.58235158 | 270 | 240 | 590 | 0.6801 | 44.20673 |
cone4_3quarterpi.txt | 6644 | 268.385009 | 22.70430898 | 260 | 240 | 580 | 0.6644 | 28.38501 |
cone5_3quarterpi.txt | 6350 | 262.5905512 | 15.23111931 | 260 | 240 | 430 | 0.635 | 22.59055 |
Cone_1_ halfpi.txt | 5777 | 342.7367146 | 83.92058695 | 320 | 240 | 590 | 0.5777 | 102.7367 |
Cone_1_3quarterpi.txt | 5125 | 357.8887805 | 85.33027697 | 330 | 240 | 590 | 0.5125 | 117.8888 |
Sweep1_d7_w10.txt | 4534 | 430.8557565 | 87.55154123 | 440 | 240 | 590 | 0.4534 | 190.8558 |
Sweep1_d5_w10.txt | 3661 | 364.1873805 | 73.35725043 | 340 | 240 | 590 | 0.3661 | 124.1874 |
Sweep1_d3_w5.txt | 2635 | 288.2049336 | 46.36198948 | 280 | 240 | 540 | 0.2635 | 48.20493 |
cone5_quarterpi.txt | 2396 | 258.6978297 | 10.86522538 | 260 | 240 | 300 | 0.2396 | 18.69783 |
cone4_quarterpi.txt | 2242 | 258.8492417 | 11.08224585 | 260 | 240 | 300 | 0.2242 | 18.84924 |
Adapted_SQ.txt | 2236 | 325.8184258 | 71.30174178 | 350 | 240 | 590 | 0.2236 | 85.81843 |
Cone3_quarterpi.txt | 2060 | 257.7669903 | 10.84412396 | 260 | 240 | 290 | 0.206 | 17.76699 |
Cone_1_quarterpi.txt | 1689 | 279.2243931 | 37.61462698 | 270 | 240 | 580 | 0.1689 | 39.22439 |
Cone_2_quarterpi.txt | 1514 | 257.3051519 | 11.50892696 | 260 | 240 | 330 | 0.1514 | 17.30515 |
Sweep1_d3_w10.txt | 1436 | 288.6699164 | 70.27055499 | 240 | 240 | 590 | 0.1436 | 48.66992 |
Sweep1_d10_w5.txt | 968 | 528.7086777 | 40.5658764 | 530 | 340 | 590 | 0.0968 | 288.7087 |
Sweep1_d10_w10.txt | 530 | 533.1320755 | 50.86772116 | 560 | 430 | 590 | 0.053 | 293.1321 |
Sweep1_d1_w5.txt | 194 | 271.4948454 | 45.12885953 | 240 | 240 | 440 | 0.0194 | 31.49485 |
Square.txt | 141 | 313.9007092 | 52.69552773 | 300 | 240 | 460 | 0.0141 | 73.90071 |
Logbook
Week | Name | Work done & hours spent | Total hours |
---|---|---|---|
1 | Geert Touw | Group setup etc (2h), Subject brainstorm (2h) | 4h |
Luc van Burik | Group setup etc (2h), Subject brainstorm (2 h) | 4h | |
Victor le Fevre | Group setup etc (2h), Subject brainstorm (2h) | 4h | |
Thijs Egbers | Group setup etc (2h), Subject brainstorm (2h) | ||
Adrian Kondanari | Group setup etc (2h), Subject brainstorm (3h) | 5h | |
Aron van Cauter | Group setup etc (2h) | ||
2 | Geert Touw | Meeting 1 (1h), Meeting 2(1h), Subject brainstorm (1h) | 3h |
Luc van Burik | Meeting 1 (1h), Meeting 2(1h), Subject brainstorm (2h) | 4h | |
Victor le Fevre | Meeting 1 (1h), Meeting 2(1h), Subject brainstorm (1h) | 3h | |
Thijs Egbers | Meeting 1 (1h), Meeting 2(1h), Subject brainstorm (2h) | 4h | |
Adrian Kondanari | Meeting 1 (1h), First concept elaboration (2h) | 3h | |
Aron van Cauter | Meeting 1 (1h) | ||
3 | Geert Touw | Meeting 1 (1h), meeting 2 (1h), Oceanic weather + hypothermia and water + shipping routes research (3h) | 5h |
Luc van Burik | Meeting 2 (1h), IAMSAR reading (3.5h), Drone navigation research (1.5h) | 6h | |
Victor le Fevre | Meeting 1 (1h), Meeting 2 (1h), Made the wiki look a bit more coherent (1h)
Wrote the problem statement and objective, users, scenario and added points to the MoSCoW (1.5h) Read Sensor and Image Recognition papers (1h) |
5.5h | |
Thijs Egbers | Meeting 1 (1h), meeting 2 (1h), sea communication research (2h) | 4h | |
Adrian Kondanari | Meeting 1 (1h), requirements (2h), MOB protocols (2h) | 5h | |
Aron van Cauter | |||
4 | Geert Touw | Meeting 1 (1h), Meeting 2(1h), Ethics (4h) | 6h |
Luc van Burik | Meeting 1 (1h), Meeting 2(1h), Search area (1.5hr), Drone search speed (1.5 hr), Wiki writing (0.5 hr) | 5.5h | |
Victor le Fevre | Meeting 1 (1h), Meeting 2(1h), looked at sensors and image recognition (2h) | 4h | |
Thijs Egbers | Meeting 1 (1h), Meeting 2(1h), drone controll research (3h) | ||
Adrian Kondanari | Meeting 1 (1h), Meeting 2(1h), sensor research (2h) | 5h | |
Aron van Cauter | |||
5 | Geert Touw | Meeting 1(1h), Made a search pattern with Victor (2h) | 3h |
Luc van Burik | Meeting 1(1h), Working on Image detection(2.5h), meeting 2 (1h), Square search (2.5h),
adapted square search(WIP) (1h) |
8 h | |
Victor le Fevre | Meeting 1(1h), designed and tried tried to make an xy-rig (3h), worked on the wiki (2h) | 6h | |
Thijs Egbers | Meeting 1(1h), meeting 2 (1h), worked on getting the raspi setup (3h),
try to get the camera and soldering (0.5h) |
5.5h | |
Adrian Kondanari | Meeting 1(0.5h), communication methods research (4h) | 4.5h | |
Aron van Cauter | Meeting 1(1h) | ||
6 | Geert Touw | Meeting 1 (1h), Meeting 2 (1h), Worked on wiki (2h) | 4h |
Luc van Burik | meeting 2 (1h), Concert search pattern to work inside rest of the program (3h), programming more patterns(2h) | 6h | |
Victor le Fevre | Meeting 1 (1h), Meeting 2 (1h), Worked on wiki (4h) | 6h | |
Thijs Egbers | Meeting 1 (1h), Meeting 2 (1h), get the camera setup (1.5h), try to optimise camera reading (1h),
convert search pattern to work inside the rest of the program (3h) |
7.5h | |
Adrian Kondanari | Heating element (5h) | 5h | |
Aron van Cauter | |||
7 | Geert Touw | Meeting 2 (demo) (4h), Wiki (1h) | 5h |
Luc van Burik | Meeting 2 (demo) (4h), finishing touches test program (2h), presentation (3 h) | 9h | |
Victor le Fevre | Meeting 2 (demo) (4h), Wiki (1h) | 5h | |
Thijs Egbers | Meeting 1 (1h), meeting 2/video making (4h), finishing touches test program (2h),
make presentation (WIP) |
||
Adrian Kondanari | wiki (2h) | 2h | |
Aron van Cauter | |||
8 | Geert Touw | Presentation (0.5h), Short meeting to prepare for presentations (0.5h), wiki (1.5) | 2.5h |
Luc van Burik | presentations (2.5 h), Short meeting before presentations (.5h), working on wiki (6h) | 9h | |
Victor le Fevre | Finalizing wiki (5h) | 5h | |
Thijs Egbers | Presentations (2.5h), short meeting with Luc (0.25h), working on the wiki (4h) | ||
Adrian Kondanari | wiki (4h) | 4h | |
Aron van Cauter |
References
- ↑ ANNUAL OVERVIEW OF MARINE CASUALTIES AND INCIDENTS 2022. (2022). In EMSA (Ares(2022)8241169). European Maritime Safety Agency. from https://www.emsa.europa.eu/newsroom/latest-news/download/7362/4867/23.html
- ↑ Gonel, O., & Çiçek, I. (2020, 25 december). STATISTICAL ANALYSIS OF MAN OVER BOARD (MOB) INCIDENTS. ResearchGate. https://www.researchgate.net/publication/348266442_STATISTICAL_ANALYSIS_OF_MAN_OVER_BOARD_MOB_INCIDENTS
- ↑ https://www.marineinsight.com/marine-safety/man-overboard-situation-on-ship-and-ways-to-tackle-it/#:~:text=1
- ↑ https://content.iospress.com/download/international-shipbuilding-progress/isp23-260-02?id=international-shipbuilding-progress%2Fisp23-260-02
- ↑ https://owaysonline.com/iamsar-search-patterns/
- ↑ 6.0 6.1 O. (2021, 5 april). IAMSAR Search Patterns Explained with Sketches - Oways Online. Oways Online. https://owaysonline.com/iamsar-search-patterns/
- ↑ O. (2021, 5 april). IAMSAR Search Patterns Explained with Sketches - Oways Online. Oways Online. https://owaysonline.com/iamsar-search-patterns/
- ↑ 8.0 8.1 "Shipping routes are changing with the climate" by Brad Plumer, published on Vox on April 25, 2016 (https://www.vox.com/2016/4/25/11503152/shipping-routes-map).
- ↑ 9.0 9.1 "Cold Water Immersion and Hypothermia" by Aceboater, published on their website on an unknown date (https://aceboater.com/en-us/cold-water-immersion-and-hypothermia).
- ↑ 10.0 10.1 "SeaTemperature.org - Sea Surface Temperature (SST) map for the world's oceans and seas" by SeaTemperature.org, accessed on April 9, 2023 (https://www.seatemperature.org/).
- ↑ Frotan, D. F., & Moths, J. M. (2022). Human Body Presence Detection in Water Environments Using Pulse Coherent Radar [Bachelor Thesis]. Malmo University.
- ↑ Lomonaco, V., Trotta, A., Ziosi, M., De Dios Yáñez Ávila, J., & Díaz-Rodríguez, N. (2018b). Intelligent Drone Swarm for Search and Rescue Operations at Sea. HAL (Le Centre pour la Communication Scientifique Directe).
- ↑ Queralta, J. P., Raitoharju, J., Gia, T. N., Passalis, N., & Westerlund, T. (2020). AutoSOS: Towards Multi-UAV Systems Supporting Maritime Search and Rescue with Lightweight AI and Edge Computing. arXiv (Cornell University). https://arxiv.org/pdf/2005.03409.pdf
- ↑ Yeong, S. P., King, L. M., & Dol, S. S. (2015). A Review on Marine Search and Rescue Operations Using Unmanned Aerial Vehicles. Zenodo (CERN European Organization for Nuclear Research). https://doi.org/10.5281/zenodo.1107672
- ↑ Burke, C., McWhirter, P. R., Veitch-Michaelis, J., McAree, O., Pointon, H. A. G., Wich, S. A., & Longmore, S. N. (2019). Requirements and Limitations of Thermal Drones for Effective Search and Rescue in Marine and Coastal Areas. Drones, 3(4), 78. https://doi.org/10.3390/drones3040078
- ↑ Long-distance Video Streaming and Telemetry via Raw WiFi Radiohttps://dev.px4.io/v1.11_noredirect/en/qgc/video_streaming_wifi_broadcast.html
- ↑ AlphaPilot: autonomous drone racing, Philipp Foehn, Dario Brescianini, Elia Kaufmann, Titus Cieslewski, Mathias Gehrig, Manasi Muglikar & Davide Scaramuzza https://link.springer.com/article/10.1007/s10514-021-10011-y
- ↑ Autonomous Waypoint-based Guidance Methods for Small Size Unmanned Aerial Vehicles, Dániel Stojcsics http://acta.uni-obuda.hu/Stojcsics_56.pdf
- ↑ https://www.raspberrypi.com/documentation/accessories/camera.htm
- ↑ https://www.researchgate.net/publication/350981551_Real-Time_Human_Detection_in_Thermal_Infrared_Images
- ↑ Battistuzzi, L., Recchiuto, C.T. & Sgorbissa, A. Ethical concerns in rescue robotics: a scoping review. Ethics Inf Technol 23, 863–875 (2021). https://doi.org/10.1007/s10676-021-09603-0